Serial Correlation Definition How To Determine And Analysis
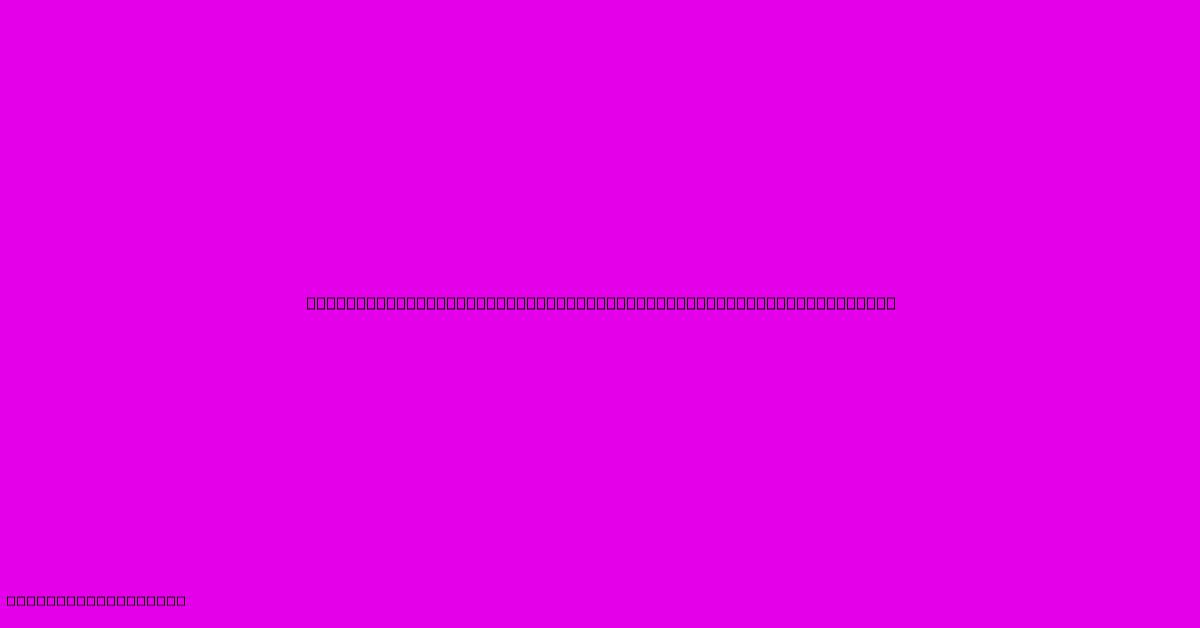
Discover more in-depth information on our site. Click the link below to dive deeper: Visit the Best Website meltwatermedia.ca. Make sure you don’t miss it!
Table of Contents
Unveiling Serial Correlation: Definition, Detection, and Analysis
Hook: Does the past predict the future in your data? The presence of serial correlation, or autocorrelation, significantly impacts the reliability of statistical analyses. Understanding and addressing this phenomenon is crucial for accurate modeling and forecasting.
Editor's Note: This comprehensive guide to serial correlation has been published today.
Relevance & Summary: Serial correlation, the correlation between members of a time series, is a critical concept in statistics and econometrics. This guide explores the definition of serial correlation, various methods for its detection (including Durbin-Watson test, autocorrelation function, and partial autocorrelation function), and techniques for its analysis and mitigation. Understanding serial correlation is crucial for avoiding flawed statistical inferences and building robust models, particularly in time series analysis, financial modeling, and forecasting. Keywords include: autocorrelation, time series analysis, Durbin-Watson test, ACF, PACF, residual analysis, heteroskedasticity, model specification.
Analysis: This guide synthesizes established statistical methods and theoretical frameworks to provide a clear and practical understanding of serial correlation. The analysis draws upon widely accepted statistical tests and visualization techniques to illustrate the detection and interpretation of serial correlation.
Key Takeaways:
- Serial correlation indicates a relationship between observations in a time series that are separated by a specific time lag.
- Several methods exist to detect serial correlation.
- Addressing serial correlation is crucial for accurate statistical inference.
- Understanding the implications of serial correlation improves model building.
Serial Correlation: A Deeper Dive
Subheading: Serial Correlation
Introduction: Serial correlation, also known as autocorrelation, describes the correlation of a time series with a lagged version of itself. It implies that the value of a variable at one time point is dependent on its value at previous time points. This dependency violates the assumption of independence, a cornerstone of many statistical methods, leading to inaccurate conclusions and unreliable forecasts if left unaddressed.
Key Aspects:
- Lag: The time interval between observations. First-order serial correlation (lag 1) indicates a correlation between consecutive observations, second-order (lag 2) between observations two periods apart, and so on.
- Positive Serial Correlation: Observations tend to be similar to their predecessors. A positive value in one period is more likely to be followed by a positive value.
- Negative Serial Correlation: Observations tend to alternate in sign. A positive value is more likely followed by a negative value, and vice versa.
- Implications: Violates assumptions of many statistical tests, leading to biased estimates and incorrect standard errors. This affects hypothesis testing and confidence interval construction.
Discussion: The presence of serial correlation can stem from various sources. Omitted variables, misspecified models, or inherent dynamics within the system being modeled can all contribute to autocorrelation. In economic time series, for instance, inertia in economic activity often leads to positive serial correlation. A country's GDP growth rate might be positively correlated with its growth rate in the previous period. Similarly, in financial markets, momentum effects can result in positive autocorrelation in asset returns, while mean reversion may cause negative autocorrelation. The impact of ignoring serial correlation is that standard errors are underestimated, leading to artificially low p-values and an inflated type I error (rejecting a true null hypothesis).
Subheading: Detecting Serial Correlation
Introduction: Identifying serial correlation is crucial for maintaining the validity of statistical inferences. Several techniques provide valuable tools for detecting its presence.
Facets:
- Durbin-Watson Test: A widely used test for first-order serial correlation in the residuals of a regression model. It tests the null hypothesis of no first-order serial correlation. A value close to 2 indicates no serial correlation, values significantly below 2 suggest positive autocorrelation, and values significantly above 2 indicate negative autocorrelation. However, the Durbin-Watson test's limitations include its inability to detect higher-order serial correlation.
- Autocorrelation Function (ACF): The ACF plots the correlation of a time series with lagged versions of itself across different lags. Significant spikes at specific lags indicate serial correlation at those lags. ACF helps visualize the strength and persistence of autocorrelation.
- Partial Autocorrelation Function (PACF): Similar to the ACF, the PACF measures the correlation between a time series and its lagged values, but it removes the effects of intermediate lags. This makes it more effective in identifying the order of autocorrelation (e.g., determining whether the correlation is primarily first-order, second-order, or higher).
Summary: Combining the Durbin-Watson test with visual inspection of ACF and PACF plots offers a comprehensive approach to detect serial correlation. The choice between ACF and PACF depends on the nature of the time series and the hypotheses being tested.
Subheading: Addressing Serial Correlation
Introduction: Once detected, serial correlation must be addressed to ensure the reliability of statistical analyses. Several strategies can mitigate or correct for autocorrelation.
Further Analysis:
- Model Specification: The most effective way to deal with serial correlation is often to identify and correct the underlying cause. This might involve adding relevant variables to a regression model that have been omitted. A poorly specified model can often lead to autocorrelation in the residuals.
- Generalized Least Squares (GLS): A statistical technique that explicitly models the autocorrelation structure in the data and then uses this information to estimate the model parameters more efficiently.
- Autoregressive Integrated Moving Average (ARIMA) Models: These models explicitly incorporate autocorrelation into the model structure itself, making them particularly suitable for time series data with serial correlation.
- Newey-West Standard Errors: These robust standard errors adjust for the presence of autocorrelation by using a weighted average of the autocovariances. While they do not correct the problem of serial correlation, they help to provide more reliable standard errors and confidence intervals.
Closing: Addressing serial correlation is crucial for drawing valid conclusions from statistical analysis. The appropriate strategy depends on the context and the underlying cause of the autocorrelation. Careful consideration of model specification, combined with techniques like GLS or ARIMA modeling, often effectively mitigates the effects of serial correlation.
FAQ
Introduction: This section addresses frequently asked questions about serial correlation.
Questions:
- Q: What are the consequences of ignoring serial correlation? A: Ignoring serial correlation leads to inefficient and biased parameter estimates, incorrect standard errors, and flawed hypothesis tests.
- Q: Can serial correlation be positive and negative? A: Yes, serial correlation can be either positive (observations tend to be similar) or negative (observations tend to alternate).
- Q: How does serial correlation differ from heteroskedasticity? A: Serial correlation concerns the correlation of errors over time, while heteroskedasticity relates to the variance of errors varying over time.
- Q: Are ARIMA models always necessary for time series with serial correlation? A: No. Sometimes, careful model specification or the use of GLS can sufficiently address serial correlation without resorting to ARIMA models.
- Q: What is the role of the ACF and PACF plots in detecting serial correlation? A: These plots help to visualize the autocorrelation structure of a time series, allowing identification of the order and strength of the autocorrelation.
- Q: Can the Durbin-Watson test detect higher-order autocorrelation? A: No, the Durbin-Watson test is primarily designed to detect first-order serial correlation.
Summary: Understanding the implications of serial correlation and employing appropriate detection and correction methods is essential for accurate statistical analysis.
Tips for Analyzing Serial Correlation
Introduction: This section provides practical tips for effectively analyzing serial correlation.
Tips:
- Visual Inspection: Always start with visual inspection of the time series data and the residuals from any regression model. Look for patterns or trends indicative of autocorrelation.
- ACF and PACF Plots: Use ACF and PACF plots to identify the order and strength of any autocorrelation present.
- Durbin-Watson Test: Employ the Durbin-Watson test as a formal test for first-order serial correlation.
- Model Diagnostics: Pay close attention to the diagnostic checks provided by statistical software, such as the Durbin-Watson statistic or autocorrelation tests.
- Consider Alternative Models: If serial correlation persists after initial modeling, consider alternative model specifications, such as ARIMA models, or GLS estimation.
- Robust Standard Errors: If correction is not fully achievable, use robust standard errors (like Newey-West) to obtain more reliable confidence intervals.
Summary: A systematic approach, combining visual inspection, statistical tests, and careful model selection, is crucial for effectively handling serial correlation.
Summary of Serial Correlation Analysis
Summary: This guide explored the concept of serial correlation, detailing its definition, implications, and methods for detection and analysis. Various techniques including the Durbin-Watson test, ACF, and PACF plots, were presented for identifying serial correlation, and strategies for addressing this issue were discussed. Accurate identification and mitigation of serial correlation are essential for obtaining valid and reliable statistical results.
Closing Message: Understanding and addressing serial correlation is a fundamental skill for any quantitative analyst. By employing the techniques outlined in this guide, researchers and practitioners can significantly enhance the robustness and validity of their statistical models and inferences, ultimately leading to more accurate predictions and informed decision-making.
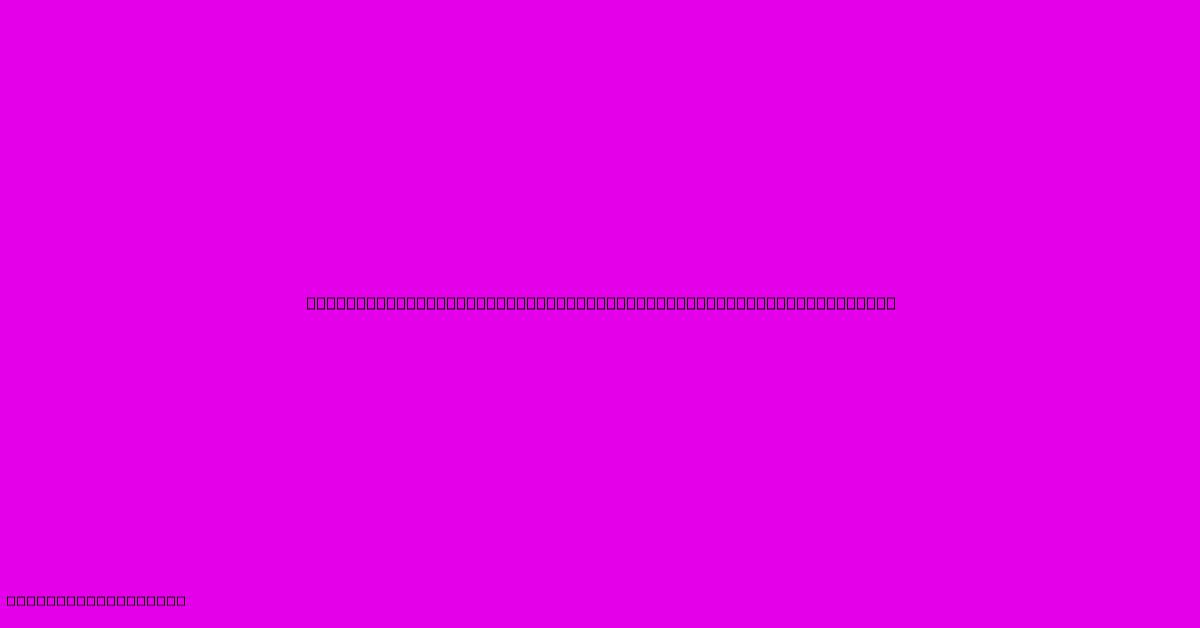
Thank you for taking the time to explore our website Serial Correlation Definition How To Determine And Analysis. We hope you find the information useful. Feel free to contact us for any questions, and don’t forget to bookmark us for future visits!
We truly appreciate your visit to explore more about Serial Correlation Definition How To Determine And Analysis. Let us know if you need further assistance. Be sure to bookmark this site and visit us again soon!
Featured Posts
-
Smart Beta Etf Definition Types Example
Jan 11, 2025
-
How To Budget When You Hate Budgeting
Jan 11, 2025
-
Critical Infrastructure Such As Utilities And Banking Are Which Partners Responsibility
Jan 11, 2025
-
Back Stop Definition How It Works In Offering And Example
Jan 11, 2025
-
What Are The Ethics Of Accounting
Jan 11, 2025