What Is The Pearson Coefficient Definition Benefits And History
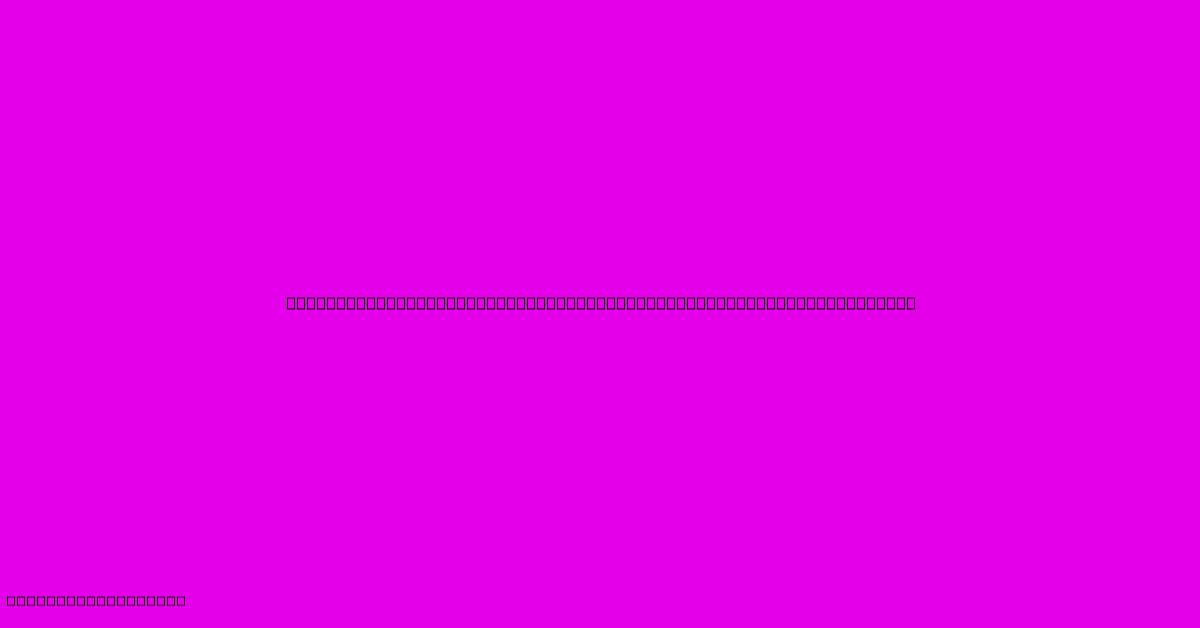
Discover more in-depth information on our site. Click the link below to dive deeper: Visit the Best Website meltwatermedia.ca. Make sure you don’t miss it!
Table of Contents
Unveiling the Pearson Correlation Coefficient: History, Definition, and Benefits
Hook: Have you ever wondered how strongly two variables are related? A powerful statistical tool, the Pearson correlation coefficient, provides a precise measure of this relationship. Its ability to quantify the linear association between variables is invaluable across diverse fields.
Editor's Note: This comprehensive guide to the Pearson correlation coefficient was published today.
Relevance & Summary: Understanding the Pearson correlation coefficient is crucial for researchers, analysts, and anyone working with data. This guide explores its historical development, precise definition, calculation methods, benefits, limitations, and applications. Keywords include: Pearson correlation, correlation coefficient, linear correlation, statistical analysis, bivariate analysis, scatter plot, statistical significance, correlation vs. causation.
Analysis: This guide synthesizes information from numerous statistical textbooks, research articles, and historical accounts to provide a thorough understanding of the Pearson correlation coefficient. It emphasizes its theoretical foundation and practical applications.
Key Takeaways:
- The Pearson correlation coefficient measures the strength and direction of a linear relationship between two variables.
- It ranges from -1 (perfect negative correlation) to +1 (perfect positive correlation), with 0 indicating no linear correlation.
- Its calculation involves standardized scores (z-scores) of the variables.
- Statistical significance testing is essential to determine if the observed correlation is likely due to chance.
- Understanding its limitations is crucial for accurate interpretation.
Subheading: The Pearson Correlation Coefficient
Introduction: The Pearson correlation coefficient, often denoted as r, is a fundamental concept in statistics. It quantifies the linear association between two continuous variables. Understanding its calculation, interpretation, and limitations is vital for proper data analysis. Its widespread use stems from its ability to succinctly summarize the relationship between two variables, facilitating further statistical analysis and informed decision-making.
Key Aspects:
- Measurement of Linear Association: The Pearson correlation coefficient specifically measures the linear relationship. Non-linear relationships might not be accurately represented.
- Strength and Direction: The coefficient's magnitude indicates the strength of the relationship (closer to -1 or +1 implies stronger association), while its sign (+ or -) indicates the direction (positive or negative).
- Standardized Score Calculation: The calculation relies on standardized scores (z-scores) of the data points, ensuring the coefficient is not affected by the units of measurement of the variables.
- Statistical Significance: Determining whether the observed correlation is statistically significant is crucial to avoid drawing incorrect conclusions based on random variation.
Discussion:
The Pearson correlation coefficient is calculated using the following formula:
r = Σ[(xi - x̄)/sx][(yi - ȳ)/sy] / (n-1)
where:
- xi and yi represent individual data points for variables X and Y respectively.
- x̄ and ȳ represent the means of variables X and Y.
- sx and sy represent the standard deviations of variables X and Y.
- n represents the number of data points.
A scatter plot visually represents the relationship between the two variables. A positive correlation shows points clustered along a line with a positive slope, while a negative correlation shows points clustered along a line with a negative slope. A weak correlation shows points scattered more randomly.
Subheading: Historical Context of the Pearson Correlation
Introduction: Understanding the Pearson correlation coefficient's historical context provides valuable insight into its development and limitations. Its origins are intertwined with the evolution of statistical methods in the late 19th and early 20th centuries.
Facets:
- Francis Galton's Contribution: Sir Francis Galton, a pioneer in the field of statistics, laid the groundwork for correlation analysis by studying the relationship between various physical traits. His work on regression analysis provided a foundation for the development of the correlation coefficient.
- Karl Pearson's Formalization: Karl Pearson formalized the concept of the correlation coefficient, refining Galton's work and establishing the widely used formula. This marked a significant advance in the ability to quantify relationships between variables.
- Early Applications: Early applications of the Pearson correlation coefficient were found in fields like biology, anthropology, and genetics, initially used to investigate the relationship between hereditary characteristics.
- Evolution and Refinements: Over time, the methodology surrounding the Pearson correlation coefficient was refined. Statistical significance testing was incorporated, providing a rigorous framework for evaluating the reliability of the calculated coefficient.
- Modern Applications: The coefficient continues to hold significant importance across numerous disciplines, from social sciences and economics to engineering and medicine. Software packages have made its calculation and interpretation readily accessible.
- Limitations: It's crucial to acknowledge the coefficient's limitation: it only measures linear relationships. Non-linear relationships might not be adequately captured. Outliers can disproportionately influence the calculated value, necessitating careful data examination and potentially outlier removal or robust statistical methods.
Summary: The historical development of the Pearson correlation coefficient reflects the evolution of statistical thinking. Its refined methodology and widespread applicability make it an indispensable tool in modern data analysis. However, awareness of its limitations, including its sensitivity to outliers and its focus on linear relationships, remains critical for accurate interpretation.
Subheading: Benefits and Applications of the Pearson Correlation Coefficient
Introduction: The Pearson correlation coefficient offers several significant benefits, making it a widely used tool across numerous fields. Its applications are vast and diverse, reflecting its ability to provide insights into relationships between variables.
Further Analysis:
- Hypothesis Testing: The coefficient facilitates hypothesis testing, enabling researchers to determine the statistical significance of relationships between variables. This allows for more confident conclusions about the presence and strength of associations.
- Predictive Modeling: The coefficient contributes to predictive modeling by providing a measure of the linear relationship between predictor variables and outcomes. Strong correlations can indicate better prediction potential.
- Data Exploration and Visualization: It guides data exploration and visualization. A scatter plot with a calculated Pearson correlation coefficient helps visualize the nature and strength of a relationship.
- Identifying Relationships between Variables: It reveals the existence and strength of relationships between variables, providing insights into potential causal relationships (although correlation does not imply causation).
- Multivariable Analysis: While fundamentally a bivariate tool, the Pearson correlation coefficient forms the foundation for more complex multivariable analyses that assess relationships among multiple variables simultaneously.
Closing: The Pearson correlation coefficient's benefits are numerous, contributing to hypothesis testing, predictive modeling, and a deeper understanding of relationships between variables. Its simple yet powerful ability to quantify linear associations makes it a cornerstone of statistical analysis.
Subheading: FAQ
Introduction: This section addresses frequently asked questions about the Pearson correlation coefficient.
Questions:
- Q: What is the difference between correlation and causation? A: Correlation indicates an association between variables, but it doesn't imply that one variable causes changes in the other. Other factors might be involved.
- Q: Can the Pearson correlation coefficient be used with non-linear data? A: No, it's designed for linear relationships. Non-linear associations require alternative methods such as rank correlation.
- Q: How do outliers affect the Pearson correlation coefficient? A: Outliers can disproportionately influence the coefficient, leading to inaccurate representation of the relationship. Robust methods or outlier removal may be necessary.
- Q: What does a correlation coefficient of 0.8 indicate? A: This indicates a strong positive linear correlation, suggesting a high degree of association between the variables.
- Q: What is the difference between a positive and a negative correlation? A: Positive correlation indicates that as one variable increases, the other also tends to increase. Negative correlation indicates that as one variable increases, the other tends to decrease.
- Q: How is the statistical significance of the Pearson correlation assessed? A: The significance is usually determined using a t-test or similar statistical tests, considering the sample size and the obtained correlation value.
Summary: Understanding the nuances of the Pearson correlation coefficient is critical for its proper application and interpretation.
Subheading: Tips for Using the Pearson Correlation Coefficient Effectively
Introduction: This section provides practical tips for maximizing the value and accuracy of using the Pearson correlation coefficient.
Tips:
- Visualize your data: Always create a scatter plot to visually inspect the relationship before calculating the coefficient. This helps identify non-linearity or outliers.
- Check for normality: The Pearson correlation assumes approximately normal distribution of the data. Transformations or non-parametric methods might be necessary if this assumption is violated.
- Address outliers: Investigate and potentially address outliers, as they can significantly skew the results. Methods include removing or transforming outliers, or using robust correlation measures.
- Consider sample size: A larger sample size generally leads to more reliable results.
- Test for statistical significance: Always test the significance of the correlation to determine if the observed relationship is likely due to chance.
- Avoid causal conclusions: Remember that correlation does not equal causation. Further research might be needed to establish causality.
- Use appropriate software: Statistical software packages simplify the calculation and interpretation of the Pearson correlation coefficient.
Summary: By following these tips, researchers can ensure more accurate and reliable results when using the Pearson correlation coefficient.
Summary: This exploration of the Pearson correlation coefficient has highlighted its historical development, precise definition, calculation methods, benefits, limitations, and widespread applications across diverse fields. Understanding its strengths and limitations is crucial for its effective application.
Closing Message: The Pearson correlation coefficient remains an invaluable statistical tool, offering insights into the relationships between variables. However, responsible interpretation, considering both its benefits and limitations, is crucial for avoiding inaccurate conclusions. Further advancements in statistical methods will continue to refine and expand the applications of this fundamental tool.
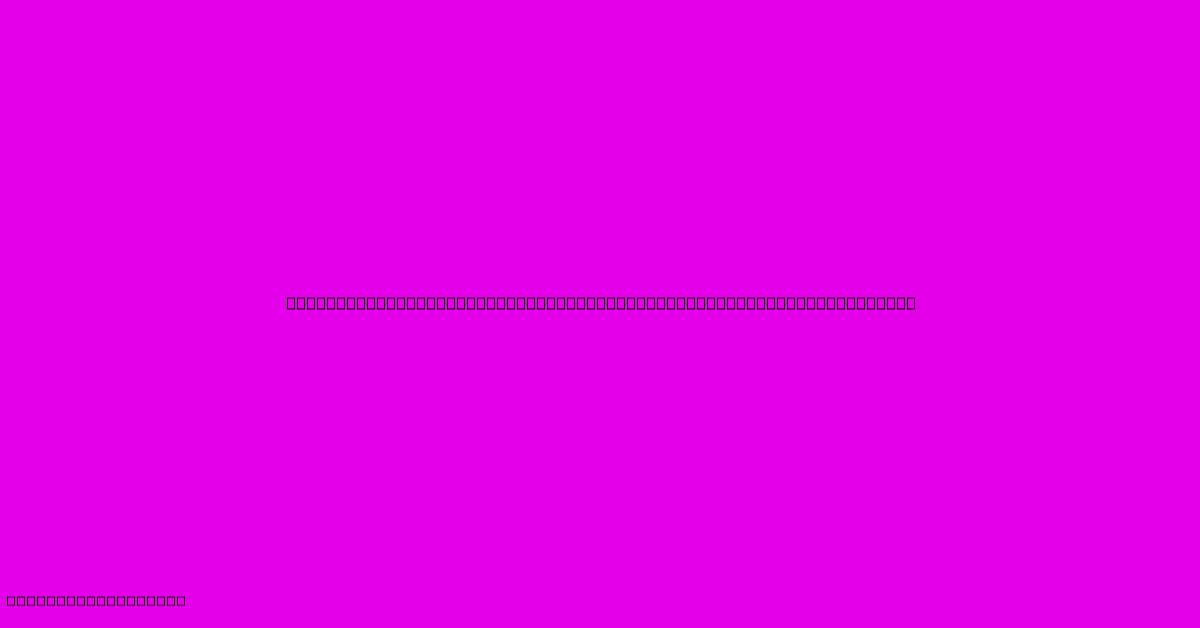
Thank you for taking the time to explore our website What Is The Pearson Coefficient Definition Benefits And History. We hope you find the information useful. Feel free to contact us for any questions, and don’t forget to bookmark us for future visits!
We truly appreciate your visit to explore more about What Is The Pearson Coefficient Definition Benefits And History. Let us know if you need further assistance. Be sure to bookmark this site and visit us again soon!
Featured Posts
-
How To Change My Credit Card On Spotify
Jan 03, 2025
-
What Percentage Of Americans Recieved Financial Education
Jan 03, 2025
-
How Much Does A Hip Replacement Cost With Insurance
Jan 03, 2025
-
How Bad Is A 530 Credit Score
Jan 03, 2025
-
What Is A Petition Definition How It Works And Example
Jan 03, 2025