A Priori Probability Definition Formula Example
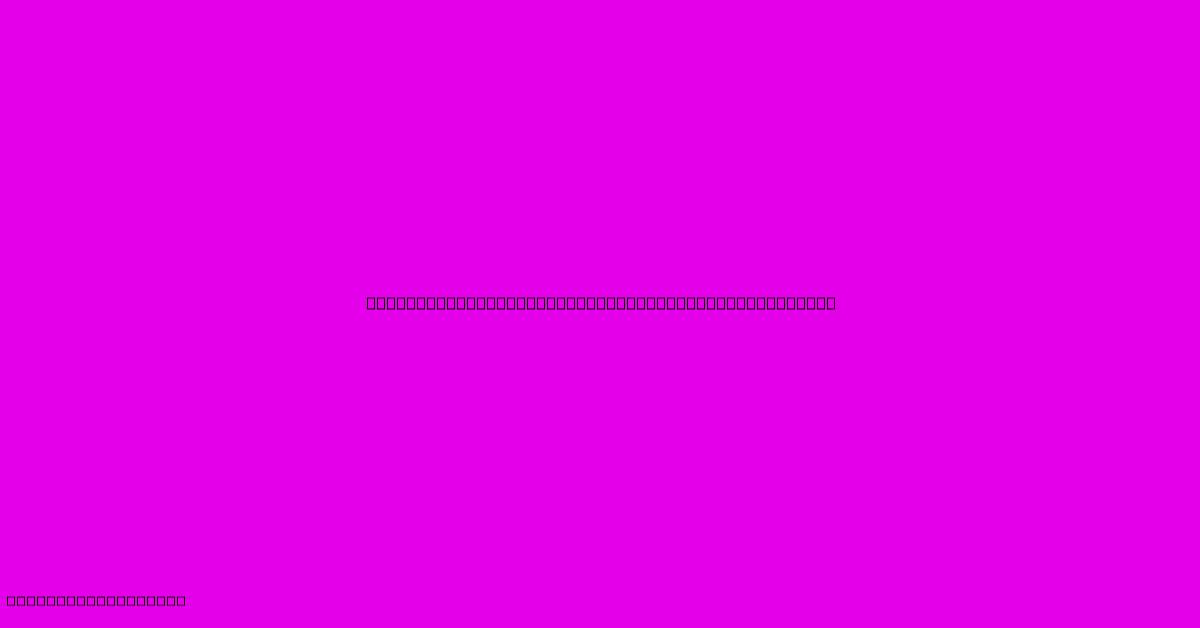
Discover more in-depth information on our site. Click the link below to dive deeper: Visit the Best Website meltwatermedia.ca. Make sure you don’t miss it!
Table of Contents
Unveiling A Priori Probability: Definition, Formula, and Examples
Hook: How can we assign probabilities to events before any observation? The concept of a priori probability offers a powerful framework for doing just that, providing crucial insights in numerous fields from statistics to machine learning.
Editor's Note: This comprehensive guide to a priori probability has been published today.
Relevance & Summary: Understanding a priori probability is vital for anyone working with probability and statistics. This guide provides a clear definition, explains the formula, and offers various examples demonstrating its practical applications. We will explore the classical interpretation, its limitations, and its contrast with a posteriori probability, using relevant semantic keywords and LSI terms like Bayesian inference, subjective probability, and prior distribution for enhanced SEO.
Analysis: This guide synthesizes information from established statistical texts and research papers to provide a thorough and accurate explanation of a priori probability. The examples provided are drawn from diverse fields to illustrate its broad applicability.
Key Takeaways:
- Definition of a priori probability.
- Formula for calculating a priori probability.
- Examples illustrating a priori probability calculations.
- Limitations of the classical approach to a priori probability.
- Contrast between a priori and a posteriori probability.
A Priori Probability: A Deep Dive
Subheading: A Priori Probability
Introduction: A priori probability, also known as classical probability, refers to the probability of an event based on prior knowledge or reasoning, before any empirical observation. It assumes that all possible outcomes are equally likely. This approach relies on deductive reasoning and logical analysis rather than experimental data.
Key Aspects: The core aspects of a priori probability include:
- Equal Likelihood: The fundamental assumption is that all possible outcomes are equally probable.
- Deductive Reasoning: Probabilities are derived through logical deduction based on the nature of the event and the sample space.
- Prior Knowledge: It utilizes prior knowledge about the event and its possible outcomes to assign probabilities.
Discussion: Consider a fair six-sided die. The a priori probability of rolling a 3 is 1/6, because there are six equally likely outcomes (1, 2, 3, 4, 5, 6), and only one of them is a 3. This is a clear example of deductive reasoning based on the inherent properties of the die. The same applies to drawing a specific card from a well-shuffled deck of cards. However, this approach has limitations. It struggles with events where outcomes are not equally likely, or when prior knowledge is incomplete or uncertain. This limitation often leads to the use of Bayesian methods, which incorporate both a priori and a posteriori probabilities.
Subheading: The Formula for A Priori Probability
Introduction: The formula for calculating a priori probability is straightforward.
Facets:
-
Formula: P(A) = N(A) / N(S)
- P(A) represents the probability of event A.
- N(A) represents the number of favorable outcomes for event A.
- N(S) represents the total number of possible outcomes in the sample space S.
-
Example 1 (Coin Toss): The probability of getting heads in a fair coin toss is P(Heads) = 1/2, because there is one favorable outcome (Heads) out of two possible outcomes (Heads, Tails).
-
Example 2 (Die Roll): The probability of rolling an even number on a fair six-sided die is P(Even) = 3/6 = 1/2, because there are three favorable outcomes (2, 4, 6) out of six possible outcomes (1, 2, 3, 4, 5, 6).
-
Risks and Mitigations: The main risk is misapplying the formula when outcomes are not equally likely. Mitigation involves careful consideration of whether the equally likely assumption holds.
-
Impacts and Implications: Accurate calculation of a priori probability is essential for making informed decisions in situations with known probabilities, especially in theoretical scenarios.
Summary: The formula provides a simple yet powerful way to calculate probabilities when the equally likely assumption holds true. Its limitations become apparent when dealing with more complex scenarios or events with unequal probabilities.
Subheading: Limitations of A Priori Probability
Introduction: The classical approach to a priori probability, while useful in simple cases, possesses significant limitations.
Further Analysis: Its reliance on the equally likely assumption often breaks down in real-world situations. For example, predicting the weather or the success of a medical treatment cannot rely on equally likely outcomes. Furthermore, the classical approach struggles to handle scenarios with incomplete information or subjective judgments. Imagine assessing the probability of a specific company succeeding – this requires considering various factors not easily reducible to equally likely outcomes.
Closing: The limitations highlight the need for more nuanced approaches, such as Bayesian inference, which combines a priori probabilities with observational data to generate more accurate a posteriori probabilities.
Subheading: A Priori vs. A Posteriori Probability
Introduction: Understanding the difference between a priori and a posteriori probability is crucial.
Further Analysis: A priori probability relies on prior knowledge or reasoning alone, while a posteriori probability (also known as empirical probability) is calculated based on observed data. For example, an a priori probability might estimate the chance of rain based on historical weather patterns for a given date. An a posteriori probability would adjust this estimate based on current weather conditions and forecasts. Bayesian inference effectively bridges these two approaches, updating prior beliefs (a priori) with new evidence (a posteriori) to produce refined posterior probabilities.
Closing: The distinction clarifies how these two probability approaches complement each other, offering different perspectives for understanding and quantifying uncertainty.
Subheading: FAQ
Introduction: This section addresses frequently asked questions regarding a priori probability.
Questions:
-
Q: What is the difference between a priori and subjective probability? A: A priori probability relies on logical reasoning and equally likely outcomes (in the classical sense), while subjective probability reflects individual beliefs or judgments based on limited or incomplete information.
-
Q: Can a priori probability be used in situations with unequal probabilities? A: Not directly in its classical form. Adjustments are needed using methods like Bayesian inference to account for unequal probabilities.
-
Q: What are some real-world applications of a priori probability? A: Game theory, risk assessment, and certain areas of statistical mechanics frequently utilize a priori probabilities.
-
Q: How does a priori probability relate to Bayesian inference? A: In Bayesian inference, a priori probability serves as the prior distribution, which is then updated with observed data to yield a posterior distribution.
-
Q: What are the limitations of using only a priori probability in decision-making? A: Ignoring observational data can lead to inaccurate or incomplete assessments of risk and uncertainty.
-
Q: Is a priori probability always accurate? A: No, its accuracy depends heavily on the validity of the equally likely assumption and the completeness of the prior knowledge.
Summary: This FAQ clarifies key aspects and misconceptions concerning a priori probability.
Subheading: Tips for Understanding A Priori Probability
Introduction: This section offers tips to enhance understanding of a priori probability.
Tips:
- Clearly define the sample space and all possible outcomes.
- Verify whether the equally likely assumption holds.
- Carefully count the number of favorable outcomes.
- Understand the formula and its applications.
- Consider the limitations of the a priori approach.
- Explore Bayesian methods for scenarios with unequal probabilities.
- Use real-world examples to solidify understanding.
- Practice calculating probabilities in various scenarios.
Summary: Mastering these tips significantly improves comprehension and application of a priori probability.
Subheading: Summary of A Priori Probability
Summary: This guide provided a detailed exploration of a priori probability, explaining its definition, formula, examples, limitations, and contrast with a posteriori probability. It highlighted the importance of recognizing the equally likely assumption and its limitations in complex real-world scenarios.
Closing Message: Understanding a priori probability is a fundamental step toward mastering probability theory and its wide-ranging applications. By embracing both its strengths and limitations, and exploring its connection to Bayesian methods, one can harness its power for accurate modeling and informed decision-making across diverse disciplines.
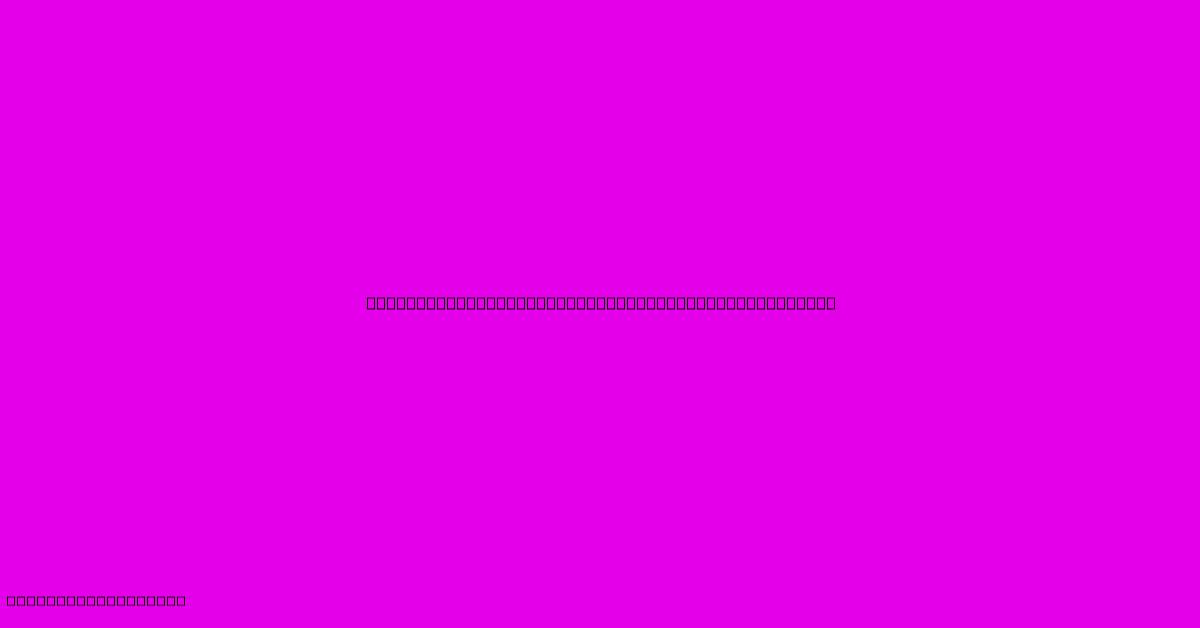
Thank you for taking the time to explore our website A Priori Probability Definition Formula Example. We hope you find the information useful. Feel free to contact us for any questions, and don’t forget to bookmark us for future visits!
We truly appreciate your visit to explore more about A Priori Probability Definition Formula Example. Let us know if you need further assistance. Be sure to bookmark this site and visit us again soon!
Featured Posts
-
When Does The Ex Spouse Collect Pension Funds
Jan 10, 2025
-
Net Unrealized Appreciation Nua Definition And Tax Treatment
Jan 10, 2025
-
How Do Student Loans Interest Work
Jan 10, 2025
-
What Process Could The Pension Funds Use To Gain Control Of Mylans Board
Jan 10, 2025
-
How To Buy Alcahol Stocks
Jan 10, 2025