Survival Analysis Definition
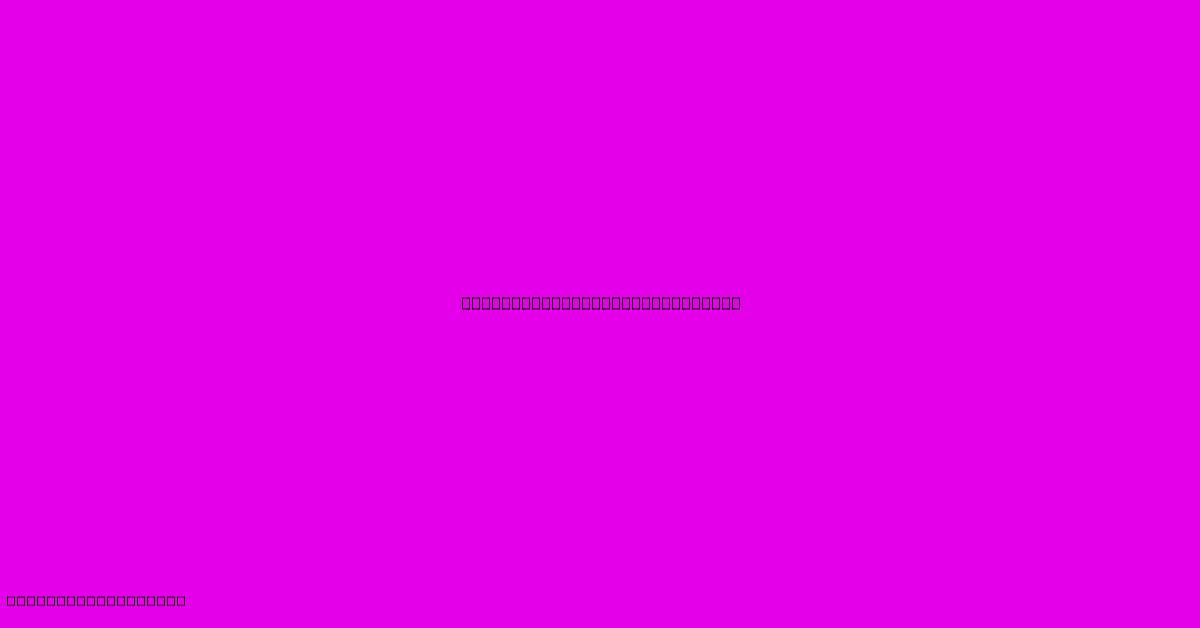
Discover more in-depth information on our site. Click the link below to dive deeper: Visit the Best Website meltwatermedia.ca. Make sure you don’t miss it!
Table of Contents
Unveiling the Secrets of Survival Analysis: A Comprehensive Guide
Hook: Have you ever wondered how long a machine might function before failure, or how effective a new treatment is in prolonging life? Survival analysis provides the powerful statistical tools to answer these questions and more, offering crucial insights across diverse fields.
Editor's Note: This comprehensive guide to Survival Analysis has been published today.
Relevance & Summary: Understanding survival analysis is crucial for researchers and professionals in numerous fields, from medicine and engineering to finance and marketing. This guide provides a detailed explanation of survival analysis, its key concepts (hazard rate, survival function, Kaplan-Meier estimator), and its applications. It will cover censoring, different models (parametric and non-parametric), and the interpretation of results. Readers will gain a solid understanding of how to analyze time-to-event data effectively.
Analysis: This guide draws upon established statistical literature and methodologies in survival analysis. Examples and illustrations are included to clarify complex concepts, making this a practical resource for anyone seeking to learn about and apply these techniques.
Key Takeaways:
- Survival analysis is a statistical method for analyzing time-to-event data.
- Key concepts include the survival function, hazard rate, and censoring.
- Both parametric and non-parametric models are used in survival analysis.
- The Kaplan-Meier estimator is a crucial non-parametric tool.
- Survival analysis finds applications in various fields.
Transition: Let's delve into the intricacies of survival analysis and explore its significant role in diverse applications.
Survival Analysis: A Deep Dive
Subheading: Survival Analysis
Introduction: Survival analysis, also known as time-to-event analysis, is a branch of statistics concerned with modeling the time until an event occurs. This event could be anything from death or disease relapse in medical studies, to equipment failure in engineering, or customer churn in business. A defining characteristic of survival data is that it often involves censoring, meaning that the exact time of the event is unknown for some individuals in the study.
Key Aspects: The core concepts underlying survival analysis include:
- Time-to-event: The duration from a starting point (e.g., treatment initiation) until the occurrence of an event of interest.
- Survival function: This function, often denoted as S(t), describes the probability that an individual will survive beyond time t. It's a decreasing function, starting at S(0) = 1 (everyone is alive at time 0) and approaching 0 as t increases.
- Hazard function (hazard rate): This function, often denoted as λ(t), represents the instantaneous risk of the event occurring at time t, given that the individual has survived until time t. It is the rate of death (or the event) at a specific point in time.
- Censoring: This occurs when the exact event time is not observed for all individuals. Reasons for censoring might include the study ending before the event occurs for some individuals (right censoring), or the individual being lost to follow-up.
Discussion: The interplay between the survival function and the hazard function is critical. A high hazard rate at a specific time implies a rapid decrease in the survival function around that time. Conversely, a low hazard rate suggests a slower decline in the survival function. Understanding this relationship is vital for interpreting the results of a survival analysis. For instance, in a clinical trial comparing two treatments, a lower hazard rate for one treatment implies that individuals receiving that treatment have a greater probability of surviving longer.
Censoring in Survival Analysis
Subheading: Censoring
Introduction: Censoring is an inherent feature of survival data. Ignoring censoring can lead to biased and inaccurate results. This section details the different types and their implications.
Facets:
- Right Censoring: This is the most common type, where the event has not occurred by the end of the study. For example, a patient may still be alive at the end of a clinical trial.
- Left Censoring: This occurs when the event has already occurred before the start of observation. This is less common in survival analysis.
- Interval Censoring: The event time is known to have occurred within an interval. For example, a patient's blood test indicates infection at some point between two visits.
- Roles: Censoring plays a crucial role in survival analysis, significantly influencing the estimation of the survival function. Appropriate methods must be employed to handle censored observations.
- Examples: In a clinical trial, patients who withdraw from the study before the event of interest occurs are right-censored. In a study of equipment failure, machines still functioning at the end of the study period are right-censored.
- Risks and Mitigations: Ignoring censoring leads to biased estimates of survival probabilities. Proper statistical methods, like the Kaplan-Meier estimator, explicitly account for censoring.
- Impacts and Implications: The proportion of censored data significantly impacts the precision of the survival estimates. Higher censoring rates can lead to wider confidence intervals, making it harder to detect significant differences between groups.
Summary: Effective handling of censoring is paramount in survival analysis. Failure to do so can lead to inaccurate conclusions. Methods like Kaplan-Meier estimation provide robust tools to account for censored observations.
Kaplan-Meier Estimator and Parametric Models
Subheading: Kaplan-Meier Estimator and Parametric Models
Introduction: The Kaplan-Meier estimator is a non-parametric method used to estimate the survival function from censored data. Parametric models, in contrast, assume a specific underlying distribution for the survival times.
Further Analysis: The Kaplan-Meier estimator is widely used due to its non-parametric nature, meaning it doesn't assume a specific distribution for the survival times. It provides a step-function estimate of the survival function, with steps occurring at each observed event time. However, for more complex analyses, parametric models that assume a specific distribution (e.g., exponential, Weibull, log-normal) might be preferred. These models allow for more powerful statistical testing and prediction.
Closing: The choice between non-parametric (Kaplan-Meier) and parametric methods depends on the specific research question and the characteristics of the data. While Kaplan-Meier offers flexibility, parametric methods can provide more precise estimations and allow for the incorporation of covariates.
Cox Proportional Hazards Model
Subheading: Cox Proportional Hazards Model
Introduction: The Cox proportional hazards model is a crucial tool for analyzing the effects of multiple covariates on the hazard rate.
Further Analysis: Unlike the Kaplan-Meier estimator, which only estimates the survival function, the Cox proportional hazards model allows researchers to investigate the effect of various factors on survival time. This model assumes that the hazard ratios between different groups remain constant over time (the proportional hazards assumption). This assumption is crucial and should be checked before interpreting the results. The model estimates hazard ratios, which indicate the relative risk of the event for different values of the covariates. For instance, in a clinical trial, it can be used to compare the survival experience between treatment groups, while adjusting for other variables like age and gender.
Closing: The Cox model is a cornerstone of survival analysis, providing a powerful framework for exploring the relationship between multiple factors and survival times. However, its assumption of proportional hazards needs careful consideration and verification.
FAQ
Subheading: FAQ
Introduction: This section addresses common questions about survival analysis.
Questions:
- Q: What is the difference between survival analysis and regression analysis? A: Regression analysis predicts a continuous outcome, while survival analysis models the time until an event occurs, often with censored data.
- Q: What are the assumptions of the Cox proportional hazards model? A: The key assumption is the proportionality of hazards, meaning the hazard ratios between groups remain constant over time.
- Q: How do I handle missing data in survival analysis? A: Strategies include imputation methods or multiple imputation techniques, but careful consideration is needed based on the type and extent of missingness.
- Q: What software packages can be used for survival analysis? A: R, SAS, and SPSS all offer extensive capabilities for survival analysis.
- Q: What are some limitations of survival analysis? A: Assumptions of models (e.g., proportional hazards) might not always hold, and interpretations should be cautious if violated.
- Q: How do I interpret a hazard ratio? A: A hazard ratio of 2 means that the group with the covariate has twice the hazard rate compared to the reference group.
Summary: Understanding these FAQs can help researchers navigate the complexities of survival analysis.
Transition: Effective application of survival analysis requires careful consideration of various aspects.
Tips for Effective Survival Analysis
Subheading: Tips for Effective Survival Analysis
Introduction: This section provides practical tips for conducting successful survival analyses.
Tips:
- Carefully define the event: Clearly define the event of interest to ensure consistent measurement and interpretation.
- Choose the appropriate model: Select the model that best suits your data and research question (Kaplan-Meier, Cox proportional hazards, or parametric models).
- Assess the proportional hazards assumption: Verify the proportionality of hazards assumption before interpreting the Cox model results.
- Address censoring properly: Utilize appropriate statistical methods to account for censoring.
- Visualize the data: Use plots like Kaplan-Meier curves to visually represent survival probabilities.
- Consider confounding factors: Account for potential confounding variables in your analysis using multivariate methods.
- Interpret results cautiously: Understand the limitations of your analysis and interpret results in the context of your study design.
Summary: Following these tips can significantly improve the quality and accuracy of your survival analysis.
Transition: This guide has explored the fundamental concepts and applications of survival analysis.
Summary of Survival Analysis
Summary: This guide has provided a comprehensive overview of survival analysis, encompassing its core concepts, key methods (Kaplan-Meier, Cox proportional hazards models), and the importance of handling censored data. Emphasis has been placed on understanding the hazard rate, survival function, and the various applications across different fields.
Closing Message: Survival analysis remains a powerful tool for understanding time-to-event data. By mastering these techniques, researchers can extract valuable insights and make informed decisions across various disciplines. Further exploration into advanced methodologies and specific applications is encouraged.
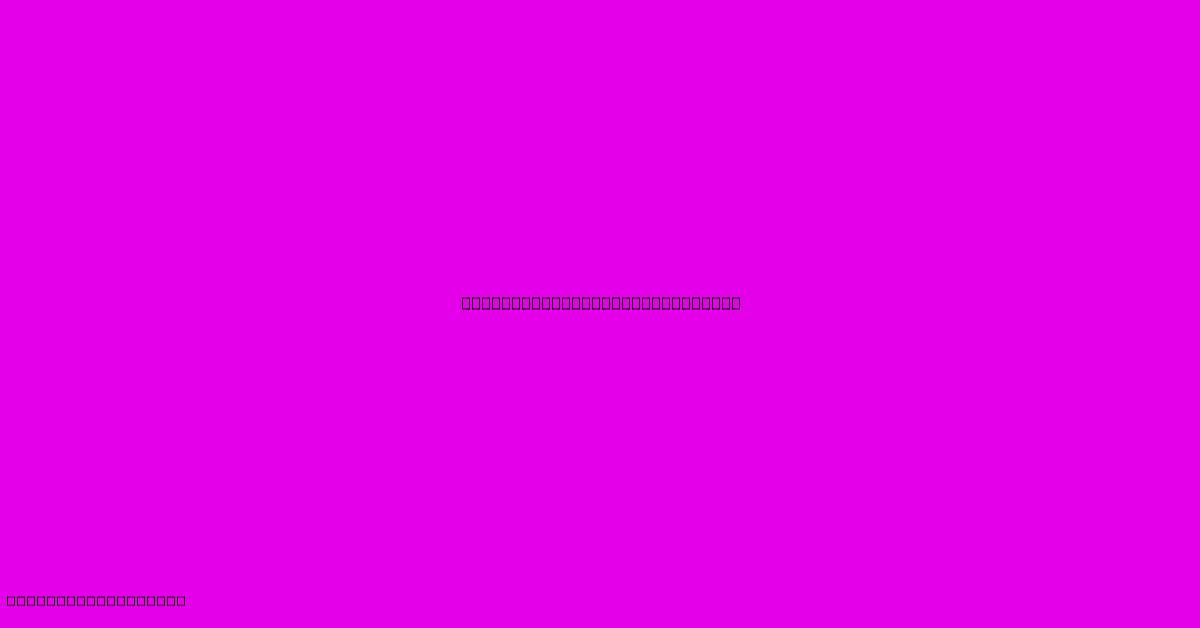
Thank you for taking the time to explore our website Survival Analysis Definition. We hope you find the information useful. Feel free to contact us for any questions, and don’t forget to bookmark us for future visits!
We truly appreciate your visit to explore more about Survival Analysis Definition. Let us know if you need further assistance. Be sure to bookmark this site and visit us again soon!
Featured Posts
-
Taxable Gain Definition
Jan 11, 2025
-
Stop Order Definition Types And When To Place
Jan 11, 2025
-
5 Year Rule Definition For Roth Traditional And Inherited Iras
Jan 11, 2025
-
What Are Social Security Benefits Definition Types And History
Jan 11, 2025
-
Assumption Clause Definition
Jan 11, 2025