Linear Relationship Definition
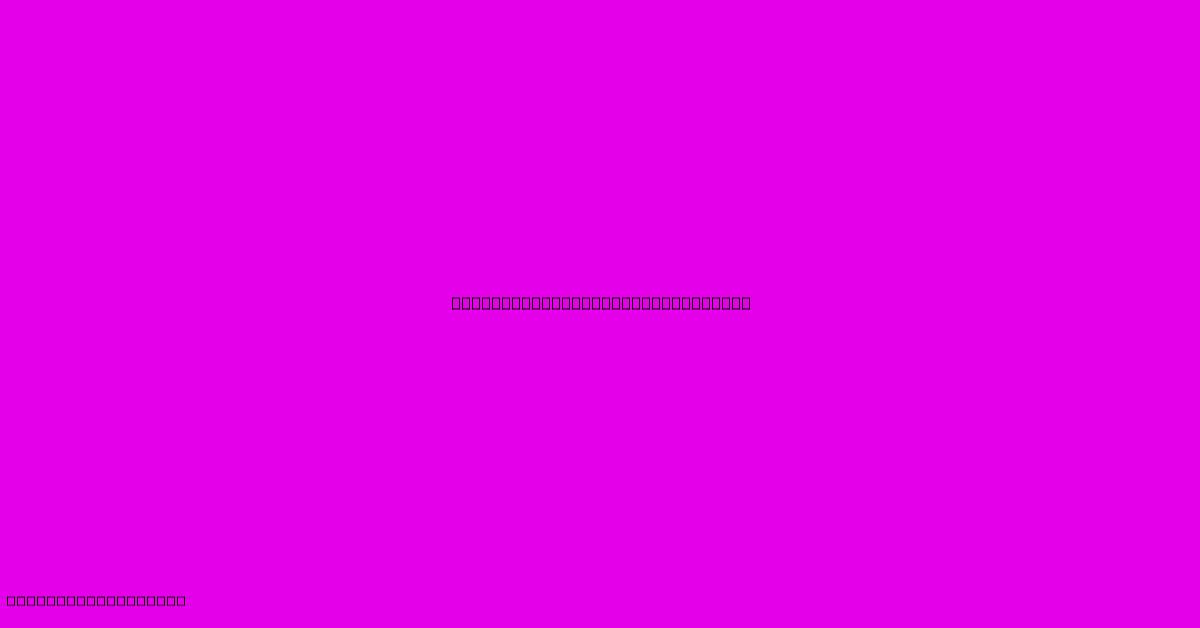
Discover more in-depth information on our site. Click the link below to dive deeper: Visit the Best Website meltwatermedia.ca. Make sure you don’t miss it!
Table of Contents
Unveiling Linear Relationships: A Comprehensive Guide
Hook: Does the strength of a plant's growth directly correlate with the amount of sunlight it receives? A definitive yes points to the crucial role of understanding linear relationships in numerous fields.
Editor's Note: This comprehensive guide to linear relationships has been published today.
Relevance & Summary: Understanding linear relationships is fundamental to interpreting data across various disciplines, from science and engineering to economics and social sciences. This guide provides a detailed exploration of linear relationships, their definition, representation, applications, and limitations. It covers key concepts like slope, intercept, correlation, and regression analysis, using illustrative examples for better comprehension. Keywords include: linear relationship, correlation, regression, slope, intercept, scatter plot, linear equation, dependent variable, independent variable, positive correlation, negative correlation, no correlation.
Analysis: This guide synthesizes information from established statistical textbooks, academic research papers, and reputable online resources to present a clear and concise overview of linear relationships. The information is structured to provide a progressive understanding, starting from basic definitions and moving towards more complex applications.
Key Takeaways:
- Linear relationships describe a constant rate of change between two variables.
- They are visually represented by straight lines on a graph.
- Correlation measures the strength and direction of a linear relationship.
- Regression analysis helps predict one variable based on the other.
- Understanding linear relationships aids in data interpretation and prediction.
Linear Relationships: A Deep Dive
Defining Linear Relationships
A linear relationship exists between two variables when a change in one variable results in a proportional change in the other. This means that the ratio between the changes remains constant. Graphically, a linear relationship is represented by a straight line. The relationship can be either positive (both variables increase together), negative (one variable increases as the other decreases), or nonexistent (no discernible relationship).
The mathematical representation of a linear relationship is typically expressed as a linear equation: y = mx + c
, where:
y
is the dependent variable (its value depends on the value of x).x
is the independent variable (its value is not influenced by y).m
is the slope of the line, representing the rate of change of y with respect to x.c
is the y-intercept, representing the value of y when x is zero.
Key Aspects of Linear Relationships
This section examines several aspects fundamental to understanding linear relationships.
1. Slope (m)
The slope is the crucial indicator of the rate of change in a linear relationship. A positive slope indicates a positive relationship (as x increases, y increases), while a negative slope signifies a negative relationship (as x increases, y decreases). A slope of zero indicates no relationship between the variables (the line is horizontal). The steeper the slope, the stronger the relationship. Mathematically, the slope is calculated as the change in y divided by the change in x: m = (y₂ - y₁) / (x₂ - x₁)
.
2. Y-Intercept (c)
The y-intercept represents the value of the dependent variable (y
) when the independent variable (x
) is zero. It's the point where the line intersects the y-axis. The significance of the y-intercept depends on the context of the relationship. For instance, in a linear model predicting plant growth, the y-intercept might represent the initial height of the plant.
3. Correlation
Correlation measures the strength and direction of a linear relationship. It's expressed using the correlation coefficient (often denoted as 'r'), which ranges from -1 to +1.
r = +1
: Perfect positive correlation (a strong, positive linear relationship).r = -1
: Perfect negative correlation (a strong, negative linear relationship).r = 0
: No linear correlation (no linear relationship).- Values between -1 and +1 indicate varying degrees of linear correlation.
4. Scatter Plots
Scatter plots are graphical representations of data points showing the relationship between two variables. In a linear relationship, the data points tend to cluster around a straight line. The visual inspection of a scatter plot provides a quick assessment of the presence and strength of a linear relationship.
Regression Analysis
Regression analysis is a statistical method used to model the relationship between a dependent variable and one or more independent variables. In the case of a linear relationship, linear regression is applied. Linear regression aims to find the "best-fit" line that minimizes the distance between the data points and the line. The equation of this line provides a model for predicting the value of the dependent variable based on the value of the independent variable.
Applications of Linear Relationships
Linear relationships find widespread applications across various fields:
- Physics: Ohm's law (V = IR) describes a linear relationship between voltage (V), current (I), and resistance (R).
- Engineering: Stress-strain relationships in materials often exhibit linear behavior within the elastic limit.
- Economics: Supply and demand curves are often modeled using linear relationships (though in reality, they are often more complex).
- Finance: Simple interest calculations are based on a linear relationship between principal, interest rate, and time.
- Biology: The relationship between the dosage of a drug and its effect sometimes follows a linear pattern within a certain range.
Limitations of Linear Relationships
It's crucial to acknowledge that not all relationships are linear. Many real-world phenomena exhibit non-linear behavior. Assuming a linear relationship when it's not appropriate can lead to inaccurate predictions and flawed conclusions. Nonlinear relationships often require more sophisticated modeling techniques.
Specific Aspects of Linear Relationships
Slope and its Interpretations
The slope's interpretation depends on the context. In a linear model predicting crop yield (y) based on fertilizer amount (x), a slope of 2 means that for every unit increase in fertilizer, the crop yield increases by 2 units. A negative slope in a model relating temperature (x) to ice cream sales (y) suggests that as temperature increases, ice cream sales decrease.
Facets:
- Role: The slope quantifies the change in the dependent variable for a unit change in the independent variable.
- Examples: Positive slope in sales vs. advertising spend, negative slope in altitude vs. air pressure.
- Risks & Mitigations: Misinterpreting the slope due to confounding variables; using appropriate statistical methods to control for confounding factors.
- Impacts & Implications: The slope's value dictates the strength and direction of the linear relationship; it is crucial for prediction and decision-making.
Correlation vs. Causation
A correlation between two variables does not imply causation. Just because two variables exhibit a linear relationship doesn't necessarily mean that one causes the other. A third, unobserved variable could be influencing both. For example, a strong positive correlation between ice cream sales and drowning incidents doesn't mean ice cream causes drowning; both are likely influenced by the common factor of hot weather.
Further Analysis: Observational studies often reveal correlations but cannot establish causation definitively. Controlled experiments are needed to ascertain causal relationships. The absence of correlation doesn't always mean the absence of a relationship, only the absence of a linear one.
Regression Analysis and Prediction
Linear regression provides a method to predict the value of the dependent variable given the independent variable. However, the accuracy of predictions depends on the strength of the correlation and the presence of outliers. Extracting meaningful insight requires considering the context and limitations of the model.
Further Analysis: Residual analysis can be used to assess the accuracy of the model by analyzing the difference between predicted and observed values. Overfitting (modeling noise rather than the underlying relationship) is a potential pitfall.
FAQ: Linear Relationships
Introduction to FAQs
This section addresses common questions concerning linear relationships.
Questions:
- Q: What are the assumptions of linear regression? A: Linearity, independence, normality of residuals, homoscedasticity, and no multicollinearity.
- Q: How do outliers affect linear regression? A: They can unduly influence the slope and intercept of the regression line, leading to biased estimates.
- Q: Can a linear relationship exist between more than two variables? A: Yes, multiple linear regression models the relationship between a dependent variable and multiple independent variables.
- Q: What is the difference between correlation and covariance? A: Covariance measures the direction of the linear relationship, while correlation measures both direction and strength (standardized).
- Q: How can I determine if a relationship is linear? A: Visually inspect a scatter plot, calculate the correlation coefficient, and perform statistical tests.
- Q: What are some common pitfalls to avoid when interpreting linear relationships? A: Confusing correlation with causation, ignoring non-linearity, and overinterpreting the model's predictions.
Summary of FAQs
Understanding the assumptions and limitations of linear regression and correlation analysis is crucial for proper interpretation and prediction.
Tips for Understanding Linear Relationships
Introduction to Tips
This section offers practical advice for understanding and applying knowledge of linear relationships.
Tips:
- Visualize data using scatter plots before performing any statistical analysis.
- Consider potential confounding variables when interpreting correlations.
- Assess the assumptions of linear regression before making inferences.
- Check for outliers and consider their impact on the model.
- Use appropriate statistical software for regression analysis.
- Always interpret the results in the context of the specific problem.
- Be cautious about extrapolating beyond the range of the observed data.
- Remember that correlation does not imply causation.
Summary of Tips
These tips enhance the understanding and practical application of linear relationships, enabling more effective data interpretation and analysis.
Summary of Linear Relationships
This guide provides a comprehensive overview of linear relationships, covering their definition, representation, analysis, and applications. Understanding linear relationships is crucial for interpreting data and making informed decisions across numerous fields.
Closing Message
The study of linear relationships provides a foundational understanding of how variables interact. Continued exploration of statistical methods and their applications will further refine our ability to model and predict real-world phenomena. The insights gained from this exploration will remain valuable tools in scientific discovery, engineering innovation, and economic modeling.
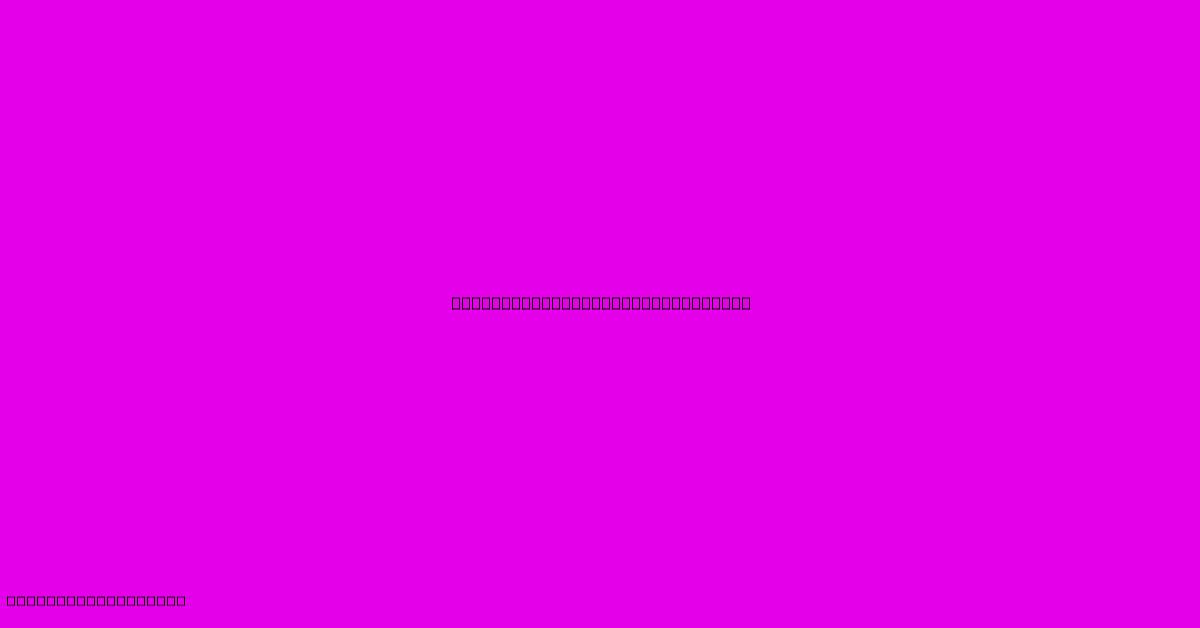
Thank you for taking the time to explore our website Linear Relationship Definition. We hope you find the information useful. Feel free to contact us for any questions, and don’t forget to bookmark us for future visits!
We truly appreciate your visit to explore more about Linear Relationship Definition. Let us know if you need further assistance. Be sure to bookmark this site and visit us again soon!
Featured Posts
-
Lipstick Effect Definition Theory Value As Economic Indicator
Jan 08, 2025
-
Where To Find Net Sales On Financial Statements
Jan 08, 2025
-
Market Capitalization Rule Definition
Jan 08, 2025
-
How Can I Build My Childs Credit Score
Jan 08, 2025
-
Foreign Bond Definition Risks Examples
Jan 08, 2025