One Tailed Test Explained Definition And Example
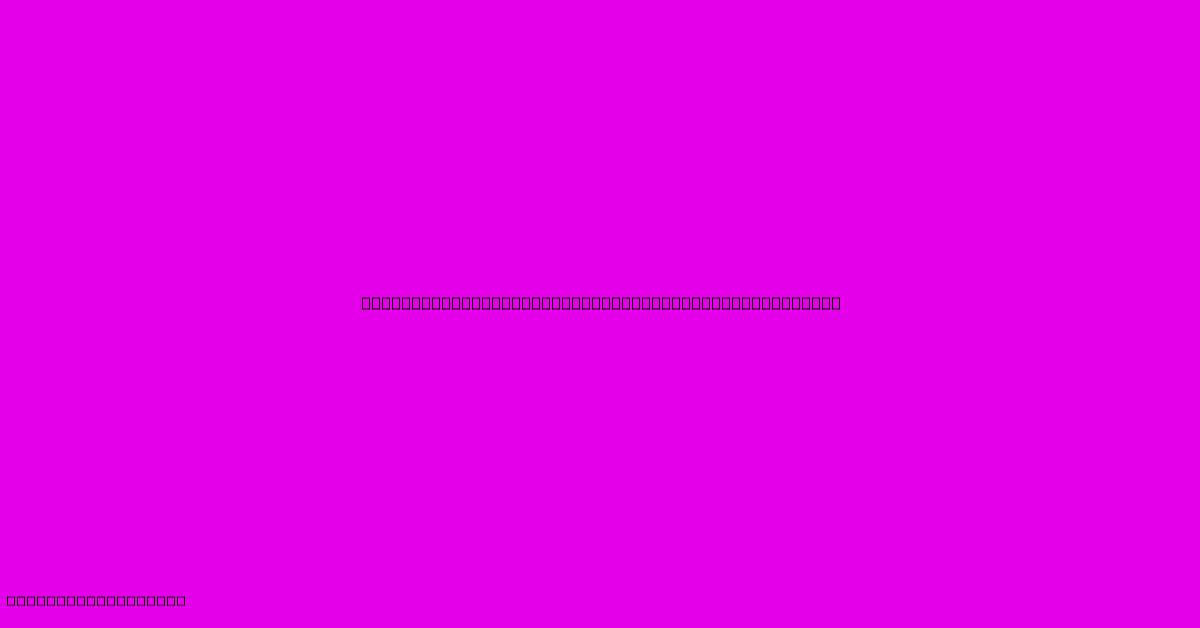
Discover more in-depth information on our site. Click the link below to dive deeper: Visit the Best Website meltwatermedia.ca. Make sure you don’t miss it!
Table of Contents
Unveiling the One-Tailed Test: A Comprehensive Guide
Hook: Does your hypothesis predict a directional change in a variable? If so, understanding the one-tailed test is crucial for accurate statistical analysis. This powerful tool allows researchers to focus their analysis on a specific direction of change, increasing the sensitivity of their findings.
Editor's Note: This comprehensive guide to one-tailed tests has been published today.
Relevance & Summary: Researchers across various fields rely on hypothesis testing to draw meaningful conclusions from data. This article provides a clear explanation of one-tailed tests, highlighting their application, advantages, and limitations. We will explore the definition, contrasting it with two-tailed tests, provide illustrative examples, and address common misconceptions. Understanding this statistical technique is essential for anyone involved in data analysis, from students to seasoned researchers. Keywords: one-tailed test, hypothesis testing, statistical significance, p-value, directional hypothesis, Type I error, Type II error.
Analysis: The following explanation incorporates established statistical principles and utilizes examples from various fields to illustrate the practical application of one-tailed tests. The content emphasizes clarity and accuracy, ensuring a comprehensive understanding of this critical statistical concept.
Key Takeaways:
- One-tailed tests assess directional hypotheses.
- They offer increased statistical power compared to two-tailed tests when the direction of the effect is known beforehand.
- Misapplication can lead to inaccurate conclusions.
- Proper understanding of p-values and critical regions is crucial.
One-Tailed Test: A Deep Dive
Subheading: One-Tailed Test
Introduction: A one-tailed test, also known as a directional hypothesis test, is a statistical test used to determine whether a population parameter is significantly greater than or less than a hypothesized value. Unlike a two-tailed test, which considers both positive and negative deviations from the hypothesized value, a one-tailed test focuses on a single direction of change. This specificity is crucial when the researcher has prior knowledge or a strong theoretical basis to predict the direction of the effect. This directional nature significantly impacts the interpretation of the results and the determination of statistical significance.
Key Aspects:
The core elements of a one-tailed test include:
- Directional Hypothesis: The researcher formulates a hypothesis that specifies the direction of the difference (greater than or less than).
- Critical Region: Only one tail of the sampling distribution contains the region of rejection (critical region).
- P-value: The probability of observing the obtained results (or more extreme results) if the null hypothesis is true. For a one-tailed test, the p-value is calculated in only one tail of the distribution.
Discussion: The choice between a one-tailed and a two-tailed test hinges on the nature of the research question. If a researcher has strong reason to believe that the effect will be in a specific direction, a one-tailed test is appropriate. For instance, a study investigating the effect of a new drug on reducing blood pressure would likely use a one-tailed test because it's anticipated that the drug will lower blood pressure, not raise it. However, if the direction of the effect is uncertain, a two-tailed test is more suitable. Misapplication of a one-tailed test when a two-tailed test is appropriate can lead to incorrect conclusions.
One-Tailed Test vs. Two-Tailed Test
Subheading: The Crucial Distinction: One-Tailed vs. Two-Tailed Tests
Introduction: Understanding the fundamental difference between one-tailed and two-tailed tests is paramount for appropriate statistical analysis. The choice directly impacts the interpretation of results and the probability of committing Type I and Type II errors.
Facets:
Feature | One-Tailed Test | Two-Tailed Test |
---|---|---|
Hypothesis | Directional (e.g., greater than, less than) | Non-directional (e.g., different from) |
Critical Region | One tail of the distribution | Both tails of the distribution |
P-value | Calculated in one tail | Calculated in both tails |
Statistical Power | Higher (when the direction is correctly predicted) | Lower |
Type I Error Risk | Lower (if the direction is correctly specified) | Higher |
Type II Error Risk | Higher (if the direction is incorrectly specified) | Lower |
Examples | Testing if a new drug reduces blood pressure | Testing if a new drug changes blood pressure |
Summary: The choice between a one-tailed and two-tailed test should be made before data collection, based on the research hypothesis. Using a one-tailed test when a two-tailed test is appropriate increases the risk of a Type II error (failing to reject a false null hypothesis). Conversely, if the direction is known a priori, a one-tailed test offers increased power to detect a true effect.
Applications and Examples of One-Tailed Tests
Subheading: Real-World Applications of One-Tailed Tests
Introduction: One-tailed tests find application in diverse fields where a directional hypothesis is justified. This section provides several practical examples to illustrate the concept.
Further Analysis:
-
Pharmaceutical Research: Clinical trials testing the efficacy of a new drug often employ one-tailed tests. If the drug is expected to improve a specific condition (e.g., lower cholesterol), a one-tailed test focusing on improvement is appropriate.
-
Educational Research: Evaluating the effectiveness of a new teaching method, where researchers expect improved student performance, could utilize a one-tailed test.
-
Economic Studies: Analyzing the impact of a government policy on unemployment, where a decrease in unemployment is anticipated, might necessitate a one-tailed test.
-
Environmental Science: Investigating if pollution levels have decreased following a cleanup effort would be a suitable application for a one-tailed test.
Closing: The decision to use a one-tailed test should be driven by a strong theoretical basis or prior knowledge supporting the directional hypothesis. Failure to meet these conditions risks invalidating the results and potentially leading to erroneous conclusions.
FAQ: Addressing Common Questions about One-Tailed Tests
Subheading: FAQ
Introduction: This section addresses frequently asked questions regarding one-tailed tests, clarifying potential misconceptions.
Questions:
-
Q: When should I use a one-tailed test? A: Use a one-tailed test when your hypothesis specifies the direction of the effect and you have strong theoretical or empirical reasons to support this direction.
-
Q: What are the potential drawbacks of using a one-tailed test? A: If the effect is in the opposite direction of your hypothesis, you may fail to detect a statistically significant result even if a true effect exists.
-
Q: How do I interpret the p-value in a one-tailed test? A: The p-value represents the probability of observing the obtained results (or more extreme results in the specified direction) if the null hypothesis is true.
-
Q: Can I switch to a one-tailed test after looking at the data? A: No, this is considered data dredging and invalidates the statistical analysis. The decision to use a one-tailed test must be made before analyzing the data.
-
Q: What is the relationship between a one-tailed test and Type I error? A: A one-tailed test can reduce the risk of a Type I error (rejecting a true null hypothesis) if the hypothesized direction is correct. However, if the direction is incorrect, the risk of a Type II error increases.
-
Q: How does sample size affect the results of a one-tailed test? A: A larger sample size generally increases the power of the test, making it more likely to detect a true effect.
Summary: Choosing between one-tailed and two-tailed tests requires careful consideration of the research question and the potential implications of Type I and Type II errors. Understanding these nuances is crucial for conducting robust statistical analyses.
Transition: Let’s now explore some practical tips for effectively utilizing one-tailed tests.
Tips for Effective Use of One-Tailed Tests
Subheading: Tips for Effective Use of One-Tailed Tests
Introduction: Implementing one-tailed tests effectively requires careful planning and a clear understanding of their limitations. This section provides valuable guidance.
Tips:
-
Clearly Define Your Hypothesis: Before collecting data, formulate a precise directional hypothesis, specifying the expected direction of the effect.
-
Justify Your Choice: Document the reasons for choosing a one-tailed test. This justification should be based on prior research, theoretical models, or strong a priori assumptions.
-
Select the Appropriate Statistical Test: Choose the appropriate statistical test for your data (e.g., one-sample t-test, one-tailed z-test, etc.).
-
Interpret Results Carefully: The p-value only reflects the probability of observing the data given the null hypothesis is true in the specified direction.
-
Report Transparency: Clearly report your chosen test and the rationale behind your decision in your research paper or report.
-
Consider Power Analysis: Before conducting the study, perform a power analysis to determine the necessary sample size to detect a meaningful effect with sufficient power.
Summary: By following these tips, researchers can ensure the appropriate and effective application of one-tailed tests, leading to more robust and meaningful conclusions.
Transition: This article has explored the intricacies of one-tailed tests, providing a comprehensive guide for researchers and data analysts.
Summary of One-Tailed Tests
Summary: This article provided a detailed explanation of one-tailed tests, including their definition, application, advantages, and limitations. The guide contrasts them with two-tailed tests, highlighting the crucial decision-making process involved in selecting the appropriate test. Real-world examples across various disciplines illustrated the practical relevance of one-tailed tests. Furthermore, frequently asked questions and valuable tips for effective use were addressed to facilitate accurate and responsible statistical analysis.
Closing Message: Understanding one-tailed tests is crucial for researchers aiming to draw accurate and meaningful conclusions from their data. The ability to choose the appropriate statistical test based on the research question and prior knowledge is a fundamental skill in the scientific method. By carefully considering the implications and limitations of this statistical tool, researchers can enhance the rigor and reliability of their findings.
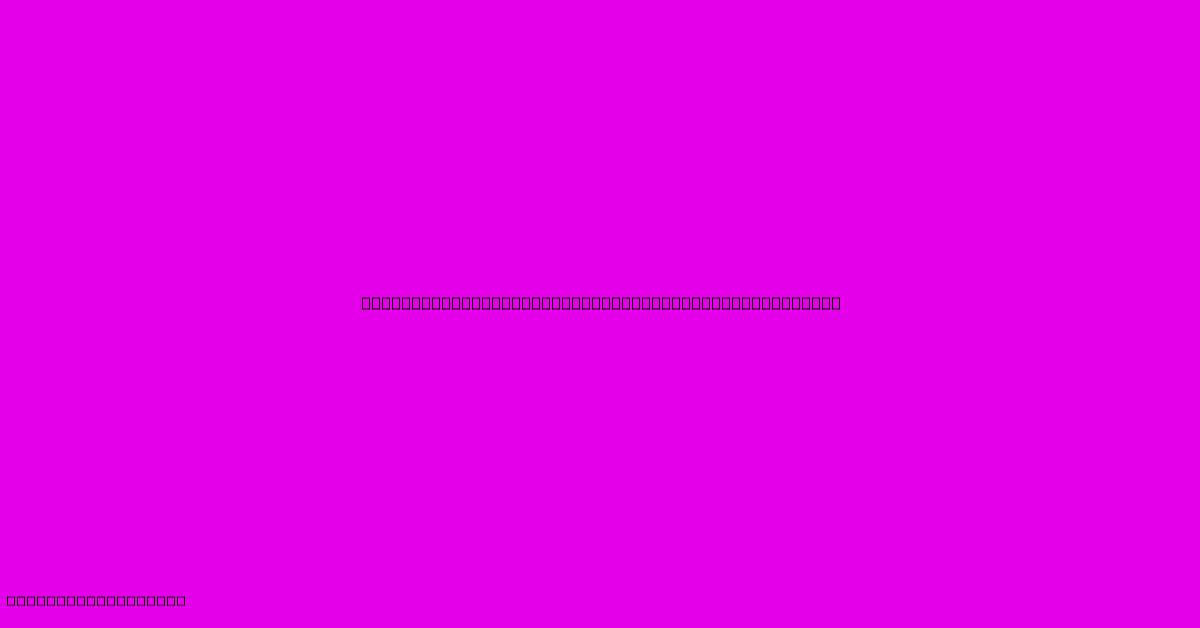
Thank you for taking the time to explore our website One Tailed Test Explained Definition And Example. We hope you find the information useful. Feel free to contact us for any questions, and don’t forget to bookmark us for future visits!
We truly appreciate your visit to explore more about One Tailed Test Explained Definition And Example. Let us know if you need further assistance. Be sure to bookmark this site and visit us again soon!
Featured Posts
-
What Gas Stations Take Credit
Jan 03, 2025
-
How To Book Hotel Without Credit Card
Jan 03, 2025
-
Open Banking Definition How It Works And Risks
Jan 03, 2025
-
How To Stop Getting Credit Card Applications In The Mail
Jan 03, 2025
-
Chaikin Oscillator Definition Calculation Formula Example
Jan 03, 2025