Bunching Definition
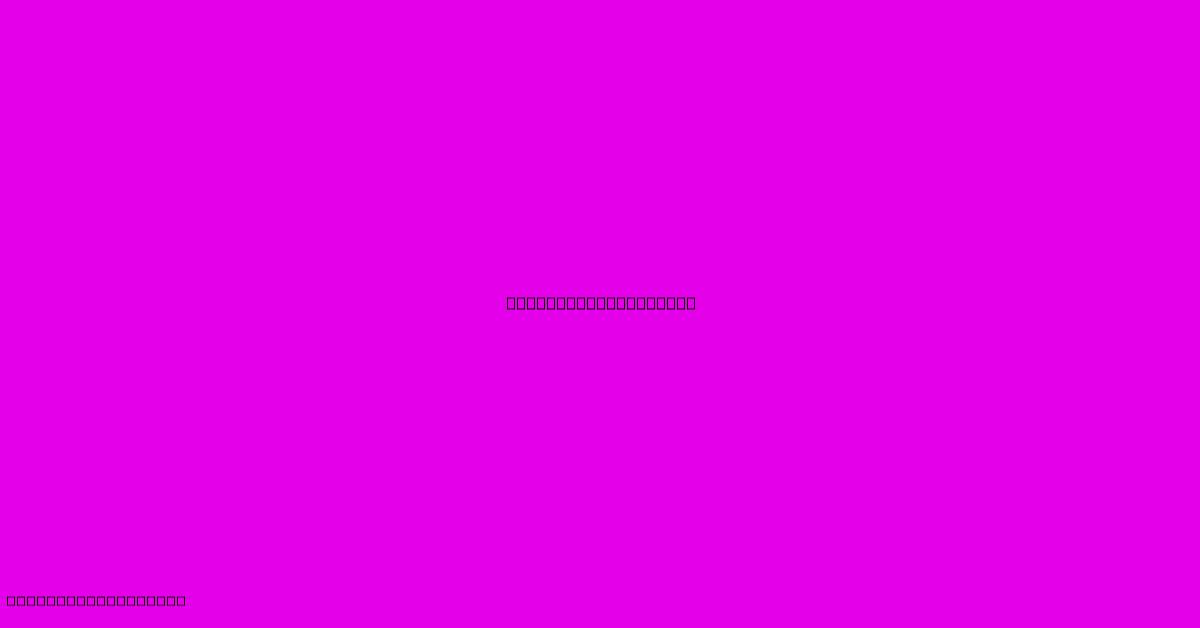
Discover more in-depth information on our site. Click the link below to dive deeper: Visit the Best Website meltwatermedia.ca. Make sure you don’t miss it!
Table of Contents
Unveiling the Nuances of Bunching: A Comprehensive Guide
Hook: What if seemingly insignificant variations in data could significantly skew your analyses? This is the crucial role of bunching—a phenomenon impacting fields from economics to statistics. Understanding its implications is vital for accurate interpretation and robust decision-making.
Editor's Note: This comprehensive guide to bunching has been published today.
Relevance & Summary: Bunching, the clustering of observations around a specific value or threshold, presents significant challenges and opportunities across various disciplines. This guide explores bunching's definition, its manifestation across different contexts, methods for detection, and strategies for mitigating its impact. Key terms explored include threshold effects, bunching analysis, regression discontinuity design, and data clustering.
Analysis: This guide synthesizes existing literature and research on bunching, focusing on its theoretical underpinnings and practical applications. It draws upon numerous case studies across economics, statistics, and related fields to illustrate the phenomenon's diverse manifestations and impact.
Key Takeaways:
- Bunching is a clustering of data points near a specific value.
- Understanding bunching is crucial for accurate data interpretation.
- Various statistical methods help detect and address bunching.
- Mitigating bunching effects is vital for reliable research.
- Bunching analysis helps researchers extract valuable information.
Bunching: Definition and Manifestation
Bunching refers to the non-random clustering of observations around a particular value or threshold. This phenomenon frequently arises when individuals or entities respond to incentives or regulations that create a clear breakpoint. The resulting data exhibit an unusual concentration of observations just below the threshold, with a significant drop-off immediately above it. This contrasts with a smooth, continuous distribution that would be expected in the absence of such a threshold effect. The intensity and extent of the bunching reveal valuable insights into the underlying behavioral responses and the effectiveness of the policy or incentive in question.
Key Aspects of Bunching Analysis
Understanding bunching requires examining several key aspects:
- Thresholds: Identifying the precise threshold driving the bunching behavior is paramount. This could be a tax bracket, a regulatory limit, or any other point at which incentives change abruptly.
- Behavioral Responses: Analyzing the bunching pattern reveals how agents respond to the threshold. The degree of clustering indicates the sensitivity to the incentive or regulation.
- Counterfactual Estimation: Determining what the distribution would look like without the threshold is vital for accurately measuring the bunching effect. This often involves sophisticated statistical techniques like regression discontinuity design.
- Data Quality: The accuracy of bunching analysis hinges on the quality and reliability of the data. Outliers or measurement errors can distort the results.
Threshold Effects and Bunching
A pivotal aspect of bunching is the existence of a threshold—a specific value that triggers a change in incentives or regulations. This discontinuity creates a point of attraction for observations, leading to bunching. Examples include:
- Taxation: Individuals may adjust their income slightly to avoid moving into a higher tax bracket. This often results in bunching at the lower bound of the higher bracket.
- Regulations: Companies might alter their behavior to comply with regulatory thresholds, leading to bunching near compliance limits.
- Healthcare: Individuals might choose healthcare plans based on deductible thresholds, potentially causing bunching around those points.
Regression Discontinuity Design and Bunching
Regression discontinuity design (RDD) is a powerful quasi-experimental technique frequently employed to analyze bunching. RDD exploits the discontinuity created by a threshold to estimate the causal effect of a treatment or intervention. By comparing outcomes for individuals just above and below the threshold, researchers can isolate the effect of the treatment while controlling for other factors. The extent of bunching informs the power and validity of the RDD analysis. A significant bunching effect enhances the precision of the causal effect estimation.
Detecting and Mitigating Bunching
Several statistical techniques help detect and address bunching:
- Visual Inspection: A simple histogram or density plot can reveal obvious bunching patterns.
- Non-parametric Density Estimation: Techniques like kernel density estimation provide a smoother representation of the data distribution, making bunching more apparent.
- Regression Analysis: Regression models can quantify the relationship between the variable of interest and the threshold, revealing the degree of bunching.
- Robust Statistical Methods: When dealing with potential outliers or measurement errors, robust statistical methods are crucial for reliable bunching analysis.
Mitigating the effects of bunching often involves careful data cleaning, appropriate statistical modeling, and consideration of the underlying behavioral mechanisms driving the clustering. In certain cases, adjusting the data or employing specialized statistical techniques may be necessary to obtain unbiased estimates.
Bunching in Economics: A Case Study
In economics, bunching is often observed in tax data, where individuals strategically adjust their income to remain in a lower tax bracket. Analyzing the degree of bunching provides insights into taxpayers' sensitivity to marginal tax rates and their willingness to engage in tax avoidance or optimization strategies. The magnitude of bunching can then be used to estimate the elasticity of taxable income with respect to tax rates. This is crucial information for designing efficient and equitable tax policies.
Bunching in Other Disciplines
While economics is a prominent area where bunching is studied, it's not limited to this field. For example:
- Environmental Science: Bunching can be observed in pollutant emission data, reflecting firms' responses to environmental regulations.
- Healthcare: Bunching may appear in studies of health insurance choices, reflecting individuals' responses to changes in cost-sharing.
- Education: Bunching may manifest in college application data, where students adjust their grades or test scores to meet admission thresholds.
FAQ
Introduction: This section addresses frequently asked questions regarding bunching.
Questions:
- Q: What is the difference between bunching and clustering? A: While both involve grouping data points, bunching specifically refers to clustering around a known threshold, driven by a specific incentive or regulation. Clustering is a more general term encompassing various grouping patterns.
- Q: Can bunching be a problem? A: Yes, if unaccounted for, bunching can lead to biased estimates and inaccurate conclusions in research.
- Q: How can I detect bunching in my data? A: Visual inspection (histograms, density plots), non-parametric density estimation, and regression analysis can all reveal bunching patterns.
- Q: What are the implications of ignoring bunching? A: Ignoring bunching can lead to misinterpretations of causal relationships and flawed policy recommendations.
- Q: What statistical methods address bunching? A: Regression discontinuity design (RDD) is particularly useful for analyzing bunching near thresholds.
- Q: How is bunching used in policy evaluation? A: Bunching analysis helps evaluate the effectiveness of policies by quantifying behavioral responses to thresholds.
Summary: Understanding and addressing bunching is critical for accurate data interpretation across various disciplines.
Transition: Let's now delve into practical tips for handling bunching in your own analyses.
Tips for Analyzing Bunching
Introduction: This section provides practical strategies for effectively analyzing and interpreting bunching in your data.
Tips:
- Visualize Your Data: Always start with visual exploration. Histograms and density plots quickly reveal bunching patterns.
- Identify the Threshold: Clearly define the threshold driving the suspected bunching.
- Consider Behavioral Responses: Think about the potential incentives or regulations leading to bunching.
- Employ Appropriate Statistical Methods: Choose methods (RDD, etc.) appropriate for your data and research question.
- Assess Data Quality: Address outliers and measurement errors to avoid skewed results.
- Interpret Cautiously: Bunching can be complex; interpret results within the context of your data and research design.
- Report Transparency: Clearly describe your methods and limitations to ensure reproducibility and allow for critical evaluation.
Summary: By following these tips, researchers can more effectively analyze and interpret bunching in their data, enhancing the accuracy and validity of their findings.
Summary of Bunching Analysis
This guide explored the multifaceted phenomenon of bunching—the clustering of observations around a threshold driven by incentives or regulations. We examined its definition, manifestations across disciplines, detection methods, and mitigation strategies. Understanding bunching is crucial for accurate data interpretation and effective policy evaluation.
Closing Message: The study of bunching provides valuable insights into behavioral responses to incentives and regulations. Future research should focus on developing more sophisticated methods to analyze bunching in complex settings and explore the interactions between bunching and other forms of behavioral biases. As data analysis becomes increasingly crucial in diverse fields, mastering the nuances of bunching is paramount for reliable and impactful research.
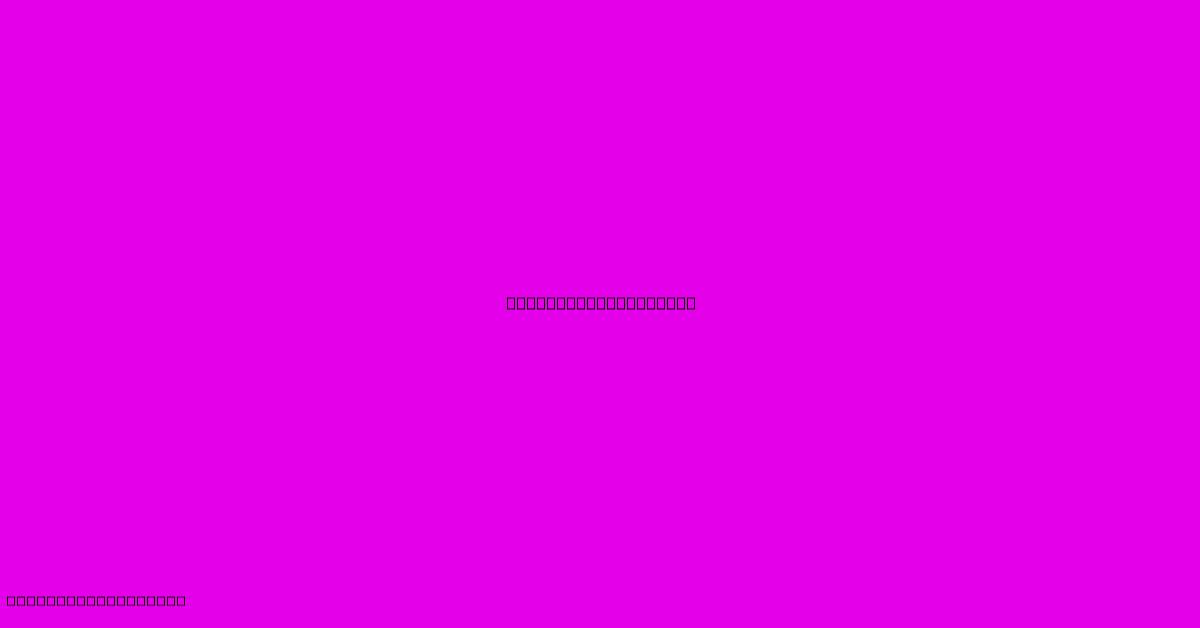
Thank you for taking the time to explore our website Bunching Definition. We hope you find the information useful. Feel free to contact us for any questions, and don’t forget to bookmark us for future visits!
We truly appreciate your visit to explore more about Bunching Definition. Let us know if you need further assistance. Be sure to bookmark this site and visit us again soon!
Featured Posts
-
What Is Title 1 Funding
Jan 08, 2025
-
What Is Blue Collar Definition And Job Examples
Jan 08, 2025
-
How Much Does Contributing To A 401k Reduce Taxes
Jan 08, 2025
-
Black Monday Definition In Stocks What Caused It And Losses
Jan 08, 2025
-
Boolean Algebra Definition
Jan 08, 2025