Forward Averaging Definition
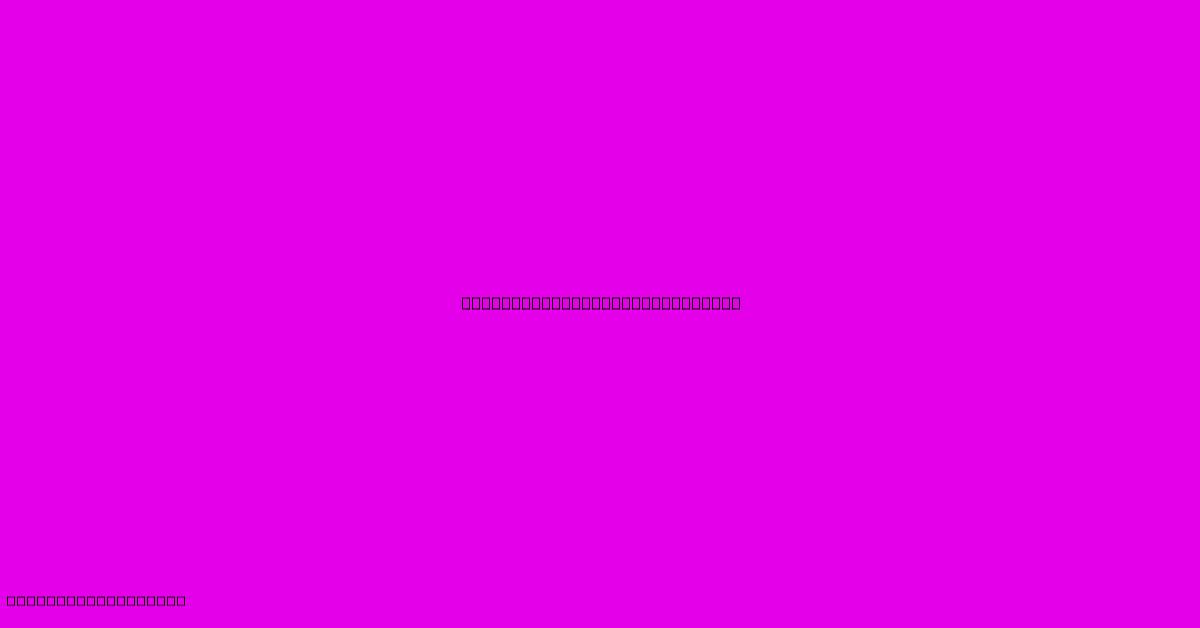
Discover more in-depth information on our site. Click the link below to dive deeper: Visit the Best Website meltwatermedia.ca. Make sure you don’t miss it!
Table of Contents
Unveiling Forward Averaging: A Deep Dive into its Definition and Applications
Hook: Have you ever wondered how financial analysts predict future trends with accuracy? A powerful technique, forward averaging, plays a pivotal role in forecasting future values by leveraging past data. This method offers valuable insights for a diverse range of applications, from financial modeling to weather prediction.
Editor's Note: This comprehensive guide to forward averaging was published today.
Relevance & Summary: Understanding forward averaging is crucial for anyone working with time series data, particularly in finance, economics, and meteorology. This article provides a detailed definition, explores its methodology, discusses its advantages and disadvantages, and presents real-world applications. Keywords include: forward averaging, moving average, forecasting, time series analysis, prediction, weighted average, exponential smoothing, trend analysis, financial forecasting, weather prediction.
Analysis: This guide synthesizes information from established statistical and financial literature, alongside practical examples to illustrate the concepts effectively. The focus is on clarifying the mechanics and applications of forward averaging, rather than delving into complex mathematical proofs.
Key Takeaways:
- Forward averaging predicts future values based on a weighted average of past data.
- It's particularly useful when identifying trends and smoothing out short-term fluctuations.
- The choice of averaging period significantly impacts the accuracy of predictions.
- Limitations include potential lag in identifying sudden shifts and sensitivity to outliers.
- Effective application requires careful consideration of the data's characteristics and the desired forecasting horizon.
Forward Averaging: A Detailed Examination
Introduction
Forward averaging, a fundamental technique in time series analysis, involves calculating a weighted average of past data points to predict future values. Unlike simple moving averages which consider past data, forward averaging focuses on predicting future outcomes. This methodology is valuable for identifying underlying trends and mitigating the impact of short-term noise or volatility within datasets. Its applications span diverse fields, including financial modeling, demand forecasting, and weather prediction.
Key Aspects of Forward Averaging
Several key aspects are essential to understanding and applying forward averaging effectively:
-
Averaging Period: This refers to the number of past data points used in the calculation. A longer averaging period smooths out short-term fluctuations but may lag in responding to significant changes in the underlying trend. Conversely, a shorter period reacts more quickly to changes but may be more susceptible to noise.
-
Weighting Scheme: The weights assigned to each past data point determine the emphasis given to different time periods. Equal weights result in a simple average, while unequal weights can be used to emphasize more recent data (giving more weight to recent observations) or older data.
-
Forecasting Horizon: This denotes the time period into the future that the forecast is made for. A longer forecasting horizon generally reduces prediction accuracy.
Discussion: Applications and Practical Considerations
The application of forward averaging is heavily influenced by the specific context. Let's consider some illustrative scenarios:
Financial Forecasting: In stock market analysis, forward averaging can be used to predict future stock prices based on historical closing prices. However, the effectiveness is subject to market volatility and unforeseen events. Analysts may employ weighted schemes, giving greater weight to recent price movements.
Weather Prediction: Meteorological agencies use forward averaging techniques in combination with other models to predict future temperatures, rainfall, and wind speeds. The choice of averaging period considers the characteristic time scales of weather patterns.
Demand Forecasting: Businesses use forward averaging to predict future sales or demand for their products. Data on past sales, seasonality, and marketing campaigns can be incorporated to improve forecasting accuracy. However, the method's performance depends heavily on the stationarity of the demand pattern. Sudden changes in consumer preference or economic downturns can render the predictions less reliable.
The Role of Weighted Averaging in Forward Averaging
Introduction
The flexibility of forward averaging is significantly enhanced by the incorporation of weighted averages. This allows for a more nuanced approach to forecasting, allowing analysts to incorporate their knowledge about the relative importance of different data points.
Facets of Weighted Averaging in Forward Averaging:
-
Role of Weights: Weights allow for the prioritization of specific data points. For instance, in financial forecasting, recent data might be weighted more heavily than older data, as recent trends often prove more indicative of immediate future developments.
-
Examples: A simple weighted average could assign weights of 0.5, 0.3, and 0.2 to the three most recent data points, giving greater importance to the most recent observation.
-
Risks and Mitigations: Assigning inappropriate weights can lead to biased predictions. Rigorous testing and validation are crucial to mitigate these risks. Careful consideration of the dataset's characteristics is also paramount.
-
Impacts and Implications: Effective weight selection can dramatically improve forecasting accuracy. However, poorly chosen weights can lead to inaccurate predictions and misguided decisions.
Summary
Weighted averages empower forward averaging with a more sophisticated and adaptive approach to forecasting. By tailoring the weights to reflect the underlying dynamics of the data, analysts can significantly enhance the accuracy and robustness of their predictions.
Limitations of Forward Averaging
Introduction
Despite its versatility, forward averaging has inherent limitations that must be carefully considered. Understanding these constraints is crucial for avoiding misinterpretations and developing robust forecasting strategies.
Further Analysis: Addressing Challenges
-
Lagging Indicators: Forward averaging inherently lags behind sudden shifts or discontinuities in the data. This makes it less effective for predicting abrupt changes.
-
Sensitivity to Outliers: Extreme values (outliers) can disproportionately influence the average, leading to inaccurate predictions. Robust statistical techniques may be necessary to mitigate this issue.
-
Stationarity Assumption: Forward averaging generally assumes that the underlying pattern of the time series is stationary (i.e., its statistical properties do not change over time). Non-stationary data can severely compromise the accuracy of forecasts.
Closing
While forward averaging is a valuable tool, its limitations underscore the need for a comprehensive approach to forecasting that incorporates other methods and techniques, alongside a thorough understanding of the data being analyzed. This approach improves the quality of the forecasting process and mitigates the risks associated with overreliance on a single forecasting technique.
FAQ: Forward Averaging
Introduction
This section addresses common questions regarding forward averaging.
Questions:
-
Q: What is the difference between forward averaging and backward averaging (moving average)? A: Forward averaging predicts future values, while backward averaging (moving average) smooths past data.
-
Q: How do I choose the appropriate averaging period? A: The optimal period depends on the characteristics of the data and the forecasting horizon. Experimentation and validation are typically required.
-
Q: Can forward averaging be used for non-stationary data? A: While not ideal, techniques like differencing can help stabilize non-stationary data before applying forward averaging.
-
Q: What are the key assumptions underlying forward averaging? A: Key assumptions include the existence of a discernible trend, relative stationarity of the data, and the relevance of past data in predicting the future.
-
Q: How can I assess the accuracy of forward averaging forecasts? A: Evaluation metrics like Mean Absolute Error (MAE), Root Mean Squared Error (RMSE), and Mean Absolute Percentage Error (MAPE) can assess accuracy.
-
Q: Is forward averaging suitable for all types of data? A: No, forward averaging is most effective for data exhibiting a clear trend and relative stability.
Summary
This FAQ section highlights the need for careful consideration of the data and context when applying forward averaging.
Tips for Effective Forward Averaging
Introduction
This section provides practical tips for optimizing the application of forward averaging.
Tips:
-
Data Preprocessing: Clean and prepare the data by handling missing values and outliers.
-
Averaging Period Selection: Experiment with different averaging periods to find the optimal balance between responsiveness and smoothing.
-
Weighting Scheme Optimization: Consider using weighted averages to adjust the emphasis given to different data points.
-
Model Validation: Rigorously validate the model using appropriate metrics and techniques.
-
Combining with Other Methods: Combine forward averaging with other forecasting methods for improved accuracy.
-
Regular Monitoring and Adjustment: Continuously monitor the forecast accuracy and adapt the model as needed.
Summary
By following these tips, practitioners can significantly enhance the effectiveness and reliability of their forward averaging forecasts.
Summary: Forward Averaging
This article presented a comprehensive exploration of forward averaging, from its fundamental definition to its practical applications and limitations. The discussion emphasized the importance of careful data preparation, appropriate averaging period selection, and model validation. The use of weighted averages to enhance forecasting accuracy was also highlighted.
Closing Message
Forward averaging, while not a panacea for all forecasting challenges, remains a valuable tool in the analyst's arsenal. By understanding its strengths and weaknesses, and employing the strategies outlined, researchers and practitioners can leverage its power to gain valuable insights from time series data and make more informed predictions about the future.
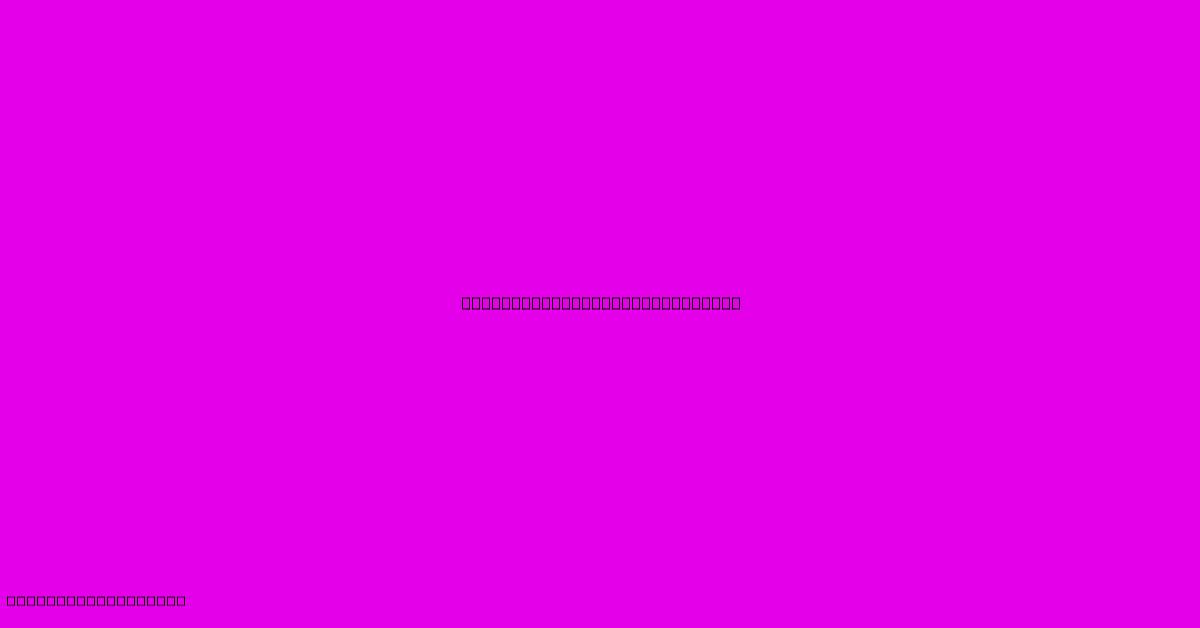
Thank you for taking the time to explore our website Forward Averaging Definition. We hope you find the information useful. Feel free to contact us for any questions, and don’t forget to bookmark us for future visits!
We truly appreciate your visit to explore more about Forward Averaging Definition. Let us know if you need further assistance. Be sure to bookmark this site and visit us again soon!
Featured Posts
-
Mandatory Mortgage Lock Definition
Jan 09, 2025
-
Inorganic Growth Definition How It Arises Methods And Example
Jan 09, 2025
-
Hashgraph Consensus Definition
Jan 09, 2025
-
How To Be An Independent Insurance Adjuster
Jan 09, 2025
-
Government Accountability Office Gao Definition
Jan 09, 2025