Positive Correlation Definition Measurement Examples
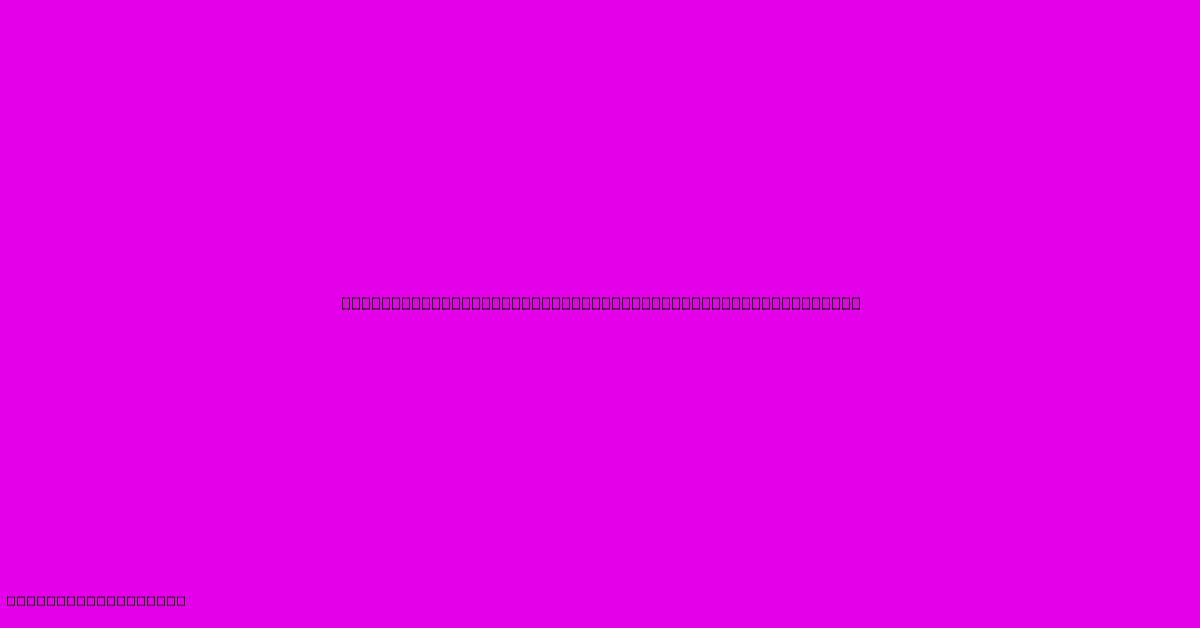
Discover more in-depth information on our site. Click the link below to dive deeper: Visit the Best Website meltwatermedia.ca. Make sure you don’t miss it!
Table of Contents
Unveiling Positive Correlation: Definition, Measurement, and Examples
Hook: Does the rise of ice cream sales mysteriously coincide with an increase in crime rates? While seemingly unrelated, this illustrates the concept of correlation – a statistical relationship between two variables. Understanding positive correlation, in particular, is crucial for interpreting data and making informed decisions across numerous fields.
Editor's Note: This comprehensive guide to positive correlation has been published today.
Relevance & Summary: Positive correlation is a fundamental statistical concept with wide-ranging applications in various disciplines. This article explores its definition, different measurement methods (including Pearson's r, Spearman's rho, and Kendall's tau), and provides illustrative examples across diverse fields like economics, healthcare, and environmental science. Understanding positive correlation empowers better decision-making by revealing patterns and predicting trends within data sets. The guide covers key aspects like correlation coefficients, scatter plots, and the crucial distinction between correlation and causation.
Analysis: This article draws on established statistical principles and utilizes numerous real-world examples to explain the concept of positive correlation. Data visualization techniques, such as scatter plots, are used to illustrate the relationship between variables, and the strengths and limitations of various correlation coefficients are discussed.
Key Takeaways:
- Positive correlation indicates that two variables tend to move in the same direction.
- Correlation coefficients (e.g., Pearson's r) quantify the strength and direction of the relationship.
- Scatter plots visually represent the relationship between variables.
- Correlation does not imply causation.
Positive Correlation: A Deep Dive
Subheading: Positive Correlation
Introduction: Positive correlation describes a statistical relationship where an increase in one variable is associated with an increase in another variable, and a decrease in one variable is associated with a decrease in the other. It’s crucial to understand that this association doesn't necessarily imply a cause-and-effect relationship. The strength of this relationship is measured using correlation coefficients, with values ranging from 0 to +1. A value of +1 represents a perfect positive correlation, while 0 indicates no linear correlation.
Key Aspects:
- Direction: Positive correlation indicates a direct relationship.
- Strength: The strength of the correlation is represented by the magnitude of the correlation coefficient.
- Linearity: Positive correlation primarily focuses on linear relationships, although non-linear positive relationships can also exist.
Discussion:
Imagine tracking the hours spent studying and the resulting exam scores for a group of students. A positive correlation would be observed if, generally, students who studied more hours tended to achieve higher scores. Similarly, consider the relationship between exercise and weight loss. Increased physical activity frequently correlates with weight reduction. These examples showcase the positive relationship between two variables, indicating a tendency for them to move together. However, it's crucial to note that other factors may also influence both variables. For instance, a student's inherent aptitude or prior knowledge could also affect their exam performance, while diet and genetics play a role in weight management besides exercise. This highlights the crucial distinction between correlation and causation.
Measuring Positive Correlation
Subheading: Correlation Coefficients
Introduction: Several methods exist for quantifying the strength and direction of a positive correlation. The most common is Pearson's correlation coefficient (r), but Spearman's rho and Kendall's tau are also used depending on the nature of the data.
Facets:
- Pearson's r: This measures the linear association between two continuous variables. Values range from -1 to +1. A value close to +1 indicates a strong positive linear correlation.
- Spearman's rho: This is a non-parametric measure of correlation that assesses the monotonic relationship between two variables. It's suitable when the data is ordinal or when the relationship isn't strictly linear. Values also range from -1 to +1.
- Kendall's tau: Another non-parametric measure that assesses the ordinal association between two variables. It's less sensitive to outliers than Spearman's rho. Values range from -1 to +1.
Summary: The choice of correlation coefficient depends on the characteristics of the data and the type of relationship being investigated. Pearson's r is best suited for linear relationships between continuous variables, while Spearman's rho and Kendall's tau are preferred for non-linear or ordinal data.
Examples of Positive Correlation
Subheading: Real-World Applications
Introduction: Positive correlation manifests in numerous real-world scenarios across various disciplines.
Further Analysis:
- Economics: The relationship between consumer spending and economic growth often exhibits a positive correlation. As the economy expands, consumer confidence and spending tend to increase.
- Healthcare: Studies have shown a positive correlation between regular exercise and cardiovascular health. Individuals who exercise regularly generally have lower risks of heart disease.
- Environmental Science: There's often a positive correlation between greenhouse gas emissions and global temperatures. Increased emissions are associated with rising global average temperatures.
- Education: A positive correlation is frequently observed between the number of books read and vocabulary size. Increased reading is often associated with a larger vocabulary.
- Marketing: A company might observe a positive correlation between its marketing spend and brand awareness. Increased marketing efforts might result in a higher level of brand recognition.
Closing: Understanding positive correlation enables more accurate interpretations of data across numerous fields. It allows for the identification of trends and patterns, enabling better forecasting and strategic decision-making. However, always remember the crucial distinction: correlation does not equal causation. Further investigation is essential to determine if a causal relationship exists between correlated variables.
FAQ: Positive Correlation
Introduction: This section addresses common queries regarding positive correlation.
Questions:
-
Q: What's the difference between positive correlation and causation? A: Positive correlation simply indicates that two variables tend to move together. Causation implies that one variable directly causes a change in the other. Correlation does not prove causation.
-
Q: Can a positive correlation be non-linear? A: While Pearson's r focuses on linear relationships, positive correlations can exist in non-linear forms. Spearman's rho and Kendall's tau are better suited for assessing these.
-
Q: How is a positive correlation visualized? A: Scatter plots are commonly used to visualize positive correlations. Points on the plot will generally trend upwards from left to right.
-
Q: What is the range of a Pearson's correlation coefficient? A: The range is from -1 to +1. +1 indicates a perfect positive linear correlation, 0 indicates no linear correlation, and -1 indicates a perfect negative linear correlation.
-
Q: Are there any limitations to using correlation coefficients? A: Yes, correlation coefficients can be affected by outliers and may not accurately reflect non-linear relationships.
-
Q: Can a strong positive correlation be misleading? A: Yes, a strong positive correlation doesn't necessarily imply a causal relationship. Other confounding variables could be at play.
Summary: Understanding the nuances of positive correlation requires careful consideration of the data and the appropriate statistical methods.
Tips for Understanding Positive Correlation
Introduction: These tips help enhance the understanding and application of positive correlation.
Tips:
- Visualize your data: Use scatter plots to visualize the relationship between variables.
- Choose the appropriate correlation coefficient: Select Pearson's r for linear relationships between continuous variables, and Spearman's rho or Kendall's tau for non-linear or ordinal data.
- Consider potential confounding variables: Don't assume causation based solely on correlation. Investigate other factors that might influence the observed relationship.
- Beware of outliers: Outliers can significantly impact correlation coefficients. Consider removing or addressing outliers before interpreting results.
- Context is key: Interpret correlation coefficients within the context of the research question and the specific variables being studied.
- Replicate your findings: If possible, replicate your analysis using different data sets to validate your conclusions.
- Consult statistical experts: If you're unsure about the best approach, seek guidance from a statistician or data analyst.
Summary: By applying these tips, researchers can more effectively interpret data and understand the true nature of positive correlations.
Summary: Positive Correlation
Summary: This article provided a comprehensive overview of positive correlation, including its definition, measurement methods, and real-world examples. The importance of differentiating between correlation and causation was emphasized.
Closing Message: Understanding positive correlation is a fundamental skill for anyone working with data. By carefully applying the appropriate statistical methods and considering potential confounding variables, one can derive valuable insights and make more informed decisions. Further research into specific areas and applications of positive correlation will continue to refine our understanding of relationships within data sets.
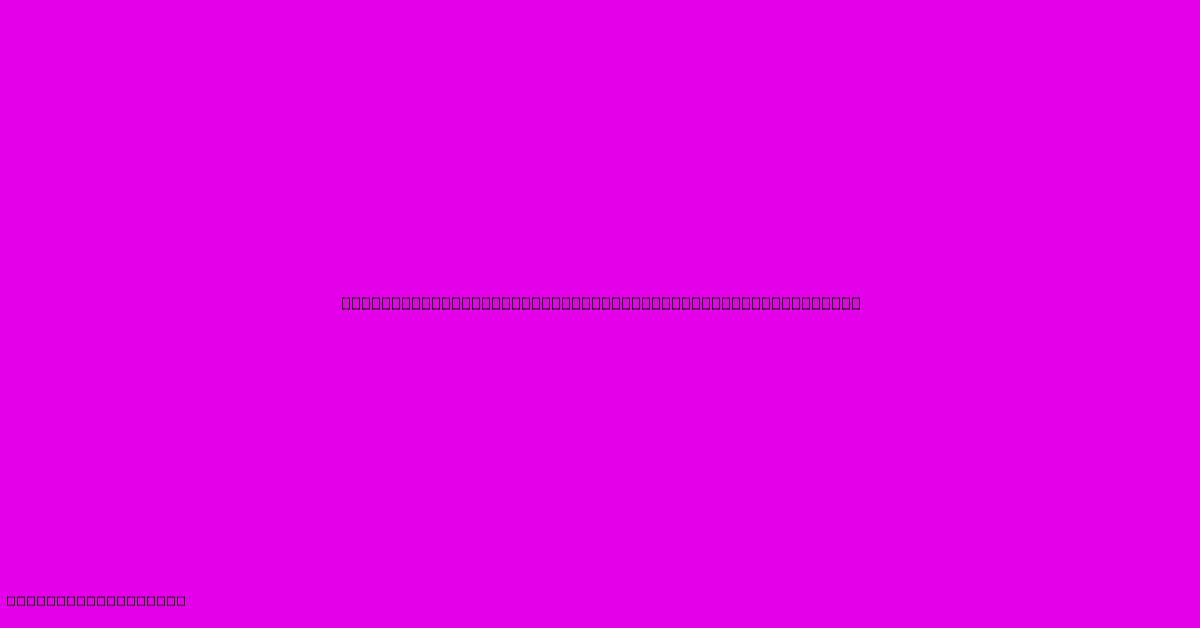
Thank you for taking the time to explore our website Positive Correlation Definition Measurement Examples. We hope you find the information useful. Feel free to contact us for any questions, and don’t forget to bookmark us for future visits!
We truly appreciate your visit to explore more about Positive Correlation Definition Measurement Examples. Let us know if you need further assistance. Be sure to bookmark this site and visit us again soon!
Featured Posts
-
How To Get Homeowners Insurance After Being Dropped
Jan 03, 2025
-
Over Selling Definition
Jan 03, 2025
-
Predator Definition
Jan 03, 2025
-
Cashiers Check Definition Benefits And Alternative Options
Jan 03, 2025
-
What Does Credit Analyst Do
Jan 03, 2025