Z Test Definition Its Uses In Statistics Simply Explained With Example
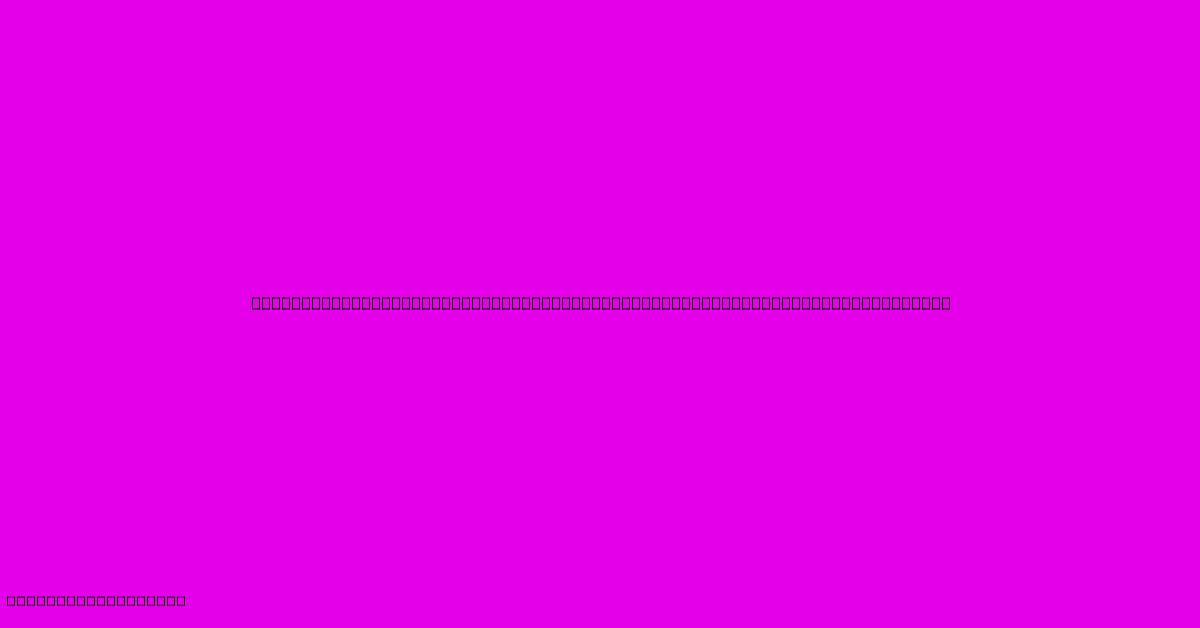
Discover more in-depth information on our site. Click the link below to dive deeper: Visit the Best Website meltwatermedia.ca. Make sure you don’t miss it!
Table of Contents
Unlocking the Power of Z-Tests: A Simple Guide with Examples
Does comparing two population means sound daunting? It doesn't have to be! The z-test, a powerful statistical tool, simplifies this process, providing insights into significant differences between groups. This guide offers a clear, concise explanation of z-tests, their applications, and illustrative examples.
Editor's Note: This comprehensive guide to z-tests was published today.
Relevance & Summary: Understanding z-tests is crucial for researchers, analysts, and anyone working with data involving population means. This guide provides a foundational understanding of z-tests, including their underlying assumptions, calculation methods, and interpretation of results. We'll cover one-sample and two-sample z-tests, along with practical examples to solidify your understanding of statistical significance and hypothesis testing. Key concepts covered include: z-score, standard error, p-value, null hypothesis, and alternative hypothesis. The guide uses clear language, avoiding jargon, making statistical concepts accessible to a wider audience.
Analysis: This guide draws upon established statistical principles and widely accepted methodologies for conducting z-tests. The examples presented are designed to be straightforward and illustrative, demonstrating the practical application of z-tests in real-world scenarios. The focus is on clear explanations and intuitive understanding rather than complex mathematical derivations.
Key Takeaways:
- Z-tests assess differences between population means and sample means or between two population means.
- They require knowledge of population standard deviation (or a large sample size to approximate it).
- Z-tests rely on the normal distribution assumption.
- The p-value determines whether the results are statistically significant.
Z-Tests: Unveiling the Significance of Differences
A z-test is a statistical test used to determine whether there's a significant difference between a sample mean and a population mean (one-sample z-test) or between the means of two independent samples (two-sample z-test). Its power lies in its ability to determine if observed differences are likely due to chance or represent a true effect.
Key Aspect 1: The One-Sample Z-Test
This test compares a sample's mean to a known population mean. Imagine a manufacturer claiming their light bulbs have an average lifespan of 1000 hours. You test a sample of 50 bulbs and find an average lifespan of 980 hours. A one-sample z-test can determine if this difference is statistically significant or simply due to random variation.
Key Aspect 2: The Two-Sample Z-Test
This test compares the means of two independent samples. Consider comparing the average test scores of students taught using two different methods. A two-sample z-test can help determine if there's a significant difference in average scores between the two groups.
One-Sample Z-Test: A Detailed Look
Introduction: The one-sample z-test assesses if a sample mean significantly differs from a known population mean. This test hinges on knowing the population standard deviation.
Facets:
- Role: To determine if a sample's mean is significantly different from a population's mean.
- Example: Comparing the average height of a sample of athletes to the known average height of the general population.
- Risks & Mitigations: Incorrect assumptions about the population standard deviation can lead to inaccurate results. Using a large sample size helps mitigate this risk.
- Impacts & Implications: Determining if a sample represents the population accurately. This has implications for various fields, including medicine, manufacturing, and social sciences.
Summary: The one-sample z-test provides a powerful way to evaluate whether a sample mean differs significantly from a known population mean, given knowledge of the population's standard deviation.
Two-Sample Z-Test: A Comparative Analysis
Introduction: The two-sample z-test compares the means of two independent samples. It is crucial to ensure the samples are independent, meaning the selection of one sample doesn't influence the selection of the other.
Further Analysis: This test is frequently applied in scenarios involving comparing treatment and control groups in experiments, where the means are compared to see if the treatment has any meaningful impact.
Facets:
- Role: To compare the means of two independent samples drawn from two different populations.
- Example: Comparing the average income of males and females in a particular profession.
- Risks & Mitigations: Violation of assumptions (independent samples, normal distribution) can lead to inaccurate results. Larger sample sizes can help mitigate the impact of violating normality.
- Impacts & Implications: This test has far-reaching consequences in A/B testing, comparative studies, and medical research, helping to make informed decisions based on the comparison of two groups.
Closing: The two-sample z-test provides a statistically rigorous way to evaluate the difference between two independent samples, aiding in drawing conclusions about group differences.
Example: One-Sample Z-Test
Let's say a company claims its energy bars have an average of 250 calories per bar. You randomly select 36 bars and find a sample mean of 245 calories with a sample standard deviation of 15 calories. The population standard deviation is known to be 18 calories (from previous large-scale studies). We’ll use a significance level (alpha) of 0.05.
- Null Hypothesis (H0): The average calories are 250.
- Alternative Hypothesis (H1): The average calories are not 250.
- Calculate the z-score: z = (sample mean - population mean) / (population standard deviation / √sample size) = (245 - 250) / (18 / √36) = -1.67
- Find the p-value: Using a z-table or statistical software, the p-value for a two-tailed test (since H1 is not equal to) with a z-score of -1.67 is approximately 0.095.
- Conclusion: Since the p-value (0.095) is greater than the significance level (0.05), we fail to reject the null hypothesis. There isn't enough evidence to conclude that the average calories differ significantly from 250.
Example: Two-Sample Z-Test
Suppose you want to compare the average height of men and women. You sample 50 men with an average height of 175 cm and a standard deviation of 10 cm, and 50 women with an average height of 165 cm and a standard deviation of 8 cm. Assume the population standard deviations are known to be 10 cm and 8 cm respectively. Again, alpha = 0.05.
- Null Hypothesis (H0): There is no difference in average height between men and women.
- Alternative Hypothesis (H1): There is a difference in average height between men and women.
- Calculate the z-score: This involves a slightly more complex calculation accounting for both sample means and standard deviations. Statistical software simplifies this. Let's assume the calculated z-score is 3.5.
- Find the p-value: A z-score of 3.5 has a very small p-value (less than 0.001) for a two-tailed test.
- Conclusion: Since the p-value is less than the significance level, we reject the null hypothesis. There is sufficient evidence to suggest a statistically significant difference in the average height between men and women.
FAQ
Introduction: This section addresses common questions about z-tests.
Questions:
- Q: What are the assumptions of a z-test? A: The data must be normally distributed, or the sample size should be large enough for the Central Limit Theorem to apply. Also, samples should be independent.
- Q: What if the population standard deviation is unknown? A: Use a t-test instead of a z-test.
- Q: How do I choose between a one-sample and two-sample z-test? A: A one-sample z-test compares a sample mean to a known population mean; a two-sample z-test compares the means of two independent samples.
- Q: What is a p-value? A: It’s the probability of observing the obtained results (or more extreme results) if the null hypothesis is true.
- Q: What is a significance level (alpha)? A: The probability of rejecting the null hypothesis when it's actually true. It’s commonly set at 0.05.
- Q: Can z-tests be used with non-normal data? A: While z-tests assume normality, larger sample sizes can mitigate deviations from normality due to the Central Limit Theorem. However, transformations or non-parametric alternatives might be more suitable for severely non-normal data.
Summary: These FAQs clarify key concepts and assumptions associated with conducting and interpreting z-tests.
Tips for Conducting Z-Tests
Introduction: This section provides practical advice for performing z-tests.
Tips:
- Verify Assumptions: Always check if the data meet the assumptions of normality and independence before conducting the test.
- Use Statistical Software: Software packages like R, SPSS, or Python (with libraries like SciPy) greatly simplify calculations.
- Interpret Results Carefully: Don’t just look at p-values; consider effect sizes and the context of the study.
- Understand Limitations: Z-tests rely on certain assumptions; violating these can lead to unreliable results.
- Clearly Define Hypotheses: State your null and alternative hypotheses clearly before conducting the test.
- Choose the Right Test: Select a one-sample or two-sample z-test depending on your research question.
- Report Results Completely: Include details like sample size, means, standard deviations, z-score, and p-value in your reports.
Summary: These tips enhance the accuracy and reliability of your z-tests, leading to more insightful interpretations of your data.
Summary of Z-Tests
Z-tests provide a valuable tool for comparing means, particularly when population standard deviation is known or sample sizes are large. Understanding the assumptions, calculations, and interpretation of z-test results is crucial for drawing meaningful conclusions from statistical analysis.
Closing Message: Mastering z-tests empowers researchers and analysts to make informed decisions based on data, advancing understanding across numerous fields. Further exploration of statistical concepts and advanced statistical methods will build upon the foundational knowledge provided here.
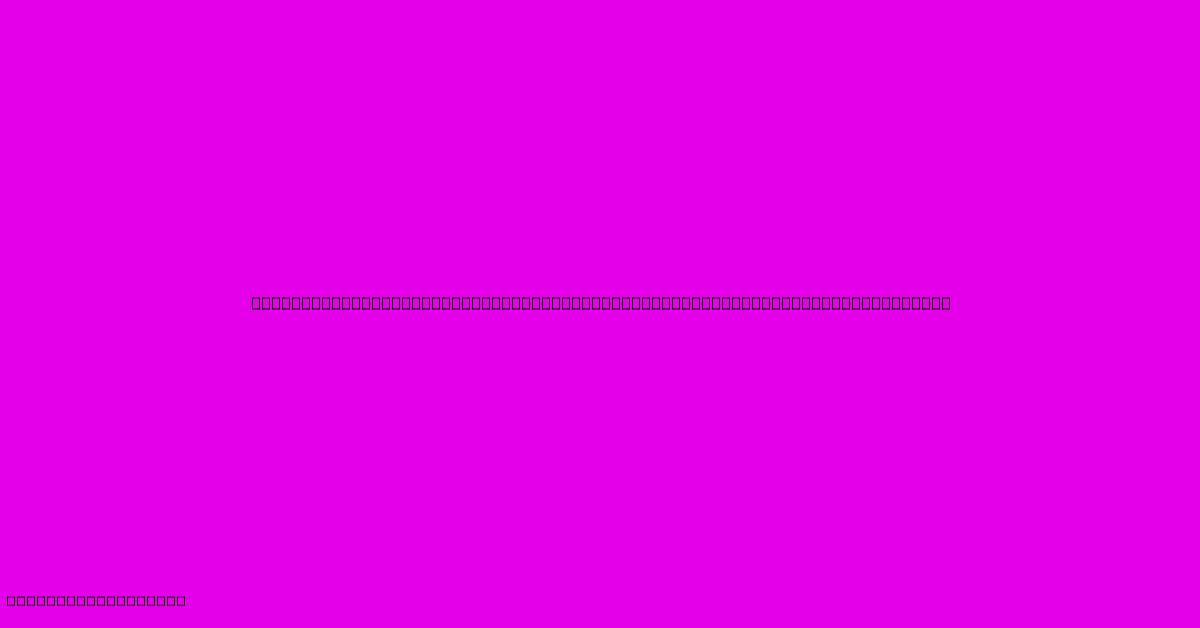
Thank you for taking the time to explore our website Z Test Definition Its Uses In Statistics Simply Explained With Example. We hope you find the information useful. Feel free to contact us for any questions, and don’t forget to bookmark us for future visits!
We truly appreciate your visit to explore more about Z Test Definition Its Uses In Statistics Simply Explained With Example. Let us know if you need further assistance. Be sure to bookmark this site and visit us again soon!
Featured Posts
-
What Is The Grace Period On In Touch Credit Union Auto Payment
Jan 06, 2025
-
Consumer Interest Definition
Jan 06, 2025
-
How To Sue The Irs
Jan 06, 2025
-
How Much Do Colonoscopies Cost With Insurance
Jan 06, 2025
-
Constructive Receipt Definition How It Works And Example
Jan 06, 2025