Central Limit Theorem Clt Definition And Key Characteristics
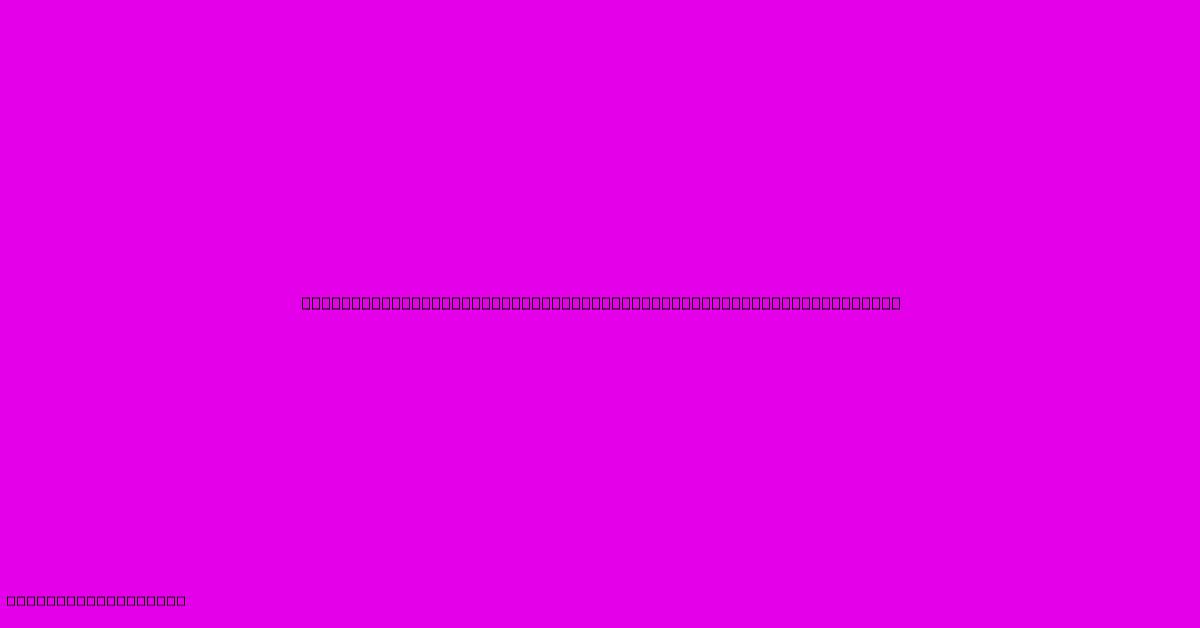
Discover more in-depth information on our site. Click the link below to dive deeper: Visit the Best Website meltwatermedia.ca. Make sure you don’t miss it!
Table of Contents
Unveiling the Central Limit Theorem: Definition, Characteristics, and Significance
Hook: Does the shape of a data distribution consistently influence the accuracy of population estimations? The Central Limit Theorem (CLT) provides a powerful answer, revealing profound implications for statistical inference and data analysis.
Editor's Note: This comprehensive guide to the Central Limit Theorem has been published today.
Relevance & Summary: Understanding the Central Limit Theorem is crucial for anyone working with data, from statisticians and researchers to data scientists and business analysts. This article will explore the theorem's definition, key characteristics, and practical applications, clarifying its importance in statistical hypothesis testing, confidence intervals, and sampling distributions. Key terms like sampling distribution, standard error, and normal distribution will be discussed in detail.
Analysis: This guide synthesizes established statistical principles and theorems related to probability distributions and sampling theory. The explanations are grounded in foundational statistical concepts to ensure clarity and comprehensiveness.
Key Takeaways:
- The CLT describes the behavior of sample means.
- It states that the distribution of sample means approaches a normal distribution as sample size increases.
- This holds true regardless of the shape of the original population distribution (provided it has a finite mean and variance).
- The CLT is fundamental to many statistical inference procedures.
Subheading: The Central Limit Theorem
Introduction: The Central Limit Theorem is a cornerstone of statistical inference. It asserts a remarkable property of sample means: irrespective of the underlying population distribution's shape, the distribution of the sample means will approximate a normal distribution under specific conditions. This makes the CLT invaluable for making inferences about population parameters based on sample data.
Key Aspects: The CLT is characterized by several key elements: the sample size (n), the population mean (µ), the population standard deviation (σ), the sample mean (x̄), and the standard error of the mean (SEM).
Discussion:
The CLT's power lies in its generality. It doesn't require the original population data to be normally distributed. This is crucial because many real-world datasets deviate from normality. Whether dealing with skewed data, bimodal data, or data with heavy tails, the CLT assures that the distribution of sample means will tend towards normality as the sample size increases. This convergence to normality allows for the application of powerful statistical tools based on the normal distribution, such as z-tests and t-tests, to analyze data. The larger the sample size, the closer the sampling distribution of the mean will be to a normal distribution. Even for highly skewed populations, with sufficient sample size (generally considered to be n ≥ 30), the CLT provides a robust approximation.
The standard error of the mean (SEM) plays a critical role in understanding the CLT. The SEM measures the variability of the sample means. It's calculated as σ/√n, where σ is the population standard deviation and n is the sample size. As the sample size increases, the SEM decreases, resulting in a narrower and more concentrated sampling distribution of the mean, thus reinforcing the convergence toward normality.
Subheading: Conditions for the Central Limit Theorem
Introduction: While the CLT is remarkably robust, certain conditions must be met for it to hold true. Understanding these conditions ensures appropriate application and interpretation of the theorem.
Facets:
- Independence: The samples must be independent. This means that the selection of one sample point should not influence the selection of any other sample point. Violation of independence can significantly affect the accuracy of the CLT's approximation.
- Random Sampling: The data must be obtained through random sampling. This ensures that each member of the population has an equal chance of being selected, preventing bias in the sample and improving the reliability of the CLT's approximation.
- Finite Variance: The population from which the samples are drawn must have a finite mean and variance. If the variance is infinite, the CLT doesn't apply. This condition is typically satisfied in practical applications.
- Sample Size: While the CLT works for smaller samples, the approximation is generally better with larger sample sizes. A commonly used rule of thumb is that a sample size of at least 30 is sufficient for a reasonable approximation, especially when the population distribution is not severely skewed.
Summary: These conditions underscore the importance of appropriate sampling techniques and data characteristics when applying the CLT. Failure to meet these conditions can lead to inaccurate conclusions based on the CLT's approximation.
Subheading: Applications of the Central Limit Theorem
Introduction: The CLT's wide-ranging applications span diverse fields, empowering researchers and analysts to make inferences about populations based on sample data.
Further Analysis: The CLT forms the basis for many statistical tests and estimations. These include:
- Confidence Intervals: Constructing confidence intervals for population means relies on the CLT's guarantee of a nearly normal distribution of sample means, even when the population distribution is unknown.
- Hypothesis Testing: Many hypothesis tests, like z-tests and t-tests, assume normality of the sampling distribution. The CLT justifies this assumption in many situations.
- Quality Control: In manufacturing and other industries, the CLT is used to monitor and control process variability. By taking samples and examining their means, manufacturers can infer characteristics of the entire production process.
- Polling and Surveys: Public opinion polls and surveys utilize the CLT to estimate population proportions with confidence intervals. The sampling distribution of the sample proportion approximates a normal distribution under certain conditions, allowing for reliable estimation.
Closing: The CLT's significance stems from its ability to simplify statistical analysis by providing a reliable approximation to normality, regardless of the population's true distribution. This simplification is vital for drawing accurate conclusions from sample data.
Subheading: FAQ
Introduction: This section addresses frequently asked questions about the Central Limit Theorem.
Questions:
-
Q: What happens if the sample size is small? A: With small sample sizes, the approximation to normality might be less accurate. The distribution of sample means may still be closer to normal than the underlying population, but the approximation improves significantly with larger sample sizes.
-
Q: Does the CLT apply to all types of data? A: No. The CLT requires independence and a finite population variance. Data with extreme outliers or heavy-tailed distributions may require additional considerations.
-
Q: Can the CLT be used with non-numerical data? A: The CLT is typically applied to numerical data, although adaptations exist for categorical data using proportions.
-
Q: Why is the standard error important? A: The standard error reflects the variability of the sample means. A smaller standard error indicates greater precision in estimating the population mean.
-
Q: What if the population distribution is unknown? A: The beauty of the CLT is that it works even if the population distribution is unknown. The key is a sufficiently large sample size.
-
Q: What are some common misconceptions about the CLT? A: A common misconception is that the CLT requires the underlying population to be normally distributed. This is incorrect; the CLT holds even for non-normal populations.
Summary: These FAQs emphasize the importance of understanding the limitations and assumptions of the CLT.
Subheading: Tips for Applying the Central Limit Theorem
Introduction: This section provides practical tips for effectively applying the Central Limit Theorem.
Tips:
- Ensure Random Sampling: Use appropriate random sampling techniques to obtain representative samples.
- Check for Independence: Verify the independence of observations before applying the CLT.
- Assess Sample Size: Aim for a sample size of at least 30 for a reliable approximation to normality.
- Consider Data Transformations: For severely skewed data, consider transformations to improve the approximation.
- Visualize the Data: Create histograms and other visual representations of your sample means to assess the degree of normality.
- Use Appropriate Statistical Tests: Choose statistical tests that align with the assumptions of the CLT.
- Understand Limitations: Be aware of the limitations of the CLT, and don't overinterpret results.
Summary: These tips highlight the importance of careful planning and execution when employing the Central Limit Theorem.
Summary: This article explored the definition, characteristics, and applications of the Central Limit Theorem. Its robustness and wide applicability make it essential for a variety of statistical analyses.
Closing Message: The Central Limit Theorem remains a powerful tool for statisticians and data analysts. Understanding its intricacies and applying its principles correctly allows for more accurate and reliable statistical inference, leading to better-informed decision-making across numerous disciplines. Further exploration of related statistical concepts will only enhance one's understanding and application of this fundamental theorem.
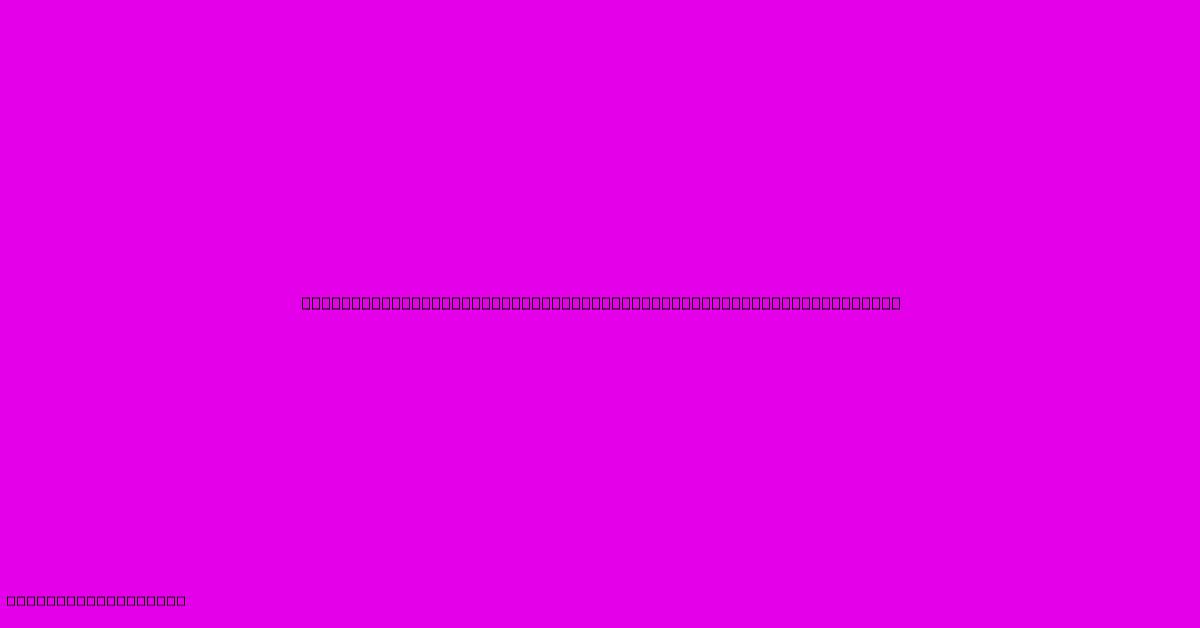
Thank you for taking the time to explore our website Central Limit Theorem Clt Definition And Key Characteristics. We hope you find the information useful. Feel free to contact us for any questions, and don’t forget to bookmark us for future visits!
We truly appreciate your visit to explore more about Central Limit Theorem Clt Definition And Key Characteristics. Let us know if you need further assistance. Be sure to bookmark this site and visit us again soon!
Featured Posts
-
What Can Ncl Onboard Credit Be Used For
Jan 03, 2025
-
How Often Does Stellantis Pay Dividends
Jan 03, 2025
-
Canadian Securities Course Csc Definition Subjects Exams
Jan 03, 2025
-
How To Remove Credit Card From Nintendo Switch
Jan 03, 2025
-
What Bank Is Burlington Credit Card
Jan 03, 2025