Nonaccrual Experience Nae Method Definition
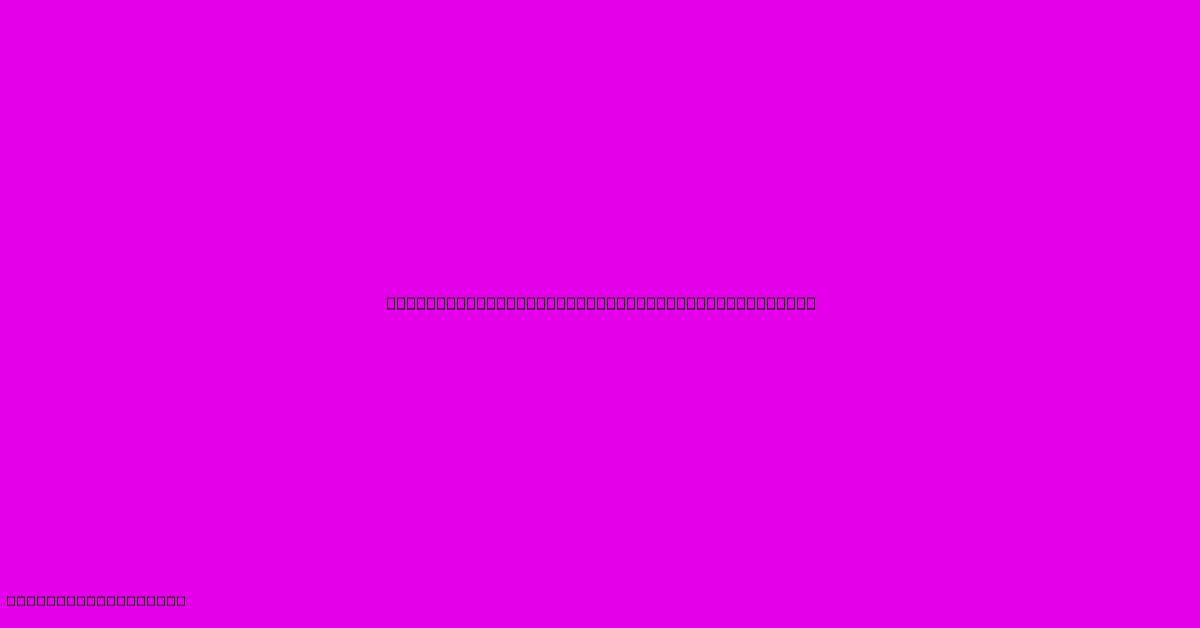
Discover more in-depth information on our site. Click the link below to dive deeper: Visit the Best Website meltwatermedia.ca. Make sure you don’t miss it!
Table of Contents
Unveiling the Mysteries of the Nonaccrual Experience (NAE) Method: A Comprehensive Guide
Hook: Does the seemingly simple act of classifying a loan as nonaccrual hold the key to unlocking a more accurate picture of a bank's financial health? The answer is a resounding yes, and understanding the Nonaccrual Experience (NAE) method is crucial for navigating the complexities of loan portfolio risk assessment.
Editor's Note: This guide to the Nonaccrual Experience (NAE) method was published today.
Relevance & Summary: The Nonaccrual Experience (NAE) method is a critical tool for financial institutions, particularly banks, to assess and manage credit risk. It provides a structured approach to analyzing the historical performance of loans that have been classified as nonaccrual, offering valuable insights into the likelihood of future loan losses and informing more effective risk management strategies. This guide explores the definition, application, and significance of the NAE method, including its key components and practical implications for financial institutions. Understanding NAE is vital for stakeholders including investors, regulators, and bank management to accurately gauge credit risk and evaluate the overall financial stability of a lending institution. This exploration delves into the calculation methods, relevant regulatory considerations, and the limitations of the NAE approach.
Analysis: This guide synthesizes information from established financial reporting standards, industry best practices, and academic research on loan loss provisioning and credit risk management. The analysis focuses on the practical application of the NAE method and its role in informing prudent lending decisions. The aim is to provide a clear and concise explanation suitable for both financial professionals and those seeking a foundational understanding of the subject.
Key Takeaways:
- NAE provides a structured approach to analyzing non-performing loans.
- It helps predict future loan losses and improve risk management.
- It is crucial for assessing the financial health of financial institutions.
- Regulatory compliance often necessitates the use of NAE methodologies.
- Limitations of NAE should be considered for a complete risk assessment.
Transition: The Nonaccrual Experience (NAE) method is not merely a technical accounting procedure; it's a fundamental tool for understanding and managing the inherent risks associated with lending. A deep dive into its components will reveal its power and limitations.
Nonaccrual Experience (NAE) Method: A Deep Dive
Introduction: The NAE method is a statistical approach used to analyze the historical behavior of loans that have been classified as nonaccrual. Nonaccrual loans are those where interest payments are not being received as scheduled, indicating significant credit deterioration. The method's primary purpose is to estimate the expected recovery rate on these loans, which is crucial for determining the appropriate level of loan loss reserves.
Key Aspects: The core components of the NAE method include:
- Loan Data Collection: Gathering comprehensive data on individual nonaccrual loans is the first critical step. This data should include the loan's original balance, the outstanding balance at the time of nonaccrual classification, the passage of time since nonaccrual, and the ultimate recovery amount (if the loan has been fully resolved).
- Grouping and Segmentation: Loans are often grouped based on relevant characteristics such as loan type, borrower industry, credit rating at origination, and collateral type. This segmentation allows for a more nuanced analysis as different loan groups can exhibit vastly different recovery patterns.
- Recovery Rate Calculation: The method involves calculating the recovery rate for each loan or group of loans. This is typically done by dividing the ultimate recovery amount by the loan's balance at the time of nonaccrual.
- Statistical Modeling: Statistical techniques are employed to analyze the historical recovery data and develop a predictive model. This model can take various forms, such as regression analysis or survival analysis. The model aims to estimate the expected recovery rate for future nonaccrual loans, taking into account relevant factors influencing recovery.
- Loan Loss Provisioning: The predicted recovery rates are incorporated into the process of estimating the necessary loan loss reserves. Adequate loan loss reserves protect the bank's financial stability by accounting for potential future loan losses.
Analyzing the Facets of the NAE Method
Data Collection and Quality
Introduction: The accuracy of the NAE method heavily depends on the quality and completeness of the underlying loan data.
Facets:
- Role: Data serves as the foundation for all subsequent calculations and modeling. Inaccurate or incomplete data directly impacts the reliability of the NAE model and the resulting predictions.
- Examples: Missing data points, errors in recording loan balances, inconsistencies in classification criteria, and inaccuracies in recording recovery amounts all represent potential data quality issues.
- Risks and Mitigations: Data quality risks can lead to biased estimates and unreliable predictions. Mitigations include robust data validation processes, thorough data cleansing procedures, and the implementation of rigorous data governance frameworks.
- Impacts and Implications: Poor data quality can result in inadequate loan loss provisioning, leading to increased financial risk for the institution. It can also affect regulatory compliance and erode investor confidence.
Statistical Modeling and Predictive Accuracy
Introduction: The statistical model selected for the NAE method significantly influences the predictive power of the analysis.
Facets:
- Role: The model transforms historical data into actionable insights by estimating future recovery rates. Model selection depends on the characteristics of the data and the desired level of predictive accuracy.
- Examples: Regression analysis, survival analysis (Kaplan-Meier method and Cox proportional hazards model), and machine learning algorithms are commonly employed in NAE modeling. The choice of model depends on the data structure and the complexity of the relationships between predictor variables and the recovery rate.
- Risks and Mitigations: Overfitting the model to the historical data is a significant risk. Mitigations include using appropriate validation techniques such as cross-validation to ensure that the model generalizes well to new data.
- Impacts and Implications: An accurate model leads to more reliable loan loss provisioning and better risk management. An inaccurate model, on the other hand, can lead to underestimated loan losses and increased financial vulnerabilities.
The Interplay Between Recovery Rates and Loan Loss Provisioning
Introduction: The NAE method's output – the predicted recovery rate – directly feeds into the calculation of loan loss provisions.
Further Analysis: The expected loss on a nonaccrual loan is calculated by subtracting the predicted recovery rate from the loan's outstanding balance. The sum of these expected losses for all nonaccrual loans in the portfolio forms the basis for the overall loan loss provision. Regulatory capital requirements often necessitate the use of robust loss estimation methodologies, including the NAE approach.
Closing: Accurately predicting recovery rates is paramount for effective loan loss provisioning. The quality of the data, the selection of an appropriate statistical model, and the understanding of the model's limitations are all vital factors in achieving this. The resulting loan loss reserve strengthens the bank's capital adequacy and supports its financial stability.
FAQ: Nonaccrual Experience Method
Introduction: This section addresses frequently asked questions concerning the NAE method.
Questions:
-
Q: What are the key differences between the NAE method and other loan loss provisioning models? A: While other models might use different inputs or methodologies (e.g., incurred loss models), NAE focuses specifically on the historical experience of nonaccrual loans to estimate recovery rates. This targeted approach offers valuable insights into the specific behavior of impaired loans.
-
Q: How frequently should the NAE model be updated? A: The model should be updated regularly, at least annually, to reflect changes in economic conditions, lending practices, and the characteristics of the nonaccrual loan portfolio. More frequent updates might be necessary in periods of significant economic uncertainty or shifts in the bank's credit risk profile.
-
Q: What are the limitations of the NAE method? A: The NAE method relies on historical data, and past performance is not necessarily indicative of future results. The model's accuracy is also dependent on the quality of the data and the appropriateness of the chosen statistical model. External factors not reflected in the historical data can significantly affect recovery rates.
-
Q: How does regulatory compliance influence the application of NAE? A: Banking regulations often require banks to maintain adequate loan loss reserves and use sound methodologies for estimating potential loan losses. The NAE method, when properly implemented, can meet these regulatory requirements.
-
Q: Can the NAE method be used for all types of loans? A: While the principles of NAE can be applied broadly, the specific model parameters and variables might need to be adjusted based on the characteristics of the loan types. For instance, a model for commercial real estate loans would differ from one for consumer loans.
-
Q: What role does the credit risk department play in NAE? A: The credit risk department plays a crucial role in all aspects of NAE, from data collection and validation to model development and interpretation of the results. Their expertise is vital in ensuring the reliability and relevance of the NAE analysis.
Summary: The NAE method is a powerful tool, but its effective application requires careful attention to data quality, model selection, and an understanding of its inherent limitations.
Transition: Understanding best practices enhances the value of NAE.
Tips for Effective Application of the NAE Method
Introduction: This section provides practical advice for improving the effectiveness of the NAE method.
Tips:
-
Invest in Data Quality: Implement robust data governance policies and ensure accuracy in data collection and validation processes. Clean, reliable data forms the bedrock of effective NAE analysis.
-
Select Appropriate Statistical Models: Choose statistical models that are suitable for the characteristics of the data and the complexity of the relationships being investigated. Model validation is crucial.
-
Segment the Loan Portfolio: Group nonaccrual loans based on relevant characteristics to identify patterns and develop more precise recovery rate estimates. This allows for a more tailored risk assessment for different loan segments.
-
Regular Model Review and Updates: Periodically review and update the NAE model to reflect changes in economic conditions, lending practices, and the composition of the nonaccrual loan portfolio. Staying current prevents model staleness.
-
Consider External Factors: Acknowledge and account for macroeconomic factors, regulatory changes, and industry-specific events that may influence recovery rates. External factors play a significant role and neglecting them introduces bias.
-
Utilize Expert Judgment: Incorporate expert judgment from credit risk professionals to refine the model and improve its predictive accuracy. Human expertise brings a qualitative dimension to the quantitative analysis.
-
Transparency and Documentation: Maintain clear and comprehensive documentation of the NAE methodology, including data sources, model specifications, and assumptions. Transparency fosters accountability and improves model understanding.
Summary: These tips promote more reliable and accurate NAE modeling, leading to improved risk management and more informed decision-making.
Conclusion: Navigating the Landscape of Credit Risk
Summary: This guide has explored the Nonaccrual Experience (NAE) method, highlighting its importance in credit risk assessment and loan loss provisioning. The method's successful application requires attention to data quality, model selection, and the incorporation of relevant external factors.
Closing Message: The NAE method is not a panacea, but rather a critical tool for financial institutions navigating the complexities of credit risk. By understanding its strengths and limitations, institutions can leverage this methodology to enhance their risk management practices and contribute to greater financial stability. Continuous improvement and refinement of the method are crucial in adapting to ever-evolving market conditions.
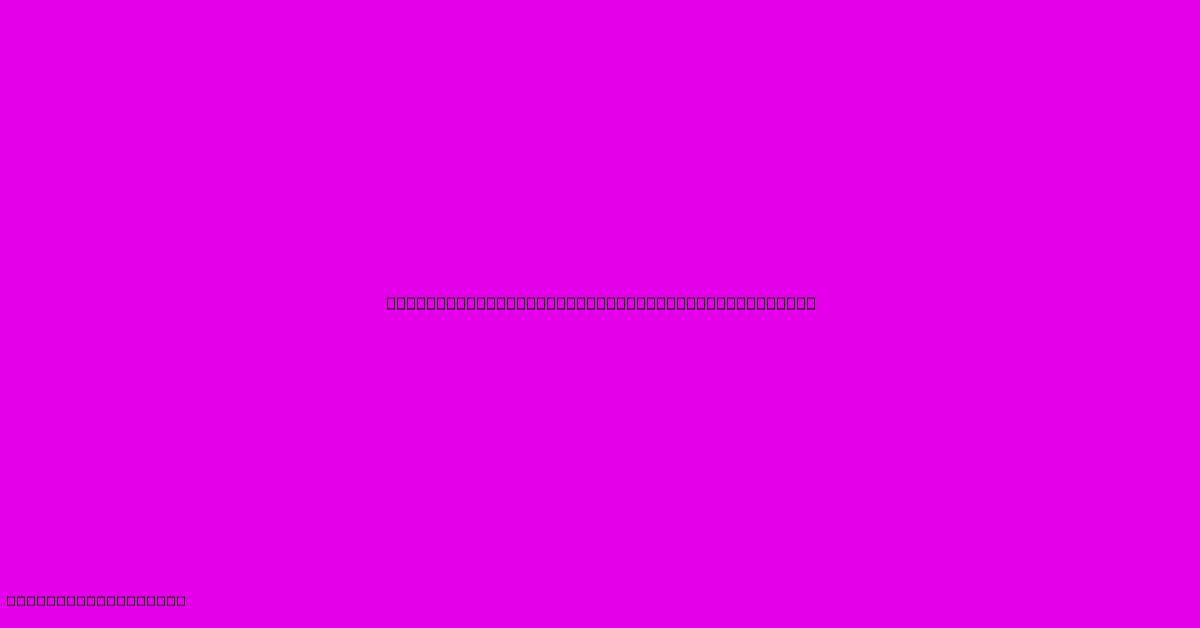
Thank you for taking the time to explore our website Nonaccrual Experience Nae Method Definition. We hope you find the information useful. Feel free to contact us for any questions, and don’t forget to bookmark us for future visits!
We truly appreciate your visit to explore more about Nonaccrual Experience Nae Method Definition. Let us know if you need further assistance. Be sure to bookmark this site and visit us again soon!
Featured Posts
-
Cash Commodity Definition
Jan 03, 2025
-
Catastrophe Excess Reinsurance Definition
Jan 03, 2025
-
What Credit Bureau Does Wayfair Pull From
Jan 03, 2025
-
How Often Does Stellantis Pay Dividends
Jan 03, 2025
-
Either Way Market Definition
Jan 03, 2025