Joint Probability Definition Formula And Example
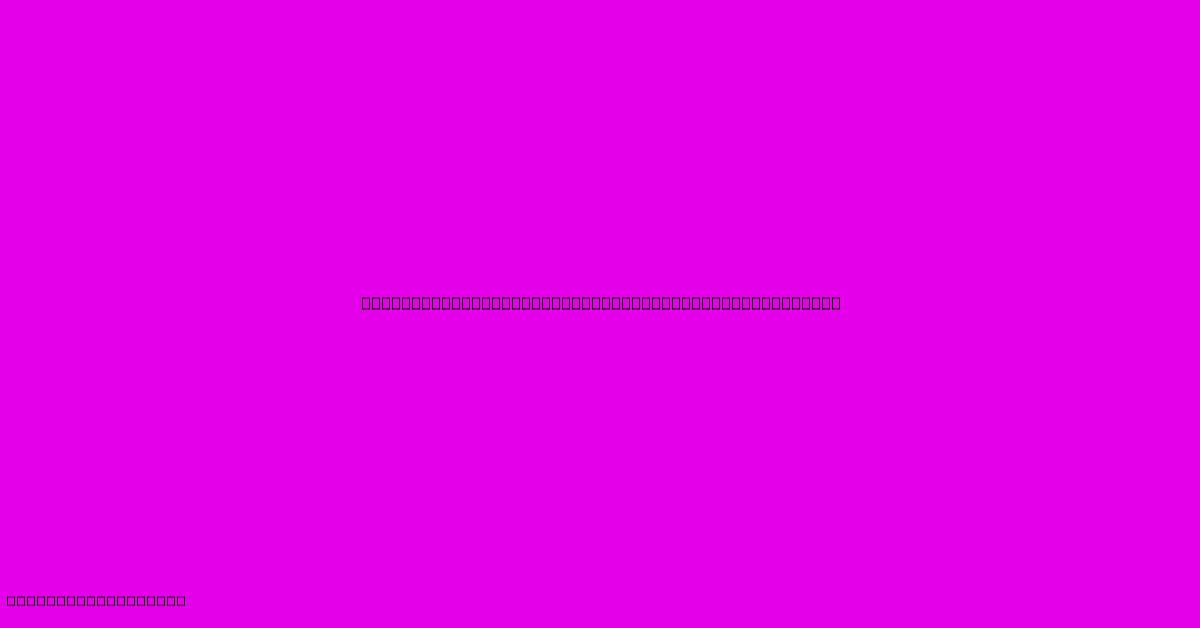
Discover more in-depth information on our site. Click the link below to dive deeper: Visit the Best Website meltwatermedia.ca. Make sure you don’t miss it!
Table of Contents
Unveiling Joint Probability: Definition, Formula, and Illuminating Examples
Hook: Have you ever wondered how likely it is for two independent events to occur simultaneously? Understanding joint probability is crucial for accurately assessing such occurrences across various fields, from finance to medicine.
Editor's Note: This comprehensive guide to joint probability has been published today.
Relevance & Summary: Joint probability is a fundamental concept in probability theory, providing insights into the likelihood of multiple events happening together. This guide explores its definition, formula, and various applications through illustrative examples. It covers conditional probability, independence, and the crucial distinctions between joint and marginal probabilities, using terms like probability distributions, sample spaces, and events.
Analysis: This guide is based on established principles of probability theory and statistical analysis. The examples presented are carefully chosen to illustrate different scenarios and nuances related to joint probability calculations. The methodology employed emphasizes clarity and practical application.
Key Takeaways:
- Joint probability measures the chance of multiple events occurring concurrently.
- The formula differs based on whether events are independent or dependent.
- Conditional probability is crucial when events are not independent.
- Understanding joint probability allows for better risk assessment and decision-making.
- Joint probability applications are widespread in various fields.
Joint Probability: A Deep Dive
Subheading: Joint Probability
Introduction: Joint probability quantifies the likelihood of two or more events happening at the same time. It is a cornerstone of probability theory, with applications ranging from predicting market trends to evaluating medical diagnoses. Understanding its nuances is key to interpreting complex scenarios and making informed decisions.
Key Aspects: The key aspects of joint probability involve understanding the relationship between events (independent vs. dependent), correctly applying the appropriate formula, and interpreting the result within the given context. The concept is closely tied to conditional probability and marginal probability.
Discussion: Let's start with the simplest scenario: two independent events. The joint probability of two independent events A and B is calculated by multiplying their individual probabilities:
P(A and B) = P(A) * P(B)
This formula only holds when the events are independent, meaning the occurrence of one event does not influence the probability of the other. However, many real-world events are not independent. In cases where events A and B are dependent, the joint probability is defined using conditional probability:
P(A and B) = P(A|B) * P(B) or P(A and B) = P(B|A) * P(A)
Where P(A|B) represents the probability of event A occurring given that event B has already occurred (and vice-versa for P(B|A)). This formula accounts for the influence one event has on the other.
Subheading: Conditional Probability and its Role in Joint Probability
Introduction: Conditional probability is intrinsically linked to joint probability, especially when dealing with dependent events. It represents the probability of an event occurring given that another event has already taken place.
Facets:
- Role: Conditional probability allows for a more accurate calculation of joint probability when events are not independent. It accounts for the impact of one event on the likelihood of the other.
- Examples: Consider drawing cards from a deck without replacement. The probability of drawing a king on the second draw depends on whether a king was drawn on the first draw.
- Risks and Mitigations: Incorrectly assuming independence when events are dependent can lead to significant errors in probability calculations. Careful consideration of the relationships between events is crucial.
- Impacts and Implications: Understanding conditional probability is essential in various fields like risk management (insurance, finance), medical diagnosis, and machine learning.
Summary: The concept of conditional probability refines the calculation of joint probability, enabling accurate assessments in situations where the occurrence of one event impacts the probability of another. This is crucial for realistic modeling of many real-world phenomena.
Subheading: Independent vs. Dependent Events in Joint Probability
Introduction: The relationship between events (independent or dependent) fundamentally affects how joint probability is calculated. Understanding this distinction is critical for accurate modeling.
Further Analysis: Independent events are those where the occurrence of one event does not affect the probability of the other. Dependent events, on the other hand, show an influence; the outcome of one event impacts the likelihood of the other occurring.
Closing: Recognizing whether events are independent or dependent dictates the appropriate formula for calculating joint probability. This distinction ensures the accuracy of probabilistic predictions and inferences.
Illustrative Examples of Joint Probability Calculations
Example 1: Independent Events
Suppose there's a 60% chance of rain (Event A) and a 30% chance of a traffic jam (Event B). Assuming these events are independent, the joint probability of both rain and a traffic jam is:
P(A and B) = P(A) * P(B) = 0.6 * 0.3 = 0.18 or 18%
Example 2: Dependent Events
Consider drawing two cards from a standard deck without replacement. What is the probability of drawing two aces?
The probability of drawing an ace on the first draw is 4/52. Given that an ace has been drawn, the probability of drawing another ace on the second draw is 3/51 (since there are only 3 aces left and 51 cards total). Therefore, the joint probability is:
P(Ace and Ace) = P(Ace1) * P(Ace2|Ace1) = (4/52) * (3/51) ≈ 0.0045 or 0.45%
FAQ: Joint Probability
Introduction: This section addresses frequently asked questions about joint probability.
Questions:
-
Q: What is the difference between joint and marginal probability?
- A: Joint probability considers the likelihood of multiple events occurring simultaneously, while marginal probability focuses on the likelihood of a single event regardless of others.
-
Q: Can joint probability be greater than 1?
- A: No, probability values always range from 0 to 1. A value greater than 1 indicates an error in calculation.
-
Q: How is joint probability used in Bayesian inference?
- A: Joint probability is fundamental in Bayesian inference, used to update prior beliefs based on new evidence through Bayes' theorem.
-
Q: What are some real-world applications of joint probability?
- A: Risk assessment, medical diagnostics, machine learning, and financial modeling.
-
Q: How does joint probability relate to the concept of covariance?
- A: Covariance measures the relationship between two random variables, while joint probability measures the probability of their simultaneous occurrence.
-
Q: Can joint probability be visualized?
- A: Yes, using tools like Venn diagrams, contingency tables, or probability distributions.
Summary: These FAQs highlight key concepts and practical applications of joint probability.
Transition: Let's move on to practical tips for mastering joint probability calculations.
Tips for Mastering Joint Probability
Introduction: This section offers practical advice for effectively understanding and applying joint probability.
Tips:
- Clearly define events: Carefully state the events in question, ensuring unambiguous definitions.
- Identify dependence: Determine if the events are independent or dependent; this is crucial for choosing the correct formula.
- Use appropriate notation: Use consistent and clear notation for events and probabilities.
- Visual aids: Use diagrams (Venn diagrams, tree diagrams) to help visualize the problem.
- Check your work: Always verify your calculations to avoid errors.
- Practice: Work through various examples to build confidence and understanding.
Summary: By following these tips, you can significantly improve your proficiency in calculating and interpreting joint probabilities.
Transition: This guide has explored the definition, formula, and applications of joint probability.
Summary of Joint Probability
Summary: This guide provides a comprehensive overview of joint probability, covering its definition, formula for both independent and dependent events, and practical applications through illustrative examples. The importance of understanding conditional probability and the distinction between independent and dependent events have been highlighted.
Closing Message: Mastering joint probability is crucial for accurate risk assessment, informed decision-making, and a deeper understanding of probabilistic models across diverse fields. Continued exploration and practical application will solidify this fundamental concept in probability theory.
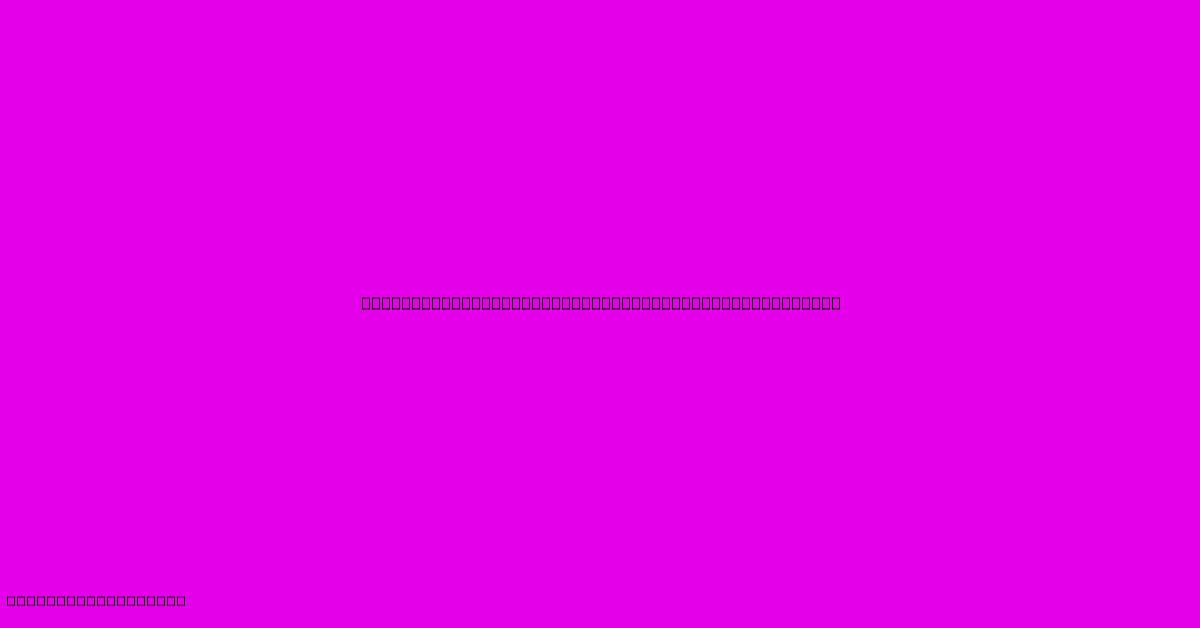
Thank you for taking the time to explore our website Joint Probability Definition Formula And Example. We hope you find the information useful. Feel free to contact us for any questions, and don’t forget to bookmark us for future visits!
We truly appreciate your visit to explore more about Joint Probability Definition Formula And Example. Let us know if you need further assistance. Be sure to bookmark this site and visit us again soon!
Featured Posts
-
How Much Are Cortisone Shots Without Insurance
Jan 09, 2025
-
In Play Definition
Jan 09, 2025
-
Interest Rate Swap Definition Types And Real World Example
Jan 09, 2025
-
Irs Publication 590 Individual Retirement Arrangements Iras Definition
Jan 09, 2025
-
The Different Types Of Mutual Funds
Jan 09, 2025