Composite Index Definition Types And Examples
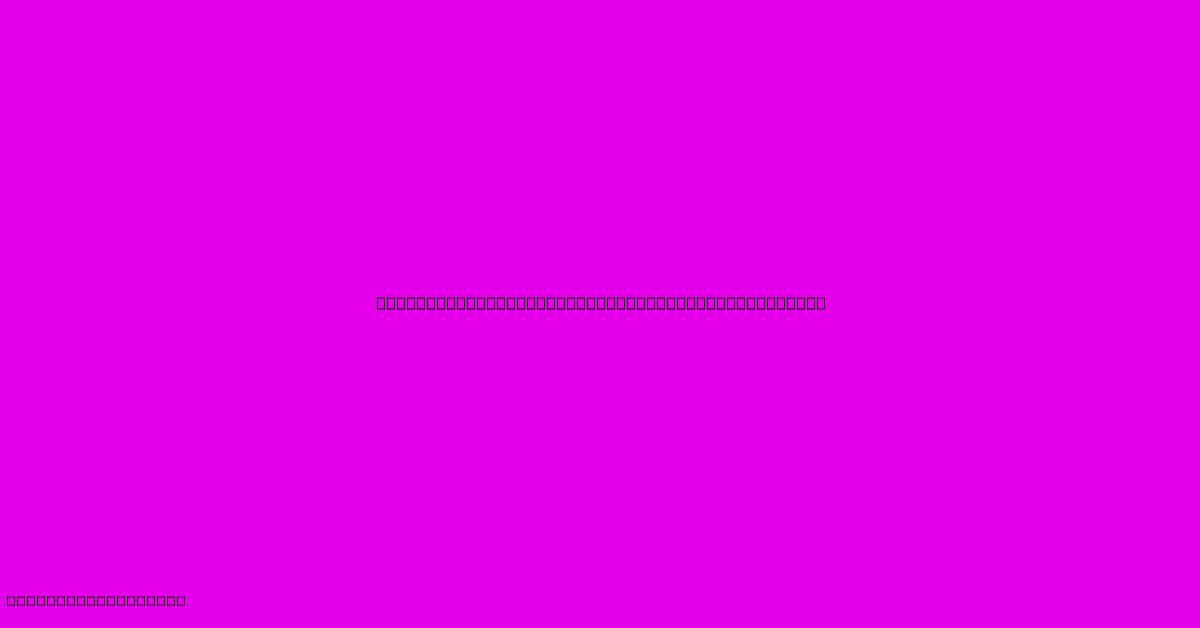
Discover more in-depth information on our site. Click the link below to dive deeper: Visit the Best Website meltwatermedia.ca. Make sure you don’t miss it!
Table of Contents
Unveiling Composite Indices: Definitions, Types, and Examples
Hook: Do you know how economists and analysts condense vast amounts of economic data into easily understandable indicators? The answer lies in the power of composite indices. These powerful tools provide crucial insights into complex systems.
Editor's Note: This comprehensive guide to composite index definition, types, and examples was published today.
Relevance & Summary: Understanding composite indices is vital for anyone analyzing economic trends, market performance, or societal well-being. This article will explore the definition, various types, and real-world examples of composite indices, clarifying their construction and applications. We'll delve into the methodology behind their creation, highlighting the importance of weighting schemes and data selection. Keywords covered include: composite index, index construction, weighting schemes, economic indicators, financial indices, social indices, environmental indices.
Analysis: This article synthesizes information from academic research papers on index number theory, statistical publications from international organizations (like the World Bank and IMF), and publicly available data on various composite indices. The examples provided are drawn from reputable sources to ensure accuracy and relevance.
Key Takeaways:
- Composite indices are aggregations of multiple indicators.
- Different weighting schemes impact index results significantly.
- Various types exist, catering to specific needs (e.g., economic, social, environmental).
- Careful consideration of data quality and selection is crucial.
- Indices are valuable for tracking trends and facilitating comparisons.
Transition: Let's now embark on a detailed exploration of composite indices, starting with a precise definition.
Composite Index: A Deep Dive
Introduction: A composite index is a single numerical value derived from combining multiple individual indicators, each reflecting a specific aspect of a broader concept. These indicators are often disparate in nature, requiring careful selection and weighting to create a meaningful aggregate measure. The significance lies in the ability to synthesize complex realities into a readily understandable metric for monitoring and analysis.
Key Aspects:
- Indicator Selection: The process begins with identifying relevant indicators that accurately capture the essence of the phenomenon being measured. This selection involves careful consideration of data availability, reliability, and relevance to the research question.
- Data Normalization: Given that indicators often have different units and scales, normalization techniques (like standardization or min-max scaling) are applied to ensure comparability before aggregation.
- Weighting Schemes: Weights are assigned to each indicator to reflect its relative importance in contributing to the overall composite score. Different weighting methods exist, each with its strengths and limitations. Common methods include equal weighting, expert-based weighting, and principal component analysis (PCA).
- Aggregation Method: Once the indicators are normalized and weighted, an aggregation method (e.g., simple averaging, weighted averaging) combines them into a single composite index value.
Discussion: The choice of weighting scheme significantly influences the final index value and interpretation. For instance, equal weighting treats all indicators as equally important, while expert-based weighting incorporates subjective judgments based on knowledge and experience. PCA, a statistical technique, assigns weights based on the indicators' variance and correlation, aiming to capture the maximum amount of information with the fewest variables. The chosen aggregation method (simple or weighted average, geometric mean, etc.) also affects the final index value. Different methods may be appropriate for different applications and datasets.
Weighting Schemes: A Closer Look
Introduction: The choice of weighting scheme is critical to the index's validity and interpretation. The weighting reflects the relative importance assigned to each individual indicator within the composite measure.
Facets:
- Equal Weighting: This assigns the same weight to each indicator. It is simple to understand and implement but may not accurately reflect the relative importance of each component if the indicators differ significantly in influence.
- Expert-Based Weighting: This involves soliciting opinions from experts to determine the weights. It incorporates qualitative judgment, but subjectivity can lead to inconsistencies and potential biases.
- Data-Driven Weighting: This uses statistical methods like PCA or factor analysis to derive weights objectively based on the data's inherent structure and relationships between indicators.
- Policy-Driven Weighting: This aligns weights with policy priorities, which can be valuable for specific goals but may neglect other aspects.
Summary: Selecting the optimal weighting scheme depends on the context, data characteristics, and intended use of the composite index. Transparency in the weighting method is essential for accurate interpretation and avoiding bias.
Examples of Composite Indices
Introduction: Numerous composite indices exist across diverse fields, providing valuable tools for monitoring and evaluating complex phenomena.
Further Analysis:
- Human Development Index (HDI): The HDI, developed by the United Nations Development Programme (UNDP), combines life expectancy, education levels, and per capita income to measure human development. It's a widely used indicator of societal well-being.
- Ease of Doing Business Index: The World Bank's Ease of Doing Business Index ranks countries based on the ease of starting and operating a business. It considers various factors, including regulations, infrastructure, and bureaucratic processes.
- Global Innovation Index (GII): The GII measures innovation capacity and performance across countries, combining factors such as research and development, intellectual property protection, and technological adoption.
- Environmental Performance Index (EPI): This index, jointly produced by Yale and Columbia universities, assesses environmental health and ecosystem vitality using indicators related to environmental quality, resource management, and climate change mitigation.
- Financial Market Indices (e.g., Dow Jones Industrial Average, S&P 500): These track the performance of a specific set of companies, reflecting market trends and economic conditions.
Closing: These examples illustrate the wide range of applications of composite indices across diverse areas, demonstrating their value in providing a holistic and simplified view of complex systems.
FAQ: Composite Indices
Introduction: This section addresses commonly asked questions regarding composite indices.
Questions:
- Q: What are the limitations of composite indices?
- A: Composite indices simplify complex realities, potentially masking important nuances or variations within the data. The choice of indicators and weighting schemes can significantly influence the results, introducing potential biases. Data quality is also crucial, and the reliability of the index depends on the quality of the underlying data.
- Q: How are composite indices used in policymaking?
- A: They provide policymakers with a concise overview of complex issues, allowing for targeted interventions and monitoring of progress towards specific goals.
- Q: What are some common pitfalls in constructing composite indices?
- A: Pitfalls include inadequate indicator selection, inappropriate weighting schemes, neglecting data quality issues, and a lack of transparency in the methodology.
- Q: Can composite indices be used for cross-country comparisons?
- A: Yes, provided the methodology is robust and the data are comparable across countries. However, cultural and institutional differences need to be considered.
- Q: How frequently are composite indices updated?
- A: The frequency varies depending on the index and data availability, ranging from annual updates to real-time tracking.
- Q: What is the difference between a composite index and a simple index?
- A: A simple index typically tracks a single variable, while a composite index aggregates multiple indicators.
Summary: Understanding the limitations and potential biases of composite indices is crucial for accurate interpretation and responsible use.
Transition: To effectively utilize composite indices, several best practices should be followed.
Tips for Constructing Effective Composite Indices
Introduction: Building a reliable and meaningful composite index requires careful planning and execution.
Tips:
- Clearly Define the Objective: Specify the purpose and intended use of the index.
- Select Relevant Indicators: Choose indicators that are reliable, readily available, and accurately capture the phenomenon of interest.
- Justify the Weighting Scheme: Transparency in the selection and justification of weights is crucial.
- Ensure Data Quality: Utilize high-quality, reliable data sources and implement appropriate quality checks.
- Document the Methodology: Provide a detailed description of the index's construction for reproducibility and transparency.
- Test and Validate: Evaluate the index's performance and robustness through sensitivity analysis and comparisons with alternative approaches.
- Communicate Clearly: Present the results in a clear, concise, and accessible manner.
- Consider Dynamic Weighting: For evolving systems, explore methods that allow weights to adjust over time.
Summary: Following these guidelines increases the likelihood of creating a robust, reliable, and informative composite index.
Transition: This exploration of composite indices has highlighted their importance as powerful tools for summarizing complex information.
Summary: A Synthesis of Composite Indices
Summary: This article has provided a comprehensive overview of composite indices, covering their definition, types, construction methods, and examples across various fields. Key aspects such as indicator selection, weighting schemes, and data normalization were analyzed, illustrating their impact on the final index value and interpretation.
Closing Message: Composite indices offer invaluable insights into multifaceted issues, enabling efficient monitoring and informed decision-making. However, awareness of their limitations and careful consideration of methodological choices are critical for accurate interpretation and meaningful use. Further research into advanced weighting techniques and the incorporation of big data could enhance the power and applicability of these powerful tools.
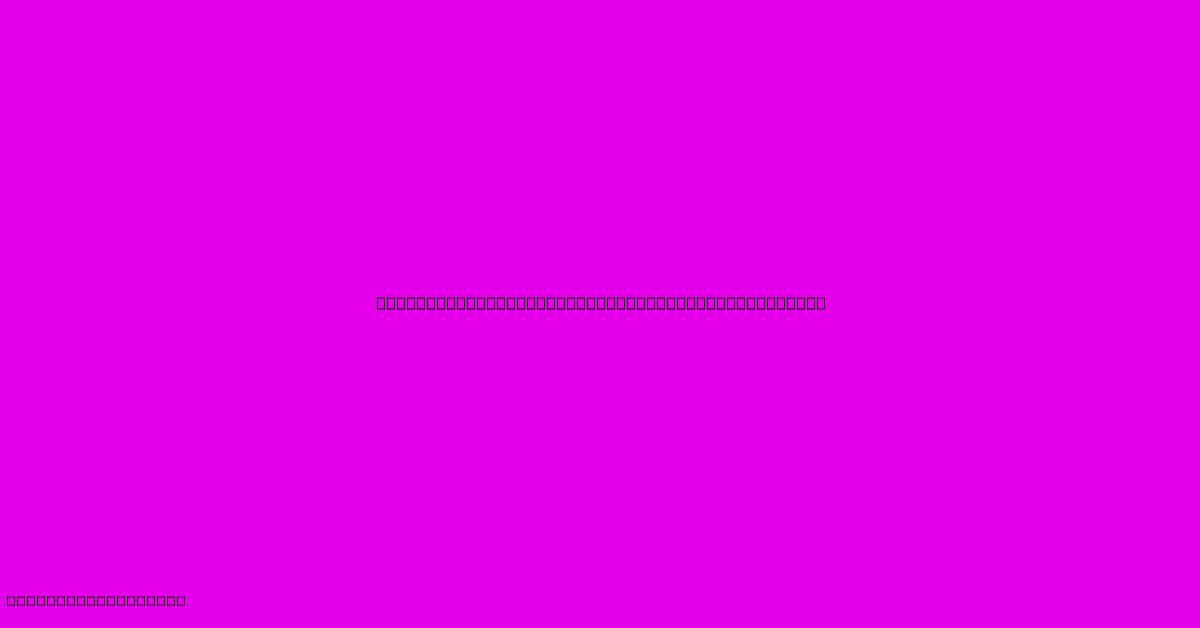
Thank you for taking the time to explore our website Composite Index Definition Types And Examples. We hope you find the information useful. Feel free to contact us for any questions, and don’t forget to bookmark us for future visits!
We truly appreciate your visit to explore more about Composite Index Definition Types And Examples. Let us know if you need further assistance. Be sure to bookmark this site and visit us again soon!
Featured Posts
-
Condominium Fee Definition How It Works Pros And Cons
Jan 06, 2025
-
How To Record Credit Card Payments In Quickbooks Online
Jan 06, 2025
-
Zmk Zambian Kwacha Definition
Jan 06, 2025
-
Cross Price Elasticity Definition Formula For Calculation And Example
Jan 06, 2025
-
Z Test Definition Its Uses In Statistics Simply Explained With Example
Jan 06, 2025