Subindex Definition
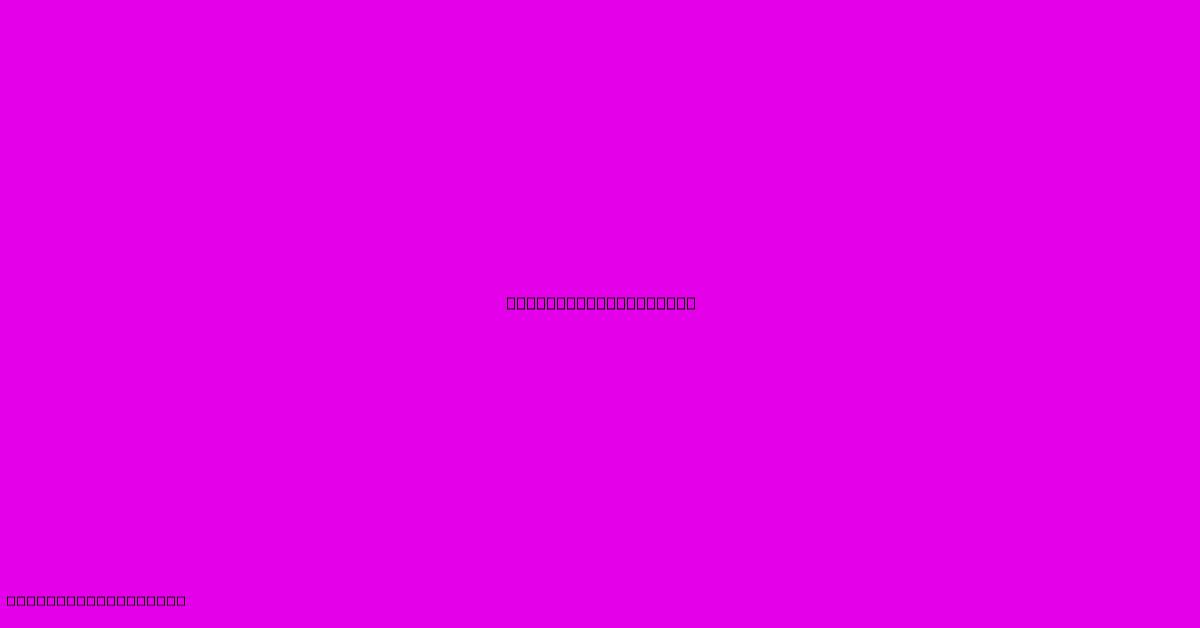
Discover more in-depth information on our site. Click the link below to dive deeper: Visit the Best Website meltwatermedia.ca. Make sure you don’t miss it!
Table of Contents
Unveiling the Power of Subindices: A Comprehensive Guide
Hook: Have you ever needed to dissect a complex dataset, analyzing specific segments within a larger whole? A bold statement: Understanding subindices is crucial for navigating the intricacies of data analysis and decision-making across diverse fields.
Editor's Note: This comprehensive guide to subindices has been published today.
Relevance & Summary: Subindices are essential for granular analysis of complex datasets. This guide explores their definition, applications, creation methods, advantages, and limitations. Readers will gain a thorough understanding of how subindices improve data interpretation and inform strategic decisions in various sectors, including finance, economics, and social sciences. Keywords: subindex, index, composite index, data analysis, weighted average, statistical analysis, sub-index, indicator, economic indicator, financial indicator, social indicator.
Analysis: This guide synthesizes information from various academic publications, industry reports, and practical applications of subindices across different disciplines. The analysis focuses on clarifying the concept, showcasing real-world applications, and outlining the steps involved in constructing effective subindices.
Key Takeaways:
- Subindices are subsets of larger indices, offering deeper insights into specific aspects.
- Creating a subindex requires careful selection of components and weighting methodology.
- Subindices enhance the precision and comprehensiveness of data analysis.
- Appropriate use of subindices improves decision-making in various fields.
Subindex: A Deeper Dive into Data Analysis
Subheading: Subindex
Introduction: A subindex is a subset or component of a larger, composite index. It represents a specific aspect or segment of a broader phenomenon, providing more granular and targeted insights than the overall index can offer. Understanding the construction and interpretation of subindices is crucial for accurate data analysis and strategic decision-making. The significance of subindices lies in their ability to disaggregate complex data into manageable, interpretable components, revealing nuances that might otherwise remain hidden within the overall index.
Key Aspects:
- Component Selection: The first critical step is selecting the components that accurately reflect the specific aspect of the broader phenomenon the subindex aims to measure. These components should be relevant, reliable, and readily available.
- Weighting Methodology: Once the components are selected, a weighting methodology must be determined. This could involve equal weighting, where all components contribute equally, or weighted averaging, where components are assigned weights based on their relative importance or influence. The choice of weighting scheme significantly impacts the subindex's overall value and interpretation.
- Data Aggregation: After assigning weights, the components are aggregated to generate the subindex value. This typically involves a mathematical formula, often a weighted average.
- Normalization: Subindices are frequently normalized to facilitate comparison across different time periods or geographical regions. Normalization ensures that fluctuations in the subindex are not solely due to differences in scale.
Discussion: Let's explore the use of subindices within the context of economic indicators. A composite economic index might track overall economic health. However, a subindex focusing solely on manufacturing output would offer more specific insight into that sector's performance. Similarly, a subindex dedicated to consumer confidence would provide a detailed view of consumer sentiment. Each subindex, carefully constructed, provides a distinct and valuable perspective, contributing to a more holistic understanding of the economic landscape.
The Role of Weighting in Subindex Construction
Subheading: Weighting Methodology
Introduction: The weighting methodology employed significantly influences the subindex's final value and interpretation. Different weighting schemes reflect different priorities and perspectives. The selection of an appropriate weighting methodology is critical for accurately representing the relative importance of each component within the subindex.
Facets:
- Equal Weighting: This simple approach assigns equal weight to each component. It's suitable when all components are considered equally important and there is no strong justification for prioritizing one over another. However, it may not accurately reflect the relative contribution of each component to the overall phenomenon.
- Weighted Averaging: This more sophisticated approach assigns different weights to different components based on their relative importance. Weight assignments often rely on expert judgment, statistical analysis, or historical data. This approach allows for a more nuanced and accurate representation of the phenomenon.
- Principal Component Analysis (PCA): PCA is a statistical technique used to identify the underlying factors driving the variation in a dataset. PCA can be used to assign weights to components in a subindex, reflecting their contribution to the overall variance. This is particularly useful when dealing with many correlated components.
- Risks and Mitigations: Improper weighting can lead to biased results and misinterpretations. Rigorous validation and sensitivity analysis are needed to mitigate these risks. Transparency in the methodology is essential for accurate interpretation.
- Impacts and Implications: The chosen weighting scheme profoundly impacts the subindex's response to changes in its components. A properly weighted subindex provides a more accurate reflection of the targeted aspect of the larger phenomenon, contributing to more reliable analysis and informed decisions.
Summary: The selection of an appropriate weighting methodology is a crucial step in subindex construction. The choice depends on the specific application, data characteristics, and analytical objectives. Transparency and careful consideration are paramount in ensuring the validity and reliability of the resulting subindex.
Applications of Subindices Across Disciplines
Subheading: Applications of Subindices
Introduction: Subindices find widespread application across a range of disciplines, enhancing data analysis and informing strategic decision-making. Their ability to disaggregate complex information into manageable segments makes them valuable tools in diverse fields.
Further Analysis:
- Finance: In finance, subindices are commonly used to track specific sectors of the stock market (e.g., technology, healthcare), providing a granular view of market performance. They are also used in the construction of credit risk indices, allowing for a more refined assessment of borrowers' creditworthiness.
- Economics: Economists utilize subindices to monitor various aspects of economic activity. For example, subindices focusing on employment, inflation, and consumer spending can contribute to a more nuanced understanding of economic trends and forecast accuracy.
- Social Sciences: Subindices play a role in measuring social phenomena. For instance, a broader index measuring social well-being might include subindices related to health, education, and income inequality, allowing for more precise analysis of social progress and disparities.
- Environmental Science: In environmental studies, subindices might monitor aspects of environmental quality (water, air), allowing for targeted analysis of environmental challenges.
Closing: The flexibility and adaptability of subindices make them valuable across disciplines. Their power lies in their capacity to provide detailed insights into specific components within broader systems, leading to more effective data interpretation and more informed decision-making.
FAQ: Subindex
Introduction: This section addresses frequently asked questions concerning subindices.
Questions:
-
Q: What is the difference between a subindex and an index? A: An index is a composite measure of a broader phenomenon; a subindex is a component of a larger index, focusing on a specific aspect.
-
Q: Why are weighted averages often used in subindex construction? A: Weighted averages allow for the incorporation of relative importance of different components, leading to a more accurate representation.
-
Q: What are the potential limitations of subindices? A: Limitations include the potential for bias in component selection and weighting, and the risk of oversimplification.
-
Q: How can the reliability of a subindex be assessed? A: Reliability can be assessed through validation studies, sensitivity analysis, and examination of the data sources and methodologies.
-
Q: Are there any ethical considerations related to the use of subindices? A: Transparency in methodology and data sources is essential to avoid misinterpretations and ensure responsible use.
-
Q: How can subindices be used to improve decision-making? A: By providing detailed insights into specific aspects of a complex phenomenon, subindices help refine analyses and support more informed choices.
Summary: Understanding the nuances of subindex construction and interpretation is crucial for their effective application.
Transition: Let's now explore practical tips for creating effective subindices.
Tips for Creating Effective Subindices
Introduction: This section provides practical tips for constructing and utilizing subindices effectively.
Tips:
-
Clearly define the objective: Articulate the specific aspect of the larger phenomenon the subindex aims to capture.
-
Select relevant components: Choose components that accurately reflect the defined objective.
-
Employ appropriate weighting methodology: Select a weighting scheme that accurately reflects the relative importance of the components.
-
Validate the subindex: Conduct rigorous validation and sensitivity analysis to assess the robustness and reliability of the subindex.
-
Ensure data quality: Utilize high-quality, reliable data sources to minimize error and bias.
-
Document the methodology: Maintain thorough documentation of the process to ensure transparency and reproducibility.
-
Communicate findings clearly: Present the subindex results in a clear, concise, and easily understandable manner.
-
Consider limitations: Acknowledge and address any limitations of the subindex.
Summary: Following these tips ensures that the subindex is well-constructed, reliable, and effectively supports data analysis and decision-making.
Transition: Let's conclude by summarizing the key takeaways from this comprehensive guide.
Summary: Subindex Definition and Application
Summary: This guide provided a comprehensive overview of subindices, covering their definition, construction methods, applications, and limitations. Emphasis was placed on the importance of careful component selection and weighting methodologies for accurate data analysis and informed decision-making. The diverse applications of subindices across numerous fields were highlighted.
Closing Message: Understanding and effectively utilizing subindices is a crucial skill for navigating the complexities of data analysis in a wide array of disciplines. The power of these tools lies in their ability to provide granular insights, revealing subtleties that might otherwise remain obscured within broader indices. As data collection and analysis become increasingly sophisticated, the careful and thoughtful use of subindices will become ever more essential for informed decision-making across sectors.
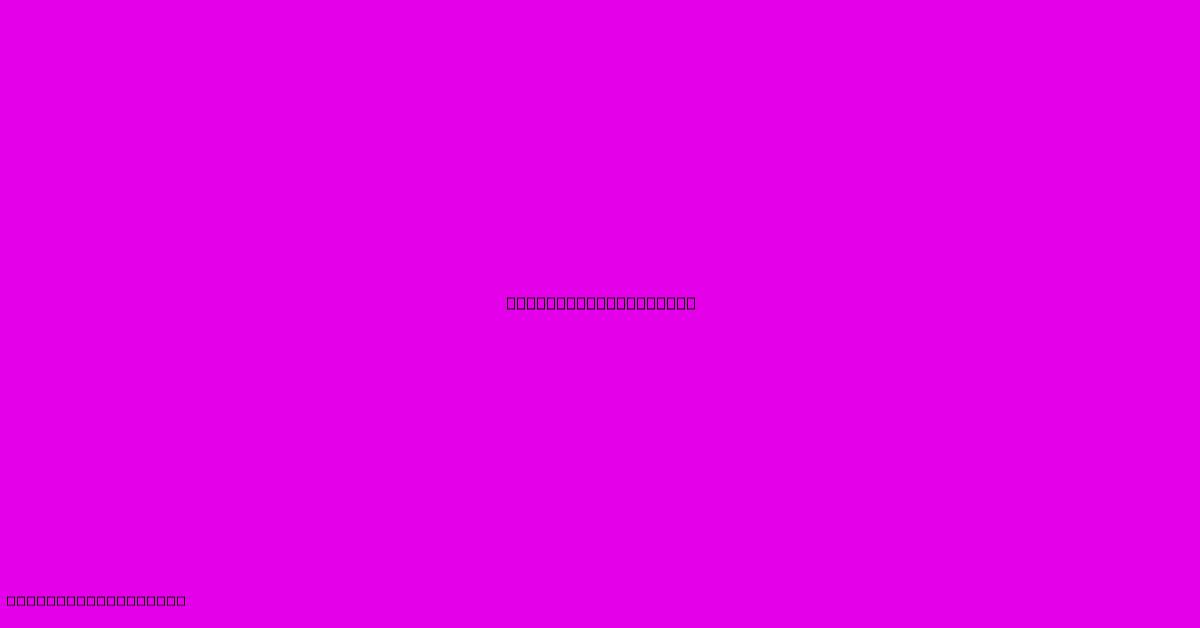
Thank you for taking the time to explore our website Subindex Definition. We hope you find the information useful. Feel free to contact us for any questions, and don’t forget to bookmark us for future visits!
We truly appreciate your visit to explore more about Subindex Definition. Let us know if you need further assistance. Be sure to bookmark this site and visit us again soon!
Featured Posts
-
At Or Better Definition
Jan 11, 2025
-
Special Employer Definition
Jan 11, 2025
-
Statutory Reserves Definition And Examples
Jan 11, 2025
-
What Is Budgeting Process
Jan 11, 2025
-
Smart Assets Definition
Jan 11, 2025