Symmetrical Distribution Defined What It Tells You And Examples
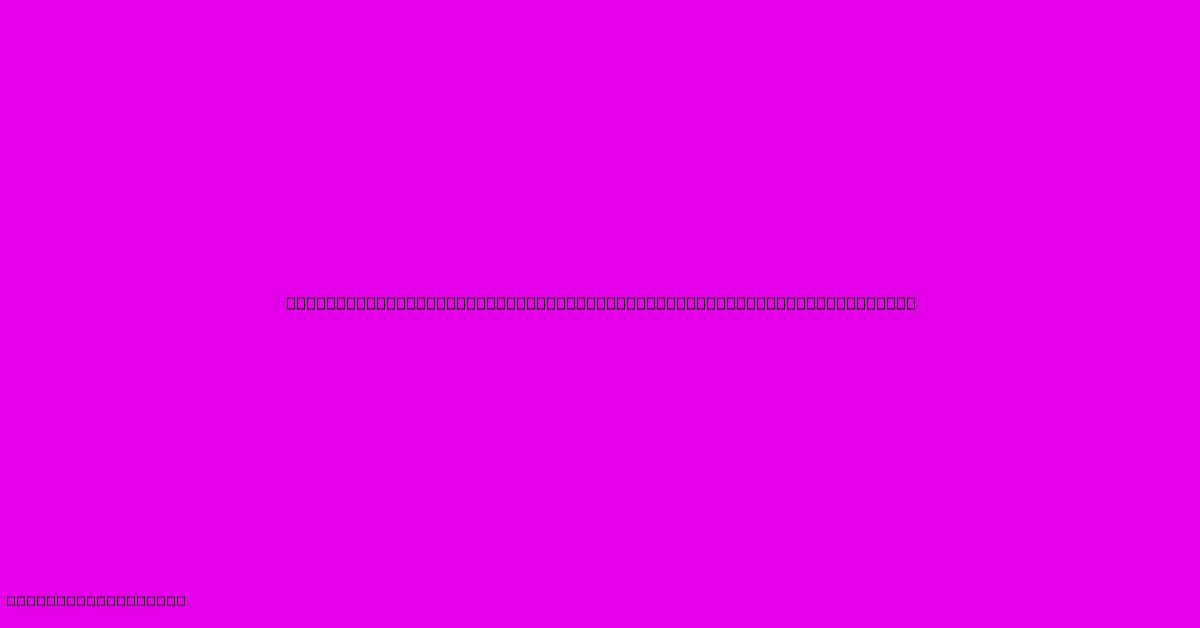
Discover more in-depth information on our site. Click the link below to dive deeper: Visit the Best Website meltwatermedia.ca. Make sure you don’t miss it!
Table of Contents
Unveiling Symmetry: Understanding Symmetrical Distributions and Their Insights
Hook: Have you ever wondered why some data sets appear almost mirror images of themselves? This inherent balance points to a powerful statistical concept: symmetrical distribution. Understanding this reveals crucial insights about the data's central tendency and variability.
Editor's Note: Nota del editor: This article on symmetrical distributions was published today.
Relevance & Summary: Symmetrical distributions are fundamental in statistics, offering valuable insights into data characteristics. This article explores the definition, implications, and real-world applications of symmetrical distributions, utilizing semantic keywords like normal distribution, mean, median, mode, variance, standard deviation, and bell curve. Understanding symmetry helps interpret data accurately and make informed decisions in various fields.
Analysis: This guide synthesizes information from numerous statistical texts and research papers to provide a comprehensive overview of symmetrical distributions. Real-world examples across diverse fields illustrate the practical application of these concepts.
Key Takeaways:
- Symmetrical distributions exhibit a balanced data spread around the central tendency.
- The mean, median, and mode are typically equal in symmetrical distributions.
- The normal distribution is the most common example of a symmetrical distribution.
- Understanding symmetry is crucial for accurate data interpretation and decision-making.
Symmetrical Distribution: A Deep Dive
Subheading: Symmetrical Distribution
Introduction: A symmetrical distribution, in simple terms, is a type of probability distribution where the left and right halves of the distribution are mirror images of each other. This characteristic balance implies a specific relationship between the measures of central tendency: the mean, median, and mode. Understanding this symmetry offers crucial information about the data's central location and the spread of values around that center. The implications extend to statistical analysis, predictive modeling, and risk assessment.
Key Aspects: The primary aspects of symmetrical distributions include:
-
Visual Representation: The most recognizable feature is its visual symmetry. If you were to draw a vertical line through the center of a symmetrical distribution's graph, the two halves would be almost identical. This visual characteristic is a quick and intuitive way to identify whether a distribution is likely symmetrical.
-
Measures of Central Tendency: In a perfectly symmetrical distribution, the mean (average), median (middle value), and mode (most frequent value) are all equal. This equality is a critical indicator of symmetry. However, slight deviations may occur in real-world data due to sampling variability or inherent data noise.
-
Spread and Dispersion: While symmetry describes the shape, the spread of data is indicated by measures like variance and standard deviation. These measure the dispersion or variability of the data points around the central tendency. A symmetrical distribution might have a wide or narrow spread, depending on the variability in the data.
Discussion: Let's delve into each aspect with examples. Imagine the heights of adult women in a large population. If graphed, this data would likely approximate a symmetrical distribution. The mean height, median height, and the modal height (the height occurring most frequently) would be approximately equal. The distribution's spread would tell us how much variability exists in the heights—a large standard deviation implies greater variation, whereas a small standard deviation implies less variability. Conversely, if the distribution was skewed (asymmetrical), it might indicate a specific factor influencing heights, such as a particular health condition impacting a subset of the population. The skewness disrupts the equality of mean, median, and mode.
The Normal Distribution: A Paragon of Symmetry
Subheading: The Normal Distribution
Introduction: The normal distribution, often depicted as a bell curve, is the quintessential example of a symmetrical distribution. Its importance stems from its frequent occurrence in many natural phenomena and its foundational role in statistical inference. Understanding the normal distribution provides a solid basis for comprehending symmetrical distributions more broadly.
Facets:
-
Role: The normal distribution serves as a model for numerous continuous variables, including height, weight, blood pressure, and test scores.
-
Examples: IQ scores, measurement errors, and the distribution of certain physical characteristics often closely resemble a normal distribution.
-
Risks and Mitigations: Misinterpreting a distribution that isn't truly normal can lead to inaccurate conclusions in statistical analysis. Transformation techniques or non-parametric methods can be employed if the data significantly deviates from normality.
-
Impacts and Implications: The central limit theorem states that the average of many independent and identically distributed random variables tends toward a normal distribution, regardless of the original distribution's shape. This makes the normal distribution critical for statistical hypothesis testing and confidence intervals.
Summary: The normal distribution's symmetry, with its equal mean, median, and mode, and its well-defined standard deviation, enables accurate probabilistic statements and inferences about the underlying population. Its pervasiveness underlines the significance of understanding symmetrical distributions in statistical analysis.
Skewness: A Departure from Symmetry
Subheading: Skewness
Introduction: Skewness measures the asymmetry of a probability distribution. While symmetrical distributions have zero skewness, asymmetrical distributions exhibit positive or negative skewness, indicating a long tail on one side of the distribution. Understanding skewness is crucial for interpreting data and selecting appropriate statistical methods.
Further Analysis: A positively skewed distribution has a long right tail, meaning the mean is greater than the median. Examples include income distributions (a few high earners skew the mean upward). Negatively skewed distributions have a long left tail, with the mean less than the median. Examples might include the lifespan of certain machines or the scores on an easy exam (a concentration of high scores).
Closing: Recognizing skewness helps avoid misinterpretations. For example, relying solely on the mean in a skewed distribution can be misleading, as the mean is highly sensitive to outliers. The median is often a more robust measure of central tendency in such cases.
FAQ: Symmetrical Distribution
Subheading: FAQ
Introduction: This section addresses frequently asked questions about symmetrical distributions.
Questions:
-
Q: What are the key characteristics of a symmetrical distribution? A: Equal mean, median, and mode, and a visually balanced distribution around the central tendency.
-
Q: Is the normal distribution the only symmetrical distribution? A: No, while the normal distribution is a prominent example, other symmetrical distributions exist, such as the uniform distribution.
-
Q: How does symmetry affect statistical analysis? A: Symmetry simplifies statistical analysis; many common tests assume or approximate normality.
-
Q: What if my data is not symmetrical? A: Non-parametric methods or data transformations might be necessary.
-
Q: How can I visually check for symmetry? A: Create a histogram or density plot of your data. Look for a mirror-image pattern.
-
Q: What are the implications of ignoring symmetry in data analysis? A: Potentially flawed conclusions and inaccurate inferences.
Summary: Understanding symmetrical distributions and their properties is fundamental for accurate data analysis and interpretation.
Transition: Let’s move to practical tips on identifying and working with symmetrical data.
Tips for Working with Symmetrical Distributions
Subheading: Tips for Identifying Symmetrical Distributions
Introduction: This section provides practical guidance on identifying and working effectively with symmetrical data.
Tips:
-
Visual Inspection: Create a histogram or box plot to visually assess the data's symmetry. Look for a balanced distribution around the center.
-
Compare Mean, Median, and Mode: Calculate these measures of central tendency. In a symmetrical distribution, they will be approximately equal.
-
Q-Q Plot: A Quantile-Quantile plot compares your data's quantiles to the quantiles of a normal distribution. A straight line suggests approximate normality (symmetry).
-
Skewness Test: Statistical tests can quantify the skewness of your data, helping determine its deviation from symmetry.
-
Data Transformation: If your data is skewed, consider transformations (like log or square root) to make it closer to symmetry.
-
Non-parametric Methods: If symmetry cannot be achieved, consider using non-parametric statistical methods that don't assume normality.
-
Consider Context: Remember, perfect symmetry is rare in real-world data. Small deviations from symmetry are often acceptable.
-
Consult an Expert: For complex data sets or critical analyses, seek advice from a statistician.
Summary: Employing these tips helps accurately assess and handle symmetrical (or nearly symmetrical) data, leading to more reliable statistical conclusions.
Transition: This article has explored the definition, importance, and applications of symmetrical distributions.
Summary of Symmetrical Distributions
Summary: This article provided a comprehensive overview of symmetrical distributions, highlighting their definition, characteristics (equal mean, median, and mode), visual representation, and the critical role of the normal distribution. Skewness was discussed as a departure from symmetry, along with practical tips for identifying and working with symmetrical data.
Closing Message: Understanding symmetrical distributions is fundamental for sound statistical analysis and decision-making in many fields. While perfect symmetry is rare, recognizing and acknowledging the level of symmetry present in your data is crucial for accurate interpretation and reliable conclusions. Continued exploration of statistical concepts enhances data literacy and strengthens the foundation for data-driven insights.
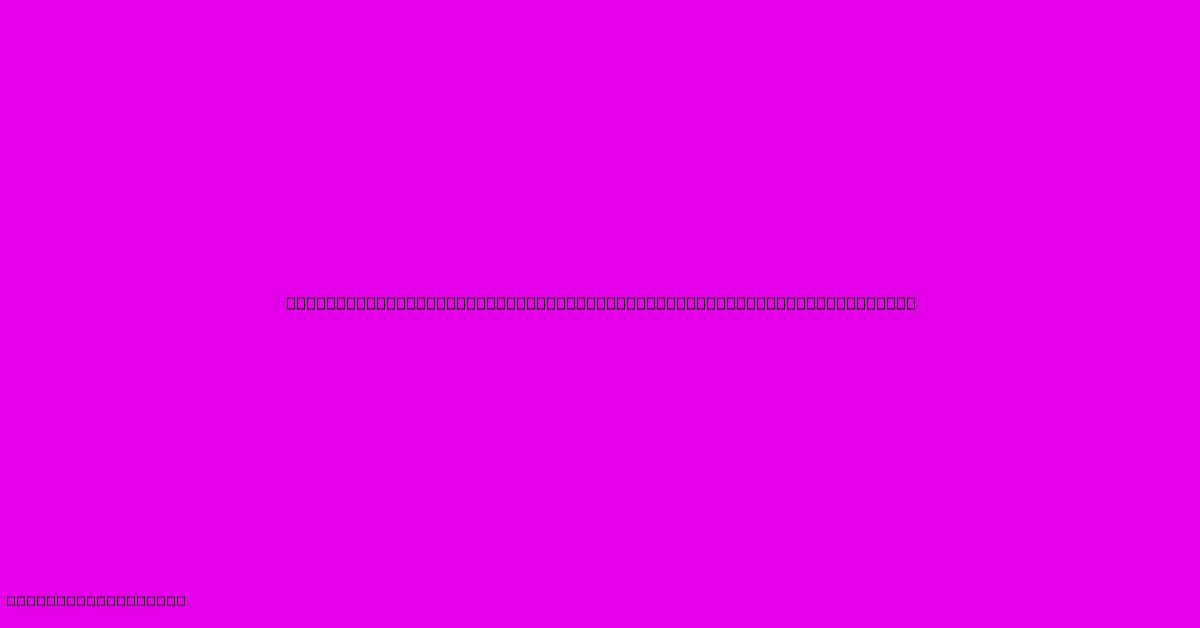
Thank you for taking the time to explore our website Symmetrical Distribution Defined What It Tells You And Examples. We hope you find the information useful. Feel free to contact us for any questions, and don’t forget to bookmark us for future visits!
We truly appreciate your visit to explore more about Symmetrical Distribution Defined What It Tells You And Examples. Let us know if you need further assistance. Be sure to bookmark this site and visit us again soon!
Featured Posts
-
Short Gold Etf Definition
Jan 11, 2025
-
Authorized Investment Definition
Jan 11, 2025
-
Sinkable Bond Definition How It Works Example
Jan 11, 2025
-
Spot Loan Definition
Jan 11, 2025
-
What Is Take Home Pay Definition Vs Gross Pay And Example
Jan 11, 2025