Black Litterman Model Definition Basics Example
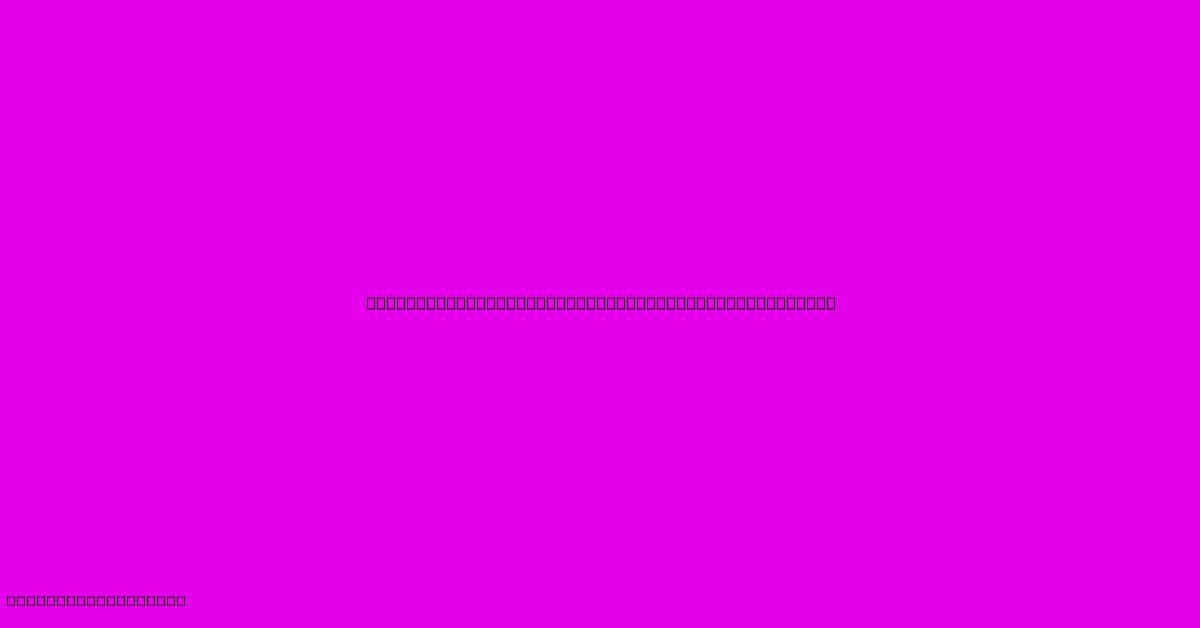
Discover more in-depth information on our site. Click the link below to dive deeper: Visit the Best Website meltwatermedia.ca. Make sure you don’t miss it!
Table of Contents
Unveiling the Black-Litterman Model: A Deep Dive into Asset Allocation
Does your portfolio allocation strategy account for your own unique views on market performance? A robust portfolio construction method should. The Black-Litterman model offers a powerful framework for integrating subjective views into traditional mean-variance optimization, leading to more informed and potentially superior investment decisions.
Editor's Note: This comprehensive guide to the Black-Litterman model has been published today.
Relevance & Summary: The Black-Litterman (BL) model revolutionized asset allocation by allowing investors to combine the objective market expectations (often derived from a market equilibrium model like the Capital Asset Pricing Model – CAPM) with their own subjective views. This results in a more personalized and potentially less volatile portfolio compared to solely relying on market equilibrium models. This guide will explore the model's mathematical foundations, practical applications, and key considerations for implementation, covering topics such as equilibrium returns, subjective views, and risk aversion.
Analysis: This guide utilizes a combination of academic research on portfolio optimization, mean-variance analysis, and Bayesian statistics to elucidate the Black-Litterman model. The explanations are grounded in the original Black-Litterman paper and subsequent refinements to make the concepts accessible to a broader audience. Numerous examples illustrate the practical application of the model.
Key Takeaways:
- The Black-Litterman model allows for the incorporation of investor views into portfolio optimization.
- It provides a structured approach to combine market equilibrium returns with subjective beliefs.
- The model produces more personalized and potentially less volatile portfolios.
- Understanding the model’s parameters, particularly the confidence level in views, is crucial.
- The model offers flexibility and can be adapted to various asset classes.
Black-Litterman Model: A Comprehensive Overview
The Black-Litterman model is a portfolio optimization technique that enhances the traditional mean-variance optimization approach. Unlike purely data-driven methods, BL incorporates investor-specific views, leading to more tailored portfolio allocations. The model's core strength lies in its ability to seamlessly integrate objective market equilibrium returns (often from the CAPM) with subjective, qualitative insights an investor might have about specific assets or market sectors. This hybrid approach helps to mitigate the inherent limitations of solely relying on market-implied expectations.
Key Aspects of the Black-Litterman Model
The BL model's functionality revolves around several critical aspects:
-
Equilibrium Returns: The model starts by establishing a set of equilibrium returns, typically derived from a market equilibrium model such as the CAPM. These returns reflect the market's overall expectation of asset performance.
-
Subjective Views: This is the heart of the BL model. Investors input their own views on specific asset returns, expressed as relative or absolute expected returns. For example, an investor might believe that a particular sector will outperform the market by a certain percentage.
-
Confidence Levels: The model requires investors to quantify their confidence in their views. A higher confidence level assigns more weight to the subjective view, while a lower confidence level gives more weight to the equilibrium returns. This is a crucial parameter influencing the final portfolio allocation.
-
Risk Aversion: The investor's risk aversion is incorporated through the risk aversion parameter (λ), which affects the portfolio's overall risk tolerance. A higher λ indicates a greater aversion to risk.
-
Portfolio Optimization: The model combines equilibrium returns, subjective views, and risk aversion to produce an optimized portfolio that balances expected return and risk. This often uses quadratic programming techniques to find the optimal asset weights.
Equilibrium Returns: The Foundation of the Black-Litterman Model
Equilibrium returns are the baseline expectations of asset performance, representing the market's overall view. The CAPM is frequently used to generate these returns, providing a framework for calculating expected returns based on market risk and asset beta. The CAPM's equation is:
E(Ri) = Rf + βi * [E(Rm) - Rf]
Where:
- E(Ri) is the expected return of asset i
- Rf is the risk-free rate of return
- βi is the beta of asset i (a measure of its systematic risk)
- E(Rm) is the expected return of the market portfolio
The CAPM, however, has its limitations; it assumes a perfectly efficient market, where all information is instantly incorporated into prices. The BL model acknowledges this limitation and allows for deviations from these equilibrium returns by integrating investor views.
Subjective Views: Incorporating Investor Expertise
The power of the Black-Litterman model lies in its ability to integrate the investor's own views. These views can be expressed in various ways, including:
-
Absolute Views: The investor expresses an expected return for a specific asset. For example, the investor believes that asset A will have a return of 12%.
-
Relative Views: The investor expresses a view on the relative performance of two assets. For instance, the investor believes that asset B will outperform asset C by 5%.
These views are represented mathematically using a vector (P) of views and a covariance matrix (Ω) reflecting the uncertainty associated with those views. Ω captures the investor's confidence in the views – a smaller Ω indicates higher confidence.
Confidence Levels and Risk Aversion: Tuning the Model
The confidence level assigned to the subjective views directly impacts the weight given to these views relative to the equilibrium returns. A high confidence level assigns a greater weight to the subjective views, effectively making the portfolio allocation more influenced by the investor’s personal insights. Conversely, low confidence levels prioritize the market equilibrium returns.
The risk aversion parameter (λ) determines the portfolio's risk tolerance. A higher λ signifies a greater aversion to risk, resulting in a more conservative portfolio with lower volatility. The choice of λ reflects the investor’s risk preferences and investment objectives.
Example: Black-Litterman Model in Action
Let's consider a simplified example with two assets, A and B. Suppose the equilibrium returns (from CAPM) are:
- E(Ra) = 8%
- E(Rb) = 10%
Assume the investor has a view that asset A will outperform asset B by 3%: E(Ra) - E(Rb) = 3%. They assign a high level of confidence to this view. The Black-Litterman model would then adjust the equilibrium returns to incorporate this view, resulting in new, revised expected returns that reflect both the market's overall expectation and the investor's specific belief. The resulting portfolio allocation would likely shift toward asset A due to the investor's confidence in its superior performance.
FAQs on the Black-Litterman Model
FAQ:
Q1: What are the limitations of the Black-Litterman model?
A1: The model relies on accurate estimations of equilibrium returns and covariance matrices. Inaccurate inputs can lead to suboptimal portfolio allocations. Additionally, determining the appropriate confidence level for investor views can be subjective.
Q2: Is the Black-Litterman model suitable for all investors?
A2: While versatile, the model requires a certain level of financial literacy and understanding of portfolio optimization principles. It’s particularly beneficial for investors with strong views on specific assets or market sectors and who are comfortable quantifying their confidence levels.
Q3: How does the Black-Litterman model differ from traditional mean-variance optimization?
A3: Traditional mean-variance optimization relies solely on market-implied expectations. The BL model adds a layer of personalization by incorporating investor-specific views, allowing for more tailored portfolio construction.
Q4: How does one determine the optimal confidence level for views?
A4: There’s no universally accepted method. It often involves a combination of experience, backtesting, and judgment. Sensitivity analysis can be used to understand how different confidence levels affect the resulting portfolio.
Q5: Can the Black-Litterman model handle multiple views simultaneously?
A5: Yes, the model can efficiently incorporate multiple views, allowing for a more holistic representation of the investor's outlook.
Q6: What software is commonly used to implement the Black-Litterman model?
A6: Various statistical software packages, including R and Python, provide libraries and functions for implementing the model.
Tips for Implementing the Black-Litterman Model
-
Start with robust equilibrium returns: Using a reliable method, such as the CAPM, for calculating equilibrium returns is critical.
-
Carefully formulate and quantify your views: Ensure views are clearly defined and their confidence level is appropriately assessed.
-
Use sensitivity analysis: Explore how different confidence levels and risk aversion parameters impact the resulting portfolio allocation.
-
Consider incorporating various asset classes: The BL model can be extended to encompass a wider range of assets, like real estate or commodities.
-
Regularly rebalance your portfolio: Market conditions change, and your views might evolve over time, necessitating regular rebalancing to maintain the desired risk and return profile.
Summary of the Black-Litterman Model
The Black-Litterman model provides a sophisticated approach to portfolio optimization, seamlessly integrating market-implied expectations with investor-specific views. Its ability to incorporate subjective insights while maintaining a quantitative framework makes it a valuable tool for informed decision-making in asset allocation. By carefully considering equilibrium returns, subjective views, confidence levels, and risk aversion, investors can construct personalized portfolios that align with their individual risk tolerance and investment objectives.
Closing Message: The Black-Litterman model offers a path toward more personalized and potentially superior investment strategies. While implementing the model requires understanding its underlying principles and parameters, the benefits of integrating personal insights into portfolio construction are significant. Continuous refinement of the model, adapting to market dynamics, and careful consideration of investor preferences remain key to unlocking its full potential.
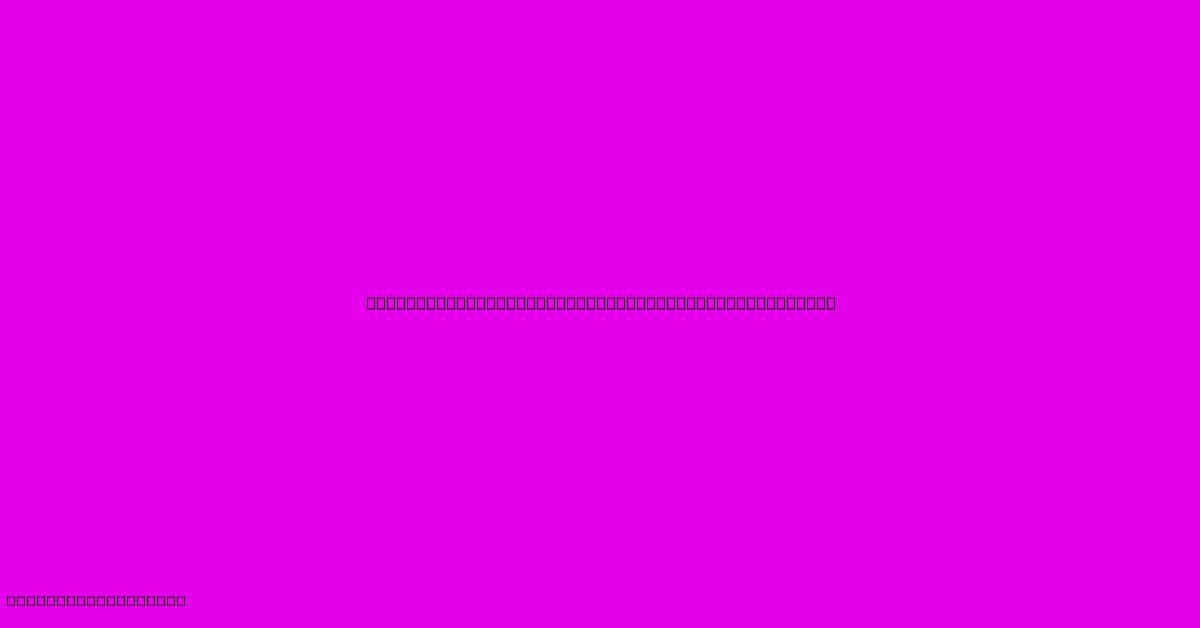
Thank you for taking the time to explore our website Black Litterman Model Definition Basics Example. We hope you find the information useful. Feel free to contact us for any questions, and don’t forget to bookmark us for future visits!
We truly appreciate your visit to explore more about Black Litterman Model Definition Basics Example. Let us know if you need further assistance. Be sure to bookmark this site and visit us again soon!
Featured Posts
-
Line Of Best Fit Definition How It Works And Calculation
Jan 08, 2025
-
How To Stop Automatic Investment Vanguard
Jan 08, 2025
-
Why Can Commodity Futures Contracts Be Bought And Sold On The Open Market
Jan 08, 2025
-
How To Make Money With Crowdfunding
Jan 08, 2025
-
Forex Broker Definition Role Regulation And Compensation
Jan 08, 2025