Leptokurtic Distributions Definition Example Vs Platykurtic
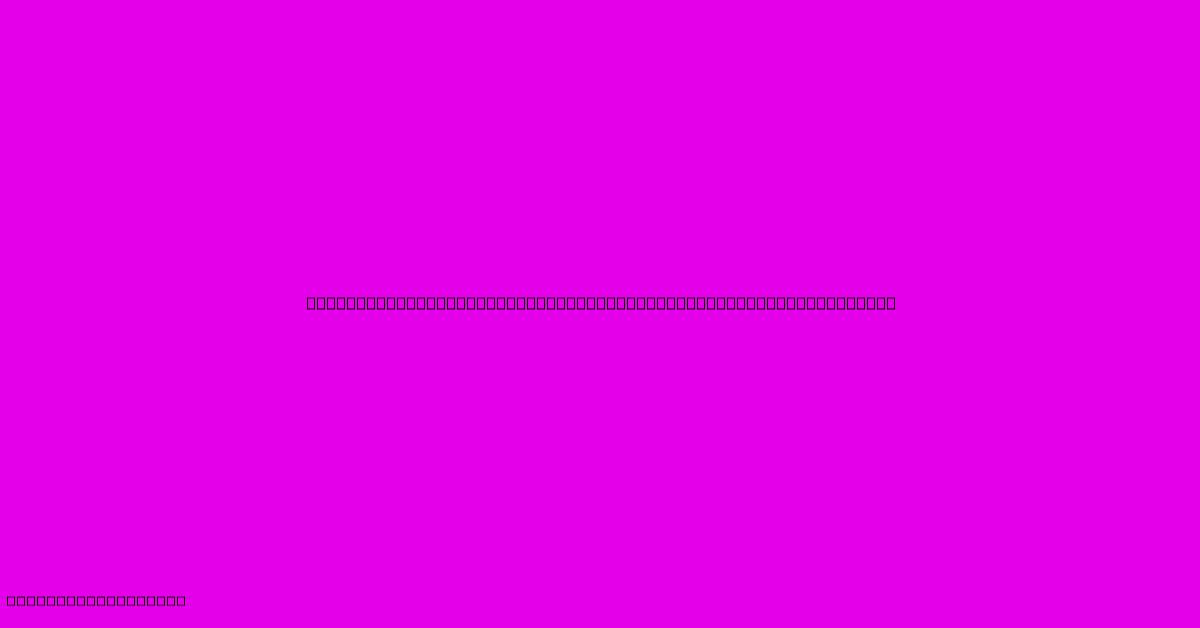
Discover more in-depth information on our site. Click the link below to dive deeper: Visit the Best Website meltwatermedia.ca. Make sure you don’t miss it!
Table of Contents
Unveiling Leptokurtic Distributions: A Deep Dive with Examples and Platykurtic Comparisons
Hook: Have you ever encountered data sets with unexpectedly high peaks and heavy tails? This phenomenon often points to a leptokurtic distribution – a statistical concept with significant implications across various fields. Understanding leptokurtosis is crucial for accurately interpreting data and making informed decisions.
Editor's Note: This comprehensive guide to leptokurtic distributions was published today.
Relevance & Summary: Leptokurtic distributions, characterized by their sharp central peak and heavy tails, differ significantly from the normal distribution. Understanding this difference is vital for accurate statistical modeling, risk assessment, and financial analysis. This article explores the definition of leptokurtosis, provides illustrative examples, and contrasts it with platykurtic distributions, highlighting their key differences and implications. Keywords include: leptokurtosis, kurtosis, platykurtosis, mesokurtosis, statistical distributions, heavy tails, peakedness, risk management, financial modeling.
Analysis: This guide leverages established statistical principles and real-world examples to explain the concept of leptokurtic distributions. It draws upon widely accepted statistical texts and research papers to provide a robust and accurate portrayal of the subject matter.
Key Takeaways:
- Leptokurtic distributions exhibit sharper peaks and heavier tails compared to a normal distribution.
- Kurtosis is a measure quantifying the "tailedness" and "peakedness" of a distribution.
- Leptokurtosis contrasts with platykurtosis (flatter distribution) and mesokurtosis (normal distribution).
- Understanding leptokurtosis is crucial for accurate data interpretation and modeling.
- Real-world examples illustrate the practical significance of leptokurtic distributions.
Transition: Let's now delve into a deeper understanding of leptokurtic distributions, exploring their characteristics, applications, and comparisons with other distribution types.
Leptokurtic Distributions: Definition and Characteristics
Leptokurtic distributions are characterized by a high degree of peakedness (a sharp central peak) and heavy tails. This means a higher concentration of data points around the mean and more extreme values in the tails compared to a normal (mesokurtic) distribution. The term "leptokurtic" originates from the Greek words "leptos" (thin or slender) and "kurtos" (curved or arched), reflecting the distribution's shape.
The measure used to quantify the peakedness and tailedness of a distribution is kurtosis. Kurtosis is a moment of a distribution, typically calculated as the fourth standardized moment. A higher kurtosis value indicates a more leptokurtic distribution. A normal distribution has a kurtosis of 3 (or excess kurtosis of 0). Leptokurtic distributions exhibit kurtosis values greater than 3 (excess kurtosis greater than 0).
Examples of Leptokurtic Distributions
Several real-world phenomena exhibit leptokurtic distributions:
-
Financial Returns: Stock market returns often follow a leptokurtic distribution. This means that while most days see relatively small price changes, there are occasional periods of extreme volatility (large positive or negative returns). These "fat tails" are crucial for risk management in finance, as they highlight the potential for significant losses.
-
Income Distribution: In many societies, income distributions are leptokurtic. A large concentration of individuals fall within a specific income range (the peak), while a smaller number of individuals earn significantly higher or lower incomes (the heavy tails).
-
Natural Disasters: The magnitude of natural disasters (earthquakes, floods, etc.) often follows a leptokurtic distribution. Most events are relatively small, but a few catastrophic events contribute significantly to the overall magnitude.
-
Insurance Claims: Insurance claim amounts often exhibit leptokurtic characteristics. Most claims are relatively small, but a few large claims (e.g., major car accidents, significant property damage) significantly impact the insurer's overall liability.
Leptokurtic vs. Platykurtic Distributions
Platykurtic distributions represent the opposite extreme of leptokurtic distributions. They are characterized by a flat peak and thin tails, indicating a lower concentration of data around the mean and fewer extreme values. Platykurtic distributions have a kurtosis value less than 3 (excess kurtosis less than 0). A uniform distribution is a classic example of a platykurtic distribution.
Here's a table summarizing the key differences:
Feature | Leptokurtic Distribution | Platykurtic Distribution | Mesokurtic Distribution (Normal) |
---|---|---|---|
Peak | Sharp, high | Flat, low | Moderate |
Tails | Heavy | Thin | Moderate |
Kurtosis | > 3 | < 3 | 3 |
Excess Kurtosis | > 0 | < 0 | 0 |
Examples | Financial returns, income | Uniform distribution | Height, weight (approximately) |
Understanding the Implications of Leptokurtosis
Recognizing the leptokurtic nature of a data set has important implications for several areas:
-
Risk Management: In finance, leptokurtosis indicates the possibility of unexpected extreme events (black swan events). Accurate risk assessment requires incorporating these "fat tails" into models.
-
Statistical Modeling: Using incorrect statistical models that assume normality when data is leptokurtic can lead to inaccurate predictions and flawed inferences.
-
Data Analysis: Understanding the shape of the data distribution allows for better interpretation of statistical measures such as the mean and standard deviation.
Point: The Impact of Leptokurtic Distributions on Financial Modeling
Introduction: The presence of leptokurtic distributions in financial data significantly impacts the accuracy and reliability of financial models. Understanding this impact is crucial for effective risk management and investment strategies.
Facets:
-
Role of Heavy Tails: The heavy tails in leptokurtic distributions lead to higher probabilities of extreme events (large gains or losses), which are not captured by models assuming normality.
-
Examples: Value-at-Risk (VaR) models, commonly used in risk management, can underestimate the risk of extreme losses if they don't account for leptokurtosis in financial returns.
-
Risks and Mitigations: Failure to account for leptokurtosis can lead to inaccurate risk assessments, underestimation of potential losses, and inadequate capital allocation. Mitigations include using alternative models that explicitly account for heavy tails, such as GARCH models or stable distributions.
-
Impacts and Implications: Inaccurate financial models can lead to poor investment decisions, insufficient risk mitigation, and potentially significant financial losses.
Summary: The presence of leptokurtosis in financial data necessitates a careful reevaluation of traditional models that assume normality. Failure to account for heavy tails can significantly impact the accuracy of risk assessments and investment strategies.
Point: Applications of Leptokurtic Distribution Analysis in Insurance
Introduction: Understanding leptokurtic distributions is fundamental in insurance for accurate risk assessment and premium calculation. The heavy tails of these distributions play a crucial role.
Further Analysis: In insurance, the frequency and severity of claims often follow leptokurtic distributions. A small number of high-severity claims significantly impact an insurer's liability. Actuaries use statistical models that account for this leptokurtosis to properly assess risk and set appropriate premiums. Ignoring the heavy tails can lead to underestimation of potential losses, jeopardizing the insurer's financial stability.
Closing: Accurate modeling of leptokurtic claim distributions is critical for the solvency and long-term viability of insurance companies.
FAQ
Introduction: This section addresses frequently asked questions about leptokurtic distributions.
Questions:
-
Q: What is the difference between kurtosis and excess kurtosis? A: Kurtosis is a measure of the tailedness and peakedness of a distribution. Excess kurtosis is the kurtosis minus 3, providing a relative comparison to the normal distribution.
-
Q: How do I identify if a distribution is leptokurtic? A: By calculating the kurtosis of the data. A kurtosis greater than 3 (or excess kurtosis greater than 0) indicates leptokurtosis.
-
Q: What are the implications of ignoring leptokurtosis in data analysis? A: Ignoring leptokurtosis can lead to inaccurate statistical inference, misinterpretation of results, and flawed decision-making.
-
Q: What statistical methods are suitable for analyzing leptokurtic data? A: Methods like robust statistics, GARCH models, and stable distributions are often used.
-
Q: Are all financial data sets leptokurtic? A: Not all; some financial data might exhibit platykurtosis or near-normality, depending on the asset class and time period.
-
Q: How does leptokurtosis affect the accuracy of the mean as a central tendency measure? A: The mean can be highly influenced by extreme values in leptokurtic distributions, making it a potentially less representative measure of central tendency than the median.
Summary: Understanding the characteristics and implications of leptokurtosis is essential for correct data interpretation and decision-making across diverse fields.
Transition: Let's now explore some practical tips for dealing with leptokurtic data.
Tips for Handling Leptokurtic Data
Introduction: This section offers practical tips for effectively handling data sets exhibiting leptokurtosis.
Tips:
-
Visual Inspection: Examine the data using histograms and box plots to visually assess the peakedness and tail behavior.
-
Kurtosis Calculation: Quantify the degree of leptokurtosis by calculating the kurtosis and excess kurtosis.
-
Data Transformation: Consider transforming the data (e.g., using logarithmic transformations) to reduce leptokurtosis and improve the applicability of standard statistical methods.
-
Robust Statistical Methods: Employ robust statistical methods less sensitive to extreme values, such as median instead of mean, or trimmed means.
-
Specialized Models: Use statistical models that explicitly account for heavy tails, such as GARCH models or stable distributions.
-
Non-parametric Methods: Consider non-parametric methods which make fewer assumptions about the data distribution.
Summary: Implementing these tips enhances the accuracy and reliability of analyses involving leptokurtic data.
Summary of Leptokurtic Distributions
This article explored leptokurtic distributions, highlighting their defining characteristics, real-world examples, and contrasts with platykurtic distributions. Understanding leptokurtosis is crucial for accurate risk assessment, financial modeling, and various other applications where data exhibits heavy tails and sharp peaks. The implications of ignoring leptokurtosis can be substantial, leading to inaccurate interpretations and flawed decisions.
Closing Message: The careful consideration of leptokurtic distributions is not just a statistical nuance; it is a fundamental aspect of accurately interpreting and modeling data in many fields. Further research into specialized statistical techniques for handling leptokurtic data is encouraged for improved analysis and decision-making.
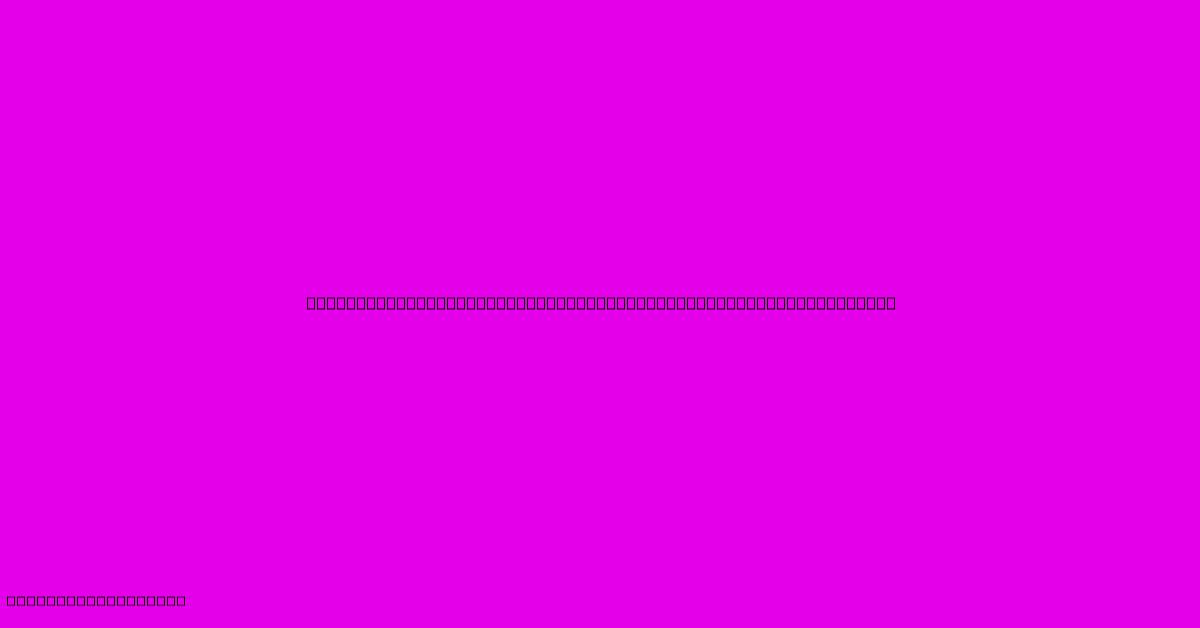
Thank you for taking the time to explore our website Leptokurtic Distributions Definition Example Vs Platykurtic. We hope you find the information useful. Feel free to contact us for any questions, and don’t forget to bookmark us for future visits!
We truly appreciate your visit to explore more about Leptokurtic Distributions Definition Example Vs Platykurtic. Let us know if you need further assistance. Be sure to bookmark this site and visit us again soon!
Featured Posts
-
How Does A Private Pension Work
Jan 08, 2025
-
How Bad Is A 560 Credit Score
Jan 08, 2025
-
How To Check Credit Score Without Ssn
Jan 08, 2025
-
Why Funding Is Important For Startups
Jan 08, 2025
-
How To Use Credit Card Flash Drive
Jan 08, 2025