Queuing Theory Definition Elements And Example
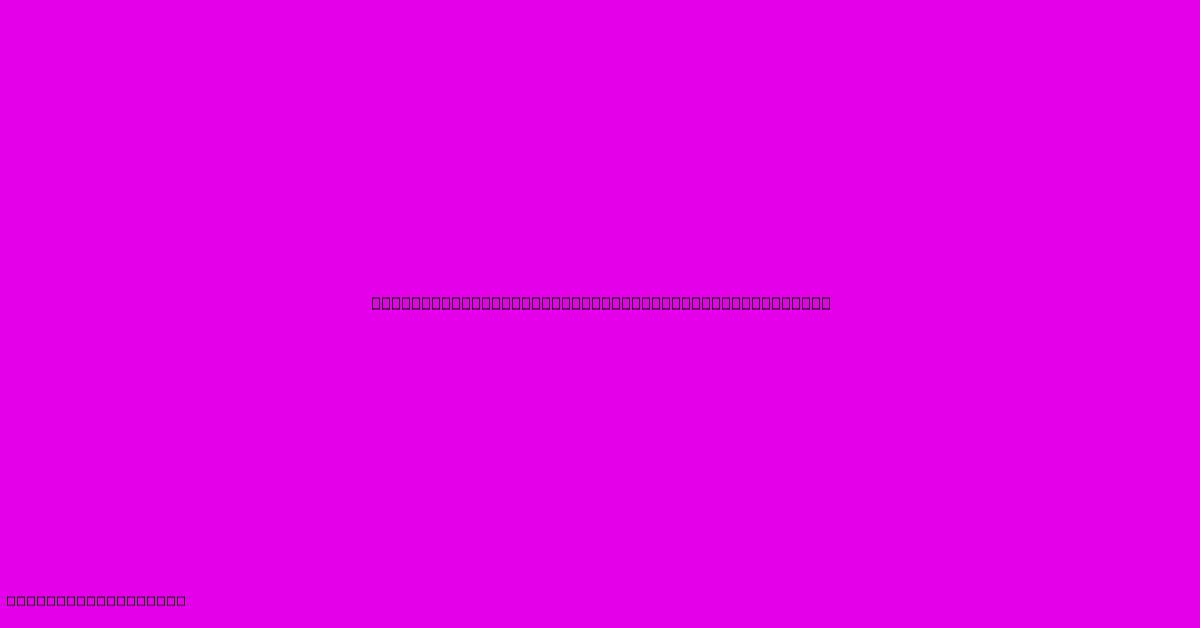
Discover more in-depth information on our site. Click the link below to dive deeper: Visit the Best Website meltwatermedia.ca. Make sure you don’t miss it!
Table of Contents
Unveiling the Secrets of Queuing Theory: Definitions, Elements, and Real-World Applications
Hook: Have you ever waited in a long line at the grocery store, airport security, or a popular theme park ride? That frustrating experience is precisely what queuing theory helps us understand and optimize. This powerful analytical tool allows us to predict and improve the efficiency of systems involving waiting lines.
Editor's Note: This comprehensive guide to queuing theory has been published today.
Relevance & Summary: Queuing theory, also known as waiting-line theory, is crucial for businesses and organizations seeking to improve operational efficiency and customer satisfaction. This article will explore the fundamental definitions, key elements, and real-world examples of queuing theory, providing insights into its applications across various industries. Keywords: queuing theory, waiting-line theory, queue management, Little's Law, queuing models, service systems, waiting time, customer satisfaction, operational efficiency.
Analysis: This guide synthesizes information from leading academic texts on operations research, statistical modeling, and management science. It leverages real-world case studies and examples to illustrate the practical applications of queuing theory concepts.
Key Takeaways:
- Understanding queuing theory enables informed decision-making in service system design.
- Key elements like arrival rate, service rate, and queue discipline significantly impact system performance.
- Various queuing models cater to different scenarios and complexities.
- Optimizing queue management can significantly reduce waiting times and improve customer satisfaction.
Queuing Theory: A Deep Dive
Introduction
Queuing theory provides a mathematical framework for analyzing and modeling systems where customers or entities arrive for service and potentially experience waiting time before receiving that service. Its significance stems from its ability to predict key performance indicators (KPIs) such as average waiting time, queue length, and server utilization, enabling proactive optimization strategies. Understanding these metrics allows businesses to make informed decisions about resource allocation, staffing levels, and system design.
Key Aspects of Queuing Theory
Queuing theory rests on several fundamental aspects that define the characteristics of a waiting-line system. These aspects are often represented using Kendall's notation, a standardized system for classifying queuing models.
1. Arrival Process: This describes how customers arrive at the service system. It's frequently modeled using Poisson processes, characterized by a constant arrival rate (λ) representing the average number of arrivals per unit time. Other arrival patterns, such as deterministic (arrivals at fixed intervals) or general distributions, can also be considered depending on the system's nature.
2. Service Process: This defines how long it takes to serve a customer. Similar to the arrival process, it is often modeled using an exponential distribution, reflecting a constant service rate (μ) representing the average number of customers served per unit time. Other distributions, like normal or uniform, might be more appropriate depending on the service characteristics.
3. Number of Servers: This refers to the number of service channels available to handle customer requests. A single-server system has one server, while multi-server systems have multiple servers working concurrently.
4. Queue Capacity: This indicates the maximum number of customers that the queue can hold. Some systems have infinite capacity, meaning the queue can grow indefinitely, while others have finite capacity, limiting the number of customers allowed to wait.
5. Queue Discipline: This specifies the order in which customers are served. Common disciplines include First-In, First-Out (FIFO), Last-In, First-Out (LIFO), and priority-based queuing, where customers with higher priority are served before others.
Exploring Key Elements: Arrival Rates and Service Rates
Arrival Rates (λ)
The arrival rate (λ) represents the average number of customers arriving at the system per unit of time. Understanding this rate is crucial for predicting queue length and waiting times. Variations in arrival rate, such as peak periods versus off-peak periods, significantly influence system performance. For instance, a restaurant experiences higher arrival rates during lunch and dinner hours compared to quieter times of the day.
Facets:
- Role: Predicts customer influx.
- Examples: Customers entering a bank, cars arriving at a toll booth, patients arriving at a hospital.
- Risks and Mitigations: Unpredictable surges in arrival rates can lead to long queues. Mitigations include appointment scheduling, flexible staffing, and surge capacity planning.
- Impacts and Implications: Higher arrival rates generally lead to longer waiting times and increased queue lengths.
Service Rates (μ)
The service rate (μ) represents the average number of customers served per unit time. It’s determined by factors like server efficiency, service complexity, and available resources. Efficient service processes contribute to shorter waiting times and reduced congestion. A fast-food restaurant with highly trained staff and streamlined processes typically exhibits higher service rates than one with inefficient operations.
Facets:
- Role: Determines how quickly the system processes requests.
- Examples: Time taken to process a transaction, complete a task, or provide a service.
- Risks and Mitigations: Low service rates lead to longer waiting times. Mitigations include process optimization, employee training, and improved equipment.
- Impacts and Implications: Higher service rates generally result in shorter waiting times and improved customer satisfaction.
Little's Law: A Fundamental Relationship
Little's Law is a fundamental theorem in queuing theory that establishes a simple but powerful relationship between average queue length (L), average arrival rate (λ), and average time spent in the system (W):
L = λW
This law holds true for a wide range of queuing systems, regardless of their specific characteristics. It provides a valuable tool for estimating queue length or average waiting time when one of the variables is known. For example, if a supermarket knows its average arrival rate and the average time customers spend shopping, Little's Law can be used to estimate the average number of customers in the store at any given time.
Queuing Models: A Diverse Landscape
Various queuing models exist to address diverse system characteristics. The choice of model depends on the specific details of the system being analyzed. Some common models include:
- M/M/1: This model assumes Poisson arrivals (M), exponential service times (M), and a single server (1).
- M/M/c: This model is similar to M/M/1 but allows for multiple servers (c).
- M/G/1: This model retains Poisson arrivals (M) and a single server (1) but allows for general service time distributions (G).
Real-World Applications of Queuing Theory
Queuing theory finds practical applications in numerous fields:
- Healthcare: Optimizing patient flow in hospitals and clinics to reduce waiting times and improve resource allocation.
- Manufacturing: Managing production lines and inventory to minimize bottlenecks and maximize throughput.
- Telecommunications: Designing efficient network systems to handle call traffic and minimize dropped calls.
- Transportation: Optimizing traffic flow, airport operations, and public transportation systems.
- Customer Service: Designing call centers and online help desks to minimize customer waiting times and improve service quality.
FAQ
Introduction
This section addresses frequently asked questions about queuing theory.
Questions
Q1: What are the limitations of queuing theory? A1: Queuing models often make simplifying assumptions (e.g., Poisson arrivals, exponential service times) that may not always perfectly reflect real-world scenarios. Furthermore, the accuracy of predictions depends on the quality of input data.
Q2: How can I apply queuing theory to my business? A2: Identify your system's arrival and service processes, estimate the relevant parameters (λ and μ), choose an appropriate queuing model, and use it to analyze key performance indicators.
Q3: What software tools are available for queuing analysis? A3: Several software packages, including simulation software and specialized queuing analysis tools, can assist with complex queuing problems.
Q4: Can queuing theory predict human behavior? A4: While queuing theory focuses on system behavior, human behavior can influence arrival rates and service times, adding complexity. Incorporating behavioral factors into the model can improve accuracy.
Q5: What are the ethical implications of using queuing theory? A5: Ensuring fairness in queuing systems is crucial. Prioritization schemes should be transparent and justifiable.
Q6: How does queuing theory relate to other operations research techniques? A6: It is closely linked to other OR methods like simulation, linear programming, and inventory management, often used in conjunction to solve complex operational problems.
Summary
Understanding and addressing common questions about queuing theory clarifies its practical implementation and limitations.
Tips for Effective Queue Management
Introduction
This section offers practical tips for improving queue management in various settings.
Tips
- Implement appointment scheduling: Reduces random arrivals and improves predictability.
- Optimize service processes: Streamline operations to reduce service times and improve efficiency.
- Use technology: Employ queuing systems and digital tools for managing and tracking queues.
- Provide clear signage and instructions: Minimizes confusion and improves customer flow.
- Train staff effectively: Well-trained staff can handle customers quickly and efficiently.
- Offer multiple service channels: Reduces wait times by providing multiple options for service.
- Monitor and analyze queue performance: Track key metrics to identify areas for improvement.
- Communicate wait times proactively: Keep customers informed about expected waiting times.
Summary
Implementing these tips contributes to improved queue management, leading to enhanced customer satisfaction and operational efficiency.
Conclusion
This exploration of queuing theory has highlighted its significance as a powerful tool for understanding and optimizing service systems. From its fundamental definitions and key elements to its diverse applications and practical implications, the analysis demonstrates its importance across various sectors. By understanding and applying the principles of queuing theory, organizations can significantly enhance their operational efficiency, improve customer satisfaction, and make more informed resource allocation decisions, ultimately leading to greater success. Future research should explore the integration of queuing theory with emerging technologies like artificial intelligence and machine learning to further enhance its capabilities and applicability.
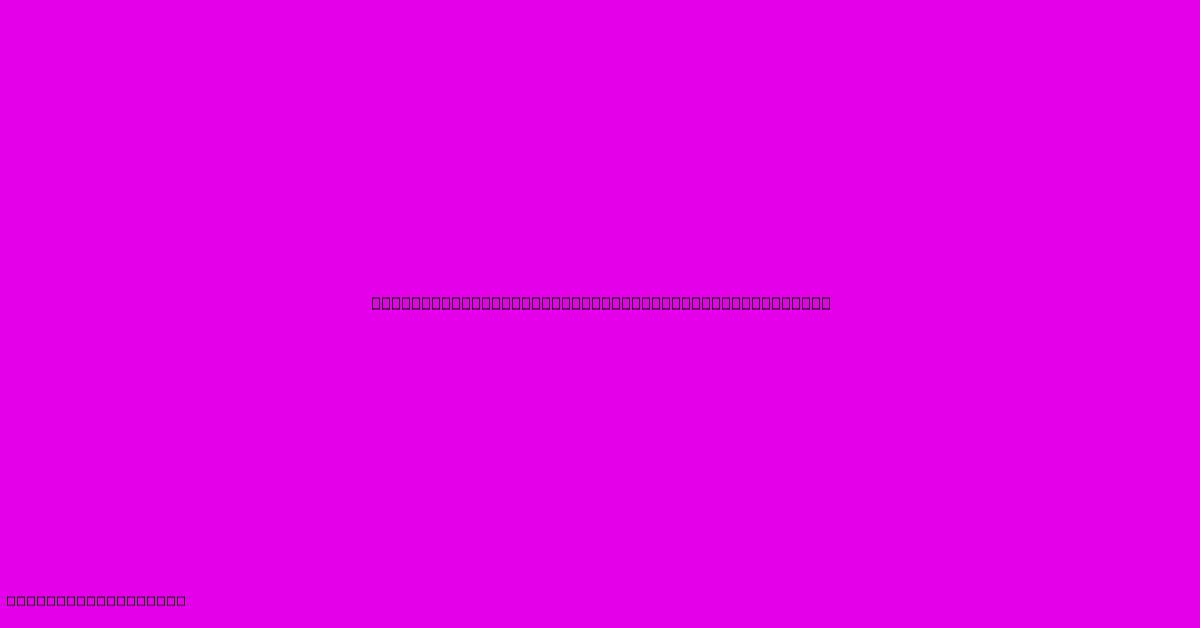
Thank you for taking the time to explore our website Queuing Theory Definition Elements And Example. We hope you find the information useful. Feel free to contact us for any questions, and don’t forget to bookmark us for future visits!
We truly appreciate your visit to explore more about Queuing Theory Definition Elements And Example. Let us know if you need further assistance. Be sure to bookmark this site and visit us again soon!
Featured Posts
-
What Does Ent Credit Union Stand For
Jan 12, 2025
-
How Often Does Macys Increase Credit Limit
Jan 12, 2025
-
Qualified Reservist Definition
Jan 12, 2025
-
Quick Assets Definition
Jan 12, 2025
-
What Can You Use Carnival Onboard Credit For
Jan 12, 2025