Box Jenkins Model Definition Uses Timeframes And Forecasting
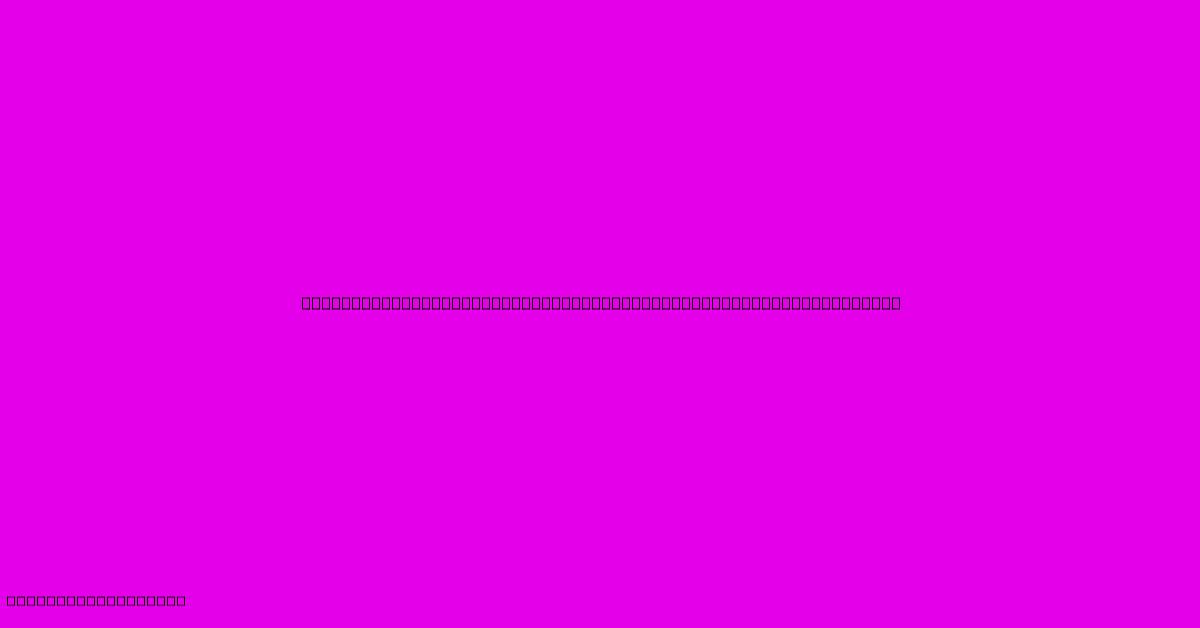
Discover more in-depth information on our site. Click the link below to dive deeper: Visit the Best Website meltwatermedia.ca. Make sure you don’t miss it!
Table of Contents
Unlocking Forecasting Accuracy: A Deep Dive into Box Jenkins Methodology
Does your forecasting strategy leave room for improvement? A robust forecasting model can significantly impact business decisions. The Box-Jenkins methodology offers a powerful, data-driven approach to time series analysis and prediction.
Editor's Note: This comprehensive guide to the Box-Jenkins model has been published today.
Relevance & Summary: Understanding and applying the Box-Jenkins methodology is crucial for businesses across various sectors. This method allows for accurate forecasting by identifying underlying patterns in time series data. This article provides a detailed explanation of the Box-Jenkins model, including its definition, uses, timeframes, and forecasting capabilities, equipping readers with the knowledge to improve their predictive analytics. Keywords include: Box-Jenkins model, ARIMA, time series analysis, forecasting, model identification, parameter estimation, diagnostic checking, timeframes, predictive modeling, statistical forecasting.
Analysis: This article synthesizes information from established statistical literature on time series analysis and forecasting, focusing on the practical application of the Box-Jenkins methodology. The analysis draws upon numerous examples to illustrate the model's capabilities and limitations across different timeframes.
Key Takeaways:
- The Box-Jenkins model is a powerful tool for time series forecasting.
- The methodology involves iterative steps of model identification, parameter estimation, and diagnostic checking.
- Different ARIMA models are suitable for various data characteristics and forecasting horizons.
- Accurate forecasting relies on careful data preparation and model selection.
- The model's effectiveness depends on the stationarity of the time series data.
The Box-Jenkins Methodology: A Comprehensive Overview
The Box-Jenkins approach is a powerful statistical method for building time series models. Its core lies in the family of Autoregressive Integrated Moving Average (ARIMA) models. Unlike simpler forecasting methods, Box-Jenkins is not a one-size-fits-all solution; rather, it's an iterative process designed to identify the most appropriate ARIMA model for a given dataset. This adaptability makes it particularly valuable for complex time series data exhibiting trends, seasonality, and cyclical patterns.
Key Aspects of the Box-Jenkins Model
The Box-Jenkins methodology comprises three crucial stages:
-
Model Identification: This stage involves analyzing the autocorrelation function (ACF) and partial autocorrelation function (PACF) of the time series data to identify the potential ARIMA model. The patterns in these functions reveal the order of the autoregressive (AR), integrated (I), and moving average (MA) components.
-
Parameter Estimation: Once a tentative ARIMA model is identified, its parameters are estimated using statistical methods such as maximum likelihood estimation. This involves finding the values that best fit the model to the observed data.
-
Diagnostic Checking: After parameter estimation, the model's adequacy is assessed through diagnostic checks. These checks evaluate the model's residuals (the differences between the predicted and actual values) to ensure they are random and uncorrelated. Significant autocorrelation in the residuals indicates model misspecification, requiring a return to the model identification stage.
Model Identification: Deciphering the Autocorrelation Functions
The autocorrelation function (ACF) measures the correlation between a time series and its lagged values. The partial autocorrelation function (PACF) measures the correlation between a time series and its lagged values, controlling for the intermediate lags. By examining the ACF and PACF plots, analysts can identify the order of the AR and MA components in the ARIMA model (p, d, q).
-
'p' (Autoregressive Order): Represents the number of lagged values of the dependent variable included in the model. A decaying ACF and a sharp cutoff at lag 'p' in the PACF suggests an AR model.
-
'd' (Integrated Order): Represents the number of times the time series needs to be differenced to achieve stationarity (constant mean and variance over time). Differencing involves subtracting consecutive data points.
-
'q' (Moving Average Order): Represents the number of lagged forecast errors included in the model. A decaying PACF and a sharp cutoff at lag 'q' in the ACF suggests an MA model.
Parameter Estimation: Fine-tuning the Model
Once the order (p, d, q) is determined, the model's parameters are estimated using statistical software packages. These parameters quantify the influence of past observations and forecast errors on current values. Maximum likelihood estimation is a common technique used for parameter estimation, aiming to find parameter values that maximize the likelihood of observing the actual data given the chosen model.
Diagnostic Checking: Validating the Model
Diagnostic checks are crucial for ensuring the model's adequacy. Common diagnostic tools include:
- Residual Analysis: Examination of the residuals to ensure they are randomly distributed with a mean of zero and constant variance. Autocorrelation in the residuals indicates model misspecification.
- Ljung-Box Test: A statistical test to assess the overall randomness of the residuals. A significant p-value indicates autocorrelation.
Timeframes and Forecasting Horizons
The Box-Jenkins methodology can be applied to various timeframes, from short-term forecasting (e.g., daily or weekly sales) to long-term forecasting (e.g., annual economic growth). The choice of timeframe and the corresponding ARIMA model depend on the data's characteristics and the forecasting horizon. Shorter timeframes may necessitate models with more complex structures to capture short-term fluctuations, while longer timeframes might benefit from simpler models that focus on long-term trends.
Real-World Applications Across Timeframes
-
Short-Term Forecasting (Daily/Weekly): Inventory management, supply chain optimization, energy demand prediction. Models may incorporate high-frequency data and capture short-term fluctuations.
-
Medium-Term Forecasting (Monthly/Quarterly): Sales forecasting, production planning, financial market analysis. Models can capture seasonal patterns and incorporate leading indicators.
-
Long-Term Forecasting (Annual): Economic forecasting, climate change modeling, demographic projections. Models typically focus on long-term trends and structural changes.
Point: ARIMA Model Selection
Introduction: The choice of the appropriate ARIMA(p,d,q) model is crucial for effective forecasting within the Box-Jenkins framework. This selection directly impacts the model's accuracy and reliability.
Facets:
- ACF and PACF Analysis: The primary method for identifying potential ARIMA models. The patterns in these plots guide the selection of p, d, and q values.
- Model Complexity: Simpler models (lower p, d, q) are preferred if they adequately capture the data's characteristics. Overly complex models can lead to overfitting.
- Stationarity: The time series must be stationary (constant mean and variance) before applying the ARIMA model. Differencing (d) transforms non-stationary data into stationary data.
- Information Criteria: AIC (Akaike Information Criterion) and BIC (Bayesian Information Criterion) are used to compare the goodness of fit of different models, penalizing for complexity.
- Diagnostic Checks: Essential for validating the selected model's adequacy. Residual analysis and hypothesis testing ensure the model accurately reflects the underlying data process.
Summary: Selecting the optimal ARIMA model involves a careful balance between model complexity and goodness of fit. A well-selected model accurately captures the data’s underlying pattern while avoiding overfitting. Thorough diagnostic checking validates the final model's performance.
Point: Limitations of the Box-Jenkins Model
Introduction: While powerful, the Box-Jenkins methodology has limitations that practitioners should be aware of. Understanding these limitations ensures the model is applied appropriately and its results are interpreted accurately.
Further Analysis:
- Data Requirements: The Box-Jenkins model requires a sufficient amount of historical data to ensure reliable parameter estimation. Limited data can hinder accurate model identification and forecasting.
- Stationarity Assumption: The model assumes that the time series is stationary or can be made stationary through differencing. Non-stationary time series may require alternative modeling approaches.
- Linearity Assumption: The model assumes a linear relationship between past observations and future values. Nonlinear relationships may require non-linear time series models.
- Outlier Sensitivity: Outliers in the data can significantly impact model parameter estimation and forecasting accuracy. Robust methods may be needed to handle outliers.
Closing: Despite these limitations, the Box-Jenkins methodology remains a valuable tool for time series forecasting when its assumptions are met and its limitations are acknowledged.
FAQ
Introduction: This section addresses common questions regarding the Box-Jenkins model.
Questions:
-
Q: What are the advantages of using the Box-Jenkins model? A: Its flexibility in handling various data patterns, data-driven approach, and ability to provide accurate forecasts are key advantages.
-
Q: What are the key assumptions of the Box-Jenkins model? A: Stationarity or transformability to stationarity, and linearity are key assumptions.
-
Q: How does the Box-Jenkins model handle seasonality? A: Seasonal ARIMA (SARIMA) models extend the standard ARIMA model to account for seasonal patterns in data.
-
Q: What software can be used for Box-Jenkins modeling? A: Statistical software packages like R, Python (statsmodels), and SPSS are commonly used.
-
Q: How can I assess the accuracy of my Box-Jenkins forecast? A: Use measures like Mean Absolute Error (MAE), Root Mean Squared Error (RMSE), and Mean Absolute Percentage Error (MAPE) to compare forecasts against actual values.
-
Q: What if my data has outliers? A: Outliers need careful consideration. Robust estimation techniques or outlier removal may be necessary.
Summary: This FAQ section provided answers to common questions, clarifying aspects of the Box-Jenkins methodology.
Tips of Box-Jenkins Modeling
Introduction: These tips help improve the application and interpretation of the Box-Jenkins methodology.
Tips:
- Thorough Data Exploration: Before modeling, examine the data visually and statistically to understand its characteristics (trends, seasonality, outliers).
- Data Preprocessing: Ensure the data is cleaned, with missing values handled appropriately.
- Stationarity Check: Always verify stationarity before model building.
- Careful Model Selection: Use ACF and PACF plots judiciously to select the appropriate ARIMA model.
- Diagnostic Checking: Always perform diagnostic checks to validate model adequacy.
- Model Validation: Use holdout data or cross-validation to assess the model's out-of-sample forecasting accuracy.
- Consider External Variables: If relevant, incorporate external variables (regressors) for more accurate predictions.
Summary: Following these tips improves the accuracy and reliability of forecasting using the Box-Jenkins methodology.
Summary of Box-Jenkins Model
The Box-Jenkins approach provides a robust framework for time series forecasting by systematically identifying, estimating, and validating ARIMA models. Its ability to handle various data patterns makes it a versatile tool in different applications. However, understanding its assumptions and limitations is crucial for effective implementation.
Closing Message: Mastering the Box-Jenkins methodology empowers businesses to make data-driven decisions based on accurate forecasting. By adopting this rigorous approach, organizations can optimize resource allocation, improve operational efficiency, and gain a competitive edge. Continuous monitoring and refinement of the model are vital to ensure its ongoing relevance and accuracy.
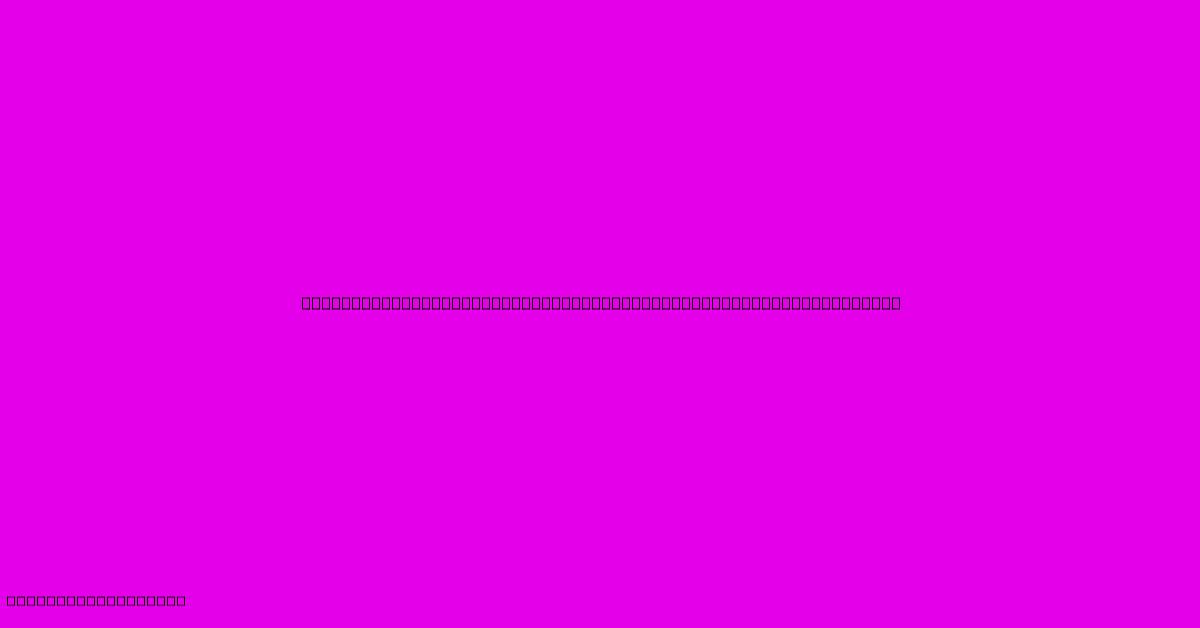
Thank you for taking the time to explore our website Box Jenkins Model Definition Uses Timeframes And Forecasting. We hope you find the information useful. Feel free to contact us for any questions, and don’t forget to bookmark us for future visits!
We truly appreciate your visit to explore more about Box Jenkins Model Definition Uses Timeframes And Forecasting. Let us know if you need further assistance. Be sure to bookmark this site and visit us again soon!
Featured Posts
-
How To Read Experian Credit Report
Jan 08, 2025
-
What Is Cash Flow Positive
Jan 08, 2025
-
What Is A Money Purchase Plan Definition And Benefits
Jan 08, 2025
-
Nasdaq Intermarket Definition
Jan 08, 2025
-
Nationalization Definition In The Oil Industry And The U S
Jan 08, 2025