Random Variable Definition Types How Its Used And Example
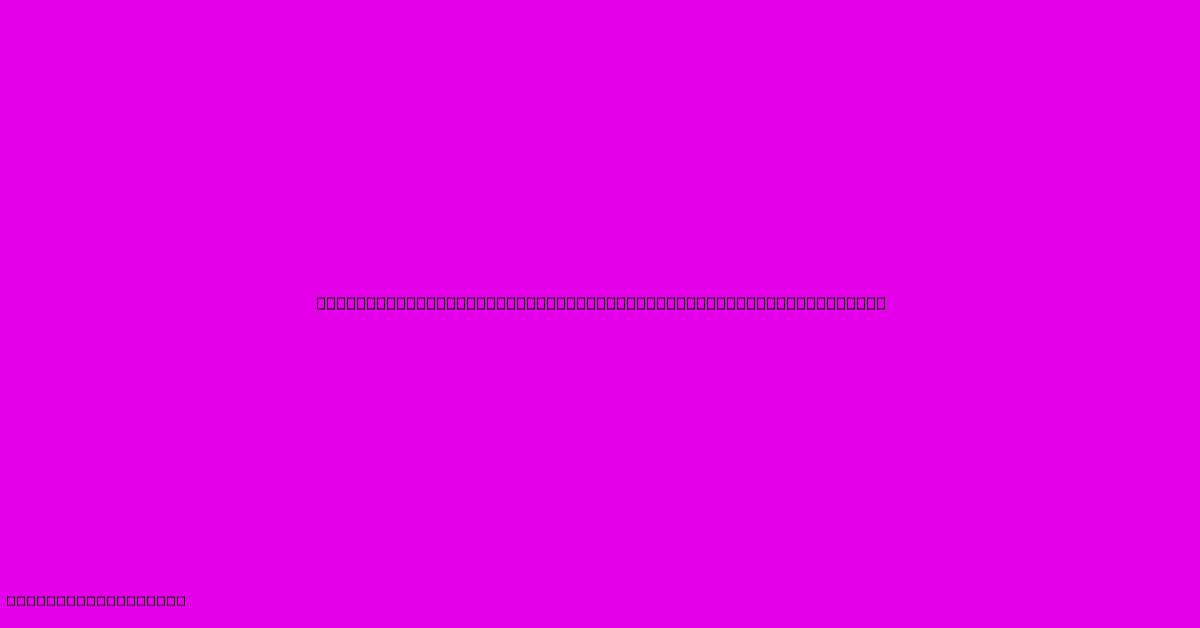
Discover more in-depth information on our site. Click the link below to dive deeper: Visit the Best Website meltwatermedia.ca. Make sure you don’t miss it!
Table of Contents
Unveiling Random Variables: Definition, Types, Usage, and Examples
Hook: What if you could predict the outcome of any uncertain event? While perfectly predicting the future remains elusive, understanding random variables provides a powerful framework for modeling and analyzing uncertainty. This exploration delves into the core concepts of random variables, their types, applications, and practical examples.
Editor's Note: This comprehensive guide to random variables has been published today.
Relevance & Summary: Understanding random variables is crucial for anyone working with data analysis, statistics, probability, and machine learning. This guide provides a clear definition, explores various types of random variables (discrete, continuous, and mixed), showcases their practical applications, and clarifies their use with illustrative examples. It covers key concepts like probability distributions, expected value, and variance, ultimately enabling readers to better interpret and utilize data involving uncertainty. Semantic keywords include: probability distribution, discrete random variable, continuous random variable, expected value, variance, statistical analysis, data modeling, probability theory, random process.
Analysis: This guide is based on established principles of probability theory and statistical analysis. The examples presented are drawn from diverse fields to demonstrate the widespread applicability of random variables. The information presented aims for clarity and accuracy, ensuring a comprehensive understanding of the topic.
Key Takeaways:
- Definition and significance of random variables.
- Categorization into discrete, continuous, and mixed types.
- Practical applications across diverse fields.
- Calculation and interpretation of expected value and variance.
Random Variables: A Foundation of Uncertainty Modeling
Random variables are fundamental in probability theory and statistics. They provide a mathematical representation of the outcomes of random phenomena. Essentially, a random variable is a variable whose value is a numerical outcome of a random phenomenon. Its importance stems from its ability to quantify uncertainty, allowing for mathematical analysis and prediction of events with probabilistic outcomes.
Key Aspects of Random Variables
Random variables are characterized by several key aspects:
- Sample Space: The set of all possible outcomes of a random experiment.
- Probability Distribution: A function describing the probability of each possible value of the random variable. This can be expressed as a probability mass function (PMF) for discrete variables or a probability density function (PDF) for continuous variables.
- Expected Value (E[X]): The average value of the random variable, weighted by its probability distribution. It represents the long-run average outcome.
- Variance (Var(X)): A measure of the spread or dispersion of the random variable's values around its expected value. A higher variance indicates greater uncertainty.
Types of Random Variables
Random variables are broadly classified into three categories:
-
Discrete Random Variables: These variables can only take on a finite number of values or a countably infinite number of values. Examples include the number of heads in three coin tosses (values: 0, 1, 2, 3), or the number of cars passing a certain point on a highway in an hour. Their probability distribution is described by a probability mass function (PMF).
-
Continuous Random Variables: These variables can take on any value within a given range or interval. Examples include the height of a person, the temperature of a room, or the time it takes to complete a task. Their probability distribution is described by a probability density function (PDF).
-
Mixed Random Variables: These variables exhibit characteristics of both discrete and continuous random variables. They have a probability distribution that is a combination of a PMF and a PDF. A classic example could be the lifetime of a lightbulb, where there's a probability of immediate failure (discrete) and a probability of failure within a continuous time interval.
Point: Discrete Random Variables
Introduction: Discrete random variables are fundamental to understanding probability and statistics, providing a framework for analyzing events with a finite or countably infinite number of outcomes.
Facets:
- Roles: Modeling events with distinct outcomes, such as coin flips, die rolls, or counting occurrences (number of defects in a batch).
- Examples: Number of successes in a fixed number of Bernoulli trials, number of customers arriving at a store in an hour.
- Risks & Mitigations: Incorrectly assigning probabilities can lead to flawed conclusions; careful data collection and probability assessment are crucial.
- Impacts & Implications: Used in various applications including quality control, risk assessment, and modeling queuing systems.
Summary: Discrete random variables are essential tools for quantifying and analyzing discrete probabilistic events, having widespread application in various fields.
Point: Continuous Random Variables
Introduction: Continuous random variables are crucial for modeling phenomena with continuous outcomes, ranging from physical measurements to financial models. Their analysis involves probability density functions rather than probability mass functions.
Further Analysis: The normal distribution, a ubiquitous continuous distribution, is often used to model many natural phenomena, including human height and weight. Other examples include the exponential distribution (modeling time until an event occurs), and the uniform distribution (modeling equally likely outcomes within a range).
Closing: Continuous random variables provide the mathematical framework for understanding and predicting phenomena with a continuous range of outcomes. Their applications are vast and crucial in many fields.
Point: Applications of Random Variables
Introduction: The practical applications of random variables span a wide range of disciplines, from finance and engineering to medicine and social sciences. Their ability to model uncertainty makes them invaluable tools in decision-making and prediction.
Further Analysis: In finance, random variables model asset returns, allowing for risk assessment and portfolio optimization. In engineering, they model system reliability, helping to design robust systems. In healthcare, they are used to model disease spread and treatment effectiveness. In social sciences, they can model voting patterns or public opinion.
Closing: The versatility of random variables underscores their fundamental role in quantifying and analyzing uncertainty across a vast spectrum of real-world problems.
FAQ
Introduction: This section addresses frequently asked questions about random variables.
Questions:
- Q: What is the difference between a random variable and a random process? A: A random variable is a single numerical outcome of a random phenomenon, while a random process is a sequence or collection of random variables over time or some other index.
- Q: How does one choose the appropriate type of random variable for a given problem? A: The choice depends on the nature of the outcome. If the outcome is discrete (countable), a discrete random variable is appropriate. If the outcome is continuous (measurable within a range), a continuous random variable is used.
- Q: What are some common probability distributions for random variables? A: Common distributions include the normal, exponential, binomial, Poisson, and uniform distributions. The choice depends on the nature of the random phenomenon.
- Q: How is the expected value calculated? A: For discrete variables, it's the sum of each value multiplied by its probability. For continuous variables, it's the integral of the variable times its probability density function.
- Q: What is the significance of the variance? A: The variance quantifies the spread or dispersion of the variable's values around its mean. A higher variance indicates greater uncertainty.
- Q: How can I visualize the probability distribution of a random variable? A: Histograms, probability mass functions (PMFs) for discrete variables, and probability density functions (PDFs) for continuous variables are commonly used for visualization.
Summary: Understanding the different types of random variables and their properties is key to accurately modeling and analyzing uncertain events.
Transition: Let's now delve deeper into some specific examples to illustrate the concept further.
Tips for Working with Random Variables
Introduction: These tips can help in effectively working with random variables.
Tips:
- Clearly define the random phenomenon and its possible outcomes.
- Identify the type of random variable (discrete, continuous, or mixed).
- Determine the appropriate probability distribution.
- Calculate the expected value and variance to understand the central tendency and spread.
- Utilize statistical software for complex calculations and visualizations.
- Always consider the context and assumptions when interpreting results.
- Validate your model with real-world data to ensure accuracy.
- Explore different probability distributions to find the best fit for your data.
Summary: By following these tips, one can effectively leverage the power of random variables in solving real-world problems involving uncertainty.
Summary of Random Variables
Summary: This guide explored the definition, types, applications, and practical examples of random variables. Understanding random variables is essential for anyone working with probabilistic data. Key aspects covered included discrete and continuous variables, probability distributions, expected value, and variance.
Closing Message: Random variables provide a powerful framework for analyzing and interpreting uncertainty in numerous fields. Further exploration of probability distributions and statistical methods will enhance one's ability to model and predict outcomes in various real-world scenarios.
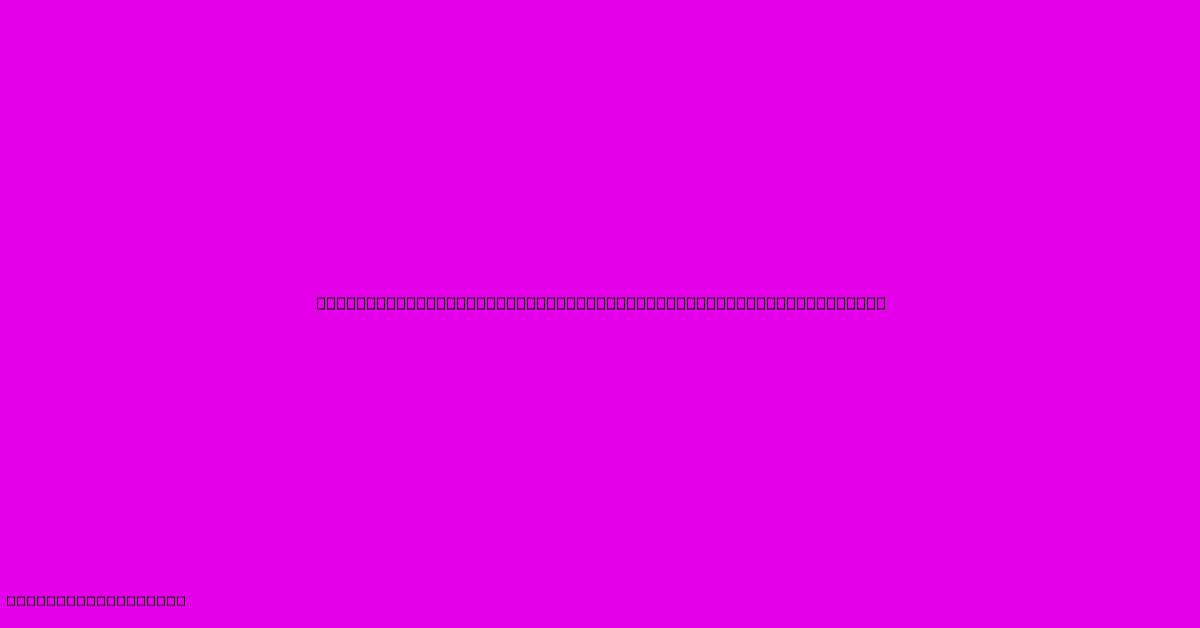
Thank you for taking the time to explore our website Random Variable Definition Types How Its Used And Example. We hope you find the information useful. Feel free to contact us for any questions, and don’t forget to bookmark us for future visits!
We truly appreciate your visit to explore more about Random Variable Definition Types How Its Used And Example. Let us know if you need further assistance. Be sure to bookmark this site and visit us again soon!
Featured Posts
-
How To Request Credit Limit Increase Apple
Jan 12, 2025
-
How To Start An Investment Fund For Real Estate
Jan 12, 2025
-
Real Economic Growth Rate Real Gdp Growth Rate Definition
Jan 12, 2025
-
How Does Hedging Futures Work
Jan 12, 2025
-
How Long Are Credit Card Transactions Pending
Jan 12, 2025