How Do You Calculate The Correlation Between The Cash Prices And Each Of The Futures Contracts
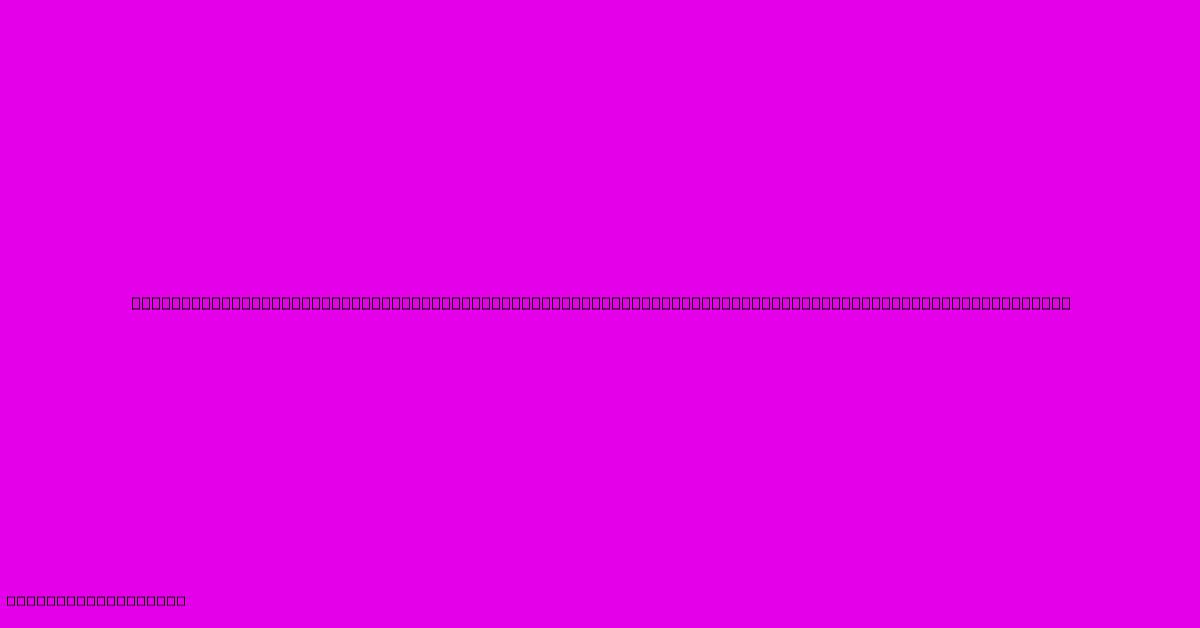
Discover more in-depth information on our site. Click the link below to dive deeper: Visit the Best Website meltwatermedia.ca. Make sure you don’t miss it!
Table of Contents
Unveiling the Correlation: Cash Prices and Futures Contracts
Hook: Does the price of a futures contract accurately reflect the anticipated price of the underlying cash commodity? Understanding the correlation between these two is crucial for effective risk management and trading strategies. A strong understanding of this relationship unlocks significant opportunities within the commodities market.
Editor's Note: This analysis of calculating the correlation between cash prices and futures contracts has been published today.
Relevance & Summary: For traders, investors, and anyone involved in commodity markets, understanding the relationship between cash and futures prices is paramount. This analysis explores various methods for calculating correlation, focusing on statistical measures and practical considerations. The summary covers correlation coefficients, regression analysis, and the impact of factors like time horizons, contract specifications, and market efficiency.
Analysis: This guide uses publicly available market data, statistical software (like R or Python), and established econometric techniques to analyze the correlation. The approach incorporates time series analysis to account for the temporal dependencies inherent in commodity price data. Different correlation measures will be explored, highlighting their strengths and limitations in the context of cash and futures price dynamics.
Key Takeaways:
- Correlation coefficients (Pearson, Spearman) quantify the linear and monotonic relationships, respectively.
- Regression analysis helps understand the predictive power of futures prices on cash prices.
- Market microstructure factors impact observed correlations.
- Time horizon significantly influences the correlation strength.
- Basis risk must be considered when interpreting correlations.
Transition: The following sections will delve into the specifics of calculating the correlation, exploring different methodologies and their implications.
Calculating the Correlation: Cash Prices and Futures Contracts
Introduction: This section details the process of calculating the correlation between cash prices and futures contracts. Accurate calculation depends on data quality, selection of appropriate statistical methods, and an awareness of market nuances.
Key Aspects: The analysis uses historical data for both cash and futures prices. Data cleaning and preprocessing are crucial steps to ensure data integrity. The choice of correlation measure (Pearson, Spearman, Kendall) depends on the nature of the relationship anticipated. Regression analysis provides further insights into the predictive power of futures prices on cash prices.
Discussion:
Data Acquisition and Preparation: Obtain historical price data from reputable sources for both the cash market and the relevant futures contracts. This usually involves collecting daily closing prices over a sufficient period (at least a year, ideally longer for more robust results). Clean the data by handling missing values (through imputation or removal), outliers (through winsorization or trimming), and any data inconsistencies. Ensure that the data is properly aligned in terms of time.
Correlation Coefficient Calculation:
-
Pearson Correlation: This measures the linear relationship between the two price series. A value of +1 indicates perfect positive correlation, -1 perfect negative correlation, and 0 no linear correlation. It's calculated as:
r = Σ[(xi - x̄)(yi - ȳ)] / √[Σ(xi - x̄)²Σ(yi - ȳ)²]
where:
- xi and yi are individual data points for cash and futures prices, respectively.
- x̄ and ȳ are the mean values of cash and futures prices.
-
Spearman Rank Correlation: This measures the monotonic relationship, regardless of linearity. It's less sensitive to outliers than Pearson correlation. It's calculated by ranking the data points and then applying the Pearson formula to the ranks.
-
Kendall's Tau Correlation: Another non-parametric measure of monotonic association, often preferred when the data contains many ties (repeated values).
Regression Analysis: Regression analysis helps determine the relationship's strength and direction, plus predictive capabilities. A simple linear regression model can be used:
Cash Price = β0 + β1 * Futures Price + ε
Where:
- β0 is the intercept.
- β1 is the slope coefficient (representing the change in cash price for a unit change in futures price).
- ε is the error term.
The R-squared value from this regression indicates the proportion of variance in cash prices explained by futures prices. A higher R-squared suggests a stronger predictive relationship.
Basis Risk and Market Microstructure
Introduction: Basis risk, the difference between cash and futures prices, significantly influences the correlation. Market microstructure factors like bid-ask spreads and trading frequency also impact the observed correlation.
Facets:
-
Basis Risk: The basis (Cash Price - Futures Price) is rarely constant. Changes in storage costs, transportation costs, and quality differences between the physical commodity and the contract specifications can affect the basis. High basis risk reduces the observed correlation.
-
Market Microstructure: Transaction costs, order flow, and market depth can influence prices, creating noise in the data and potentially weakening the correlation. High-frequency trading can also impact the correlation.
-
Market Efficiency: In an efficient market, futures prices accurately reflect market expectations of future cash prices. This should lead to a high correlation. Deviations from high correlation suggest market inefficiencies or other factors at play.
-
Time Horizon: Short-term correlations might be weaker due to greater noise and less predictable factors. Longer-term correlations generally show a stronger relationship.
Summary: Basis risk and market microstructure effects complicate the interpretation of correlation. These factors must be considered when analyzing the results and drawing conclusions about the relationship between cash and futures prices.
Time Horizon's Influence on Correlation
Introduction: The correlation between cash and futures prices varies significantly depending on the time horizon considered. This section explores this dependency and its implications.
Further Analysis: Short-term correlations can be heavily influenced by short-term market sentiment and speculative trading, leading to less consistent relationships. Longer-term correlations often reflect the fundamental supply and demand dynamics of the underlying commodity.
Closing: Understanding the time horizon’s influence is critical for interpreting correlation results and developing appropriate trading or hedging strategies. A strategy based on a short-term correlation might fail to capture the long-term relationship between cash and futures.
FAQ: Calculating Correlation Between Cash and Futures Prices
Introduction: This section addresses frequently asked questions concerning the calculation of correlation between cash and futures prices.
Questions:
-
Q: What data frequency should be used? A: Daily data is commonly used, but weekly or monthly data can also be employed depending on the research question and data availability.
-
Q: How much data is sufficient? A: At least one year of data is recommended, with longer periods offering more robust results.
-
Q: What if there are missing data points? A: Missing data can be handled through imputation techniques (e.g., linear interpolation) or by removing the affected periods, but these choices can introduce biases.
-
Q: What if the futures contract expires? A: The analysis should be limited to the period before the contract's expiry. One can then use a different contract that replaces it in the market.
-
Q: How do I account for different contract specifications? A: Ensure that you are comparing cash prices to the most relevant futures contract (e.g., location, quality).
-
Q: How to interpret a low correlation? A: A low correlation might reflect high basis risk, market inefficiencies, or the influence of factors not explicitly considered in the analysis.
Summary: The choice of methodology and data handling techniques significantly impacts the calculated correlation. Careful consideration of these issues is essential for accurate and meaningful results.
Transition: The next section offers practical tips for improving the accuracy and interpretation of correlation analysis.
Tips for Accurate Correlation Analysis
Introduction: This section provides practical tips for conducting more accurate and informative correlation analysis between cash and futures prices.
Tips:
-
Data Validation: Carefully validate data sources, ensuring consistency and accuracy.
-
Outlier Treatment: Address outliers appropriately (winsorization, trimming).
-
Appropriate Correlation Measure: Choose the correlation measure based on the presumed nature of the relationship (linear vs. monotonic).
-
Consider Basis Risk: Explicitly analyze and account for the basis to understand its contribution to the overall correlation.
-
Regression Diagnostics: Conduct thorough diagnostic checks on the regression model to ensure its validity (e.g., check for heteroscedasticity, autocorrelation).
-
Robustness Checks: Perform sensitivity analysis to assess the results' robustness to different data periods, correlation measures, and data cleaning techniques.
-
Contextual Interpretation: Always interpret correlation in the context of the market and any relevant economic factors.
Summary: By following these tips, you can strengthen the accuracy and improve the interpretation of correlation analysis between cash and futures prices.
Summary of Correlation Analysis: Cash and Futures Prices
Summary: This analysis explored methods for calculating the correlation between cash and futures prices. Various correlation coefficients and regression analysis were discussed, along with considerations for basis risk, market microstructure effects, and the influence of time horizons. The importance of data quality and appropriate methodology was emphasized.
Closing Message: Understanding the dynamic relationship between cash and futures prices is vital for effective risk management, price forecasting, and profitable trading strategies in commodity markets. Ongoing monitoring and refinement of analysis techniques are necessary given the ever-evolving nature of these markets.
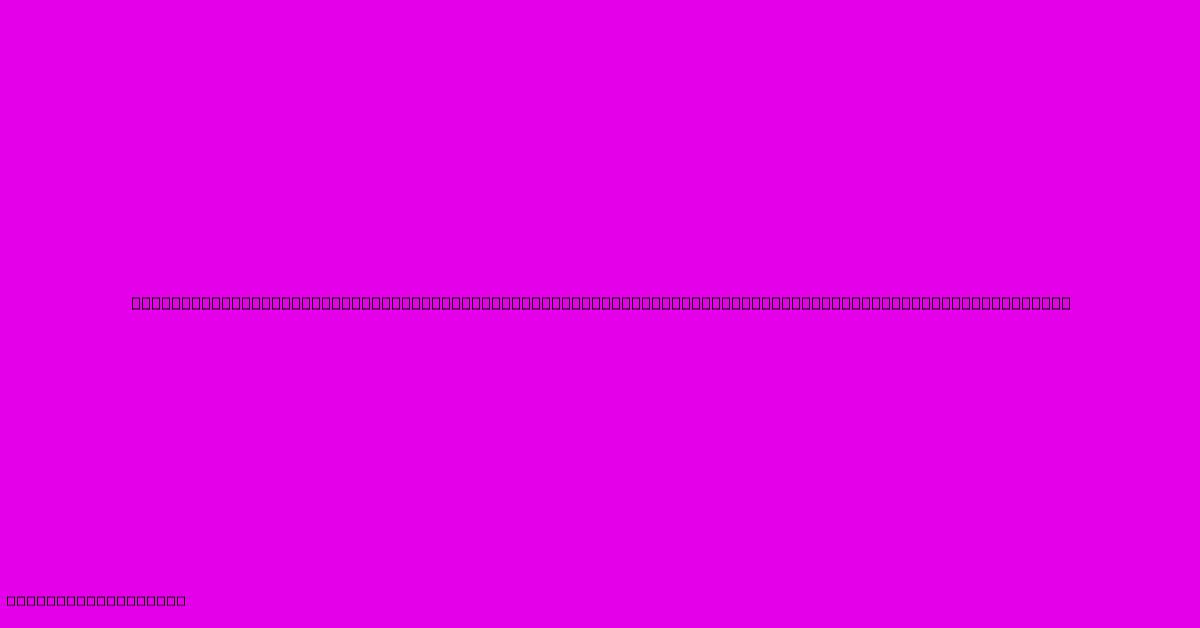
Thank you for taking the time to explore our website How Do You Calculate The Correlation Between The Cash Prices And Each Of The Futures Contracts. We hope you find the information useful. Feel free to contact us for any questions, and don’t forget to bookmark us for future visits!
We truly appreciate your visit to explore more about How Do You Calculate The Correlation Between The Cash Prices And Each Of The Futures Contracts. Let us know if you need further assistance. Be sure to bookmark this site and visit us again soon!
Featured Posts
-
Labor Union Definition History And Examples
Jan 08, 2025
-
Linear Price Scale Definition
Jan 08, 2025
-
Forex E Book Definition
Jan 08, 2025
-
Long Leg Definition
Jan 08, 2025
-
How Should Your Student Loan Payment Compare With Your Salary After Graduating College
Jan 08, 2025