Dynamic Scoring Definition
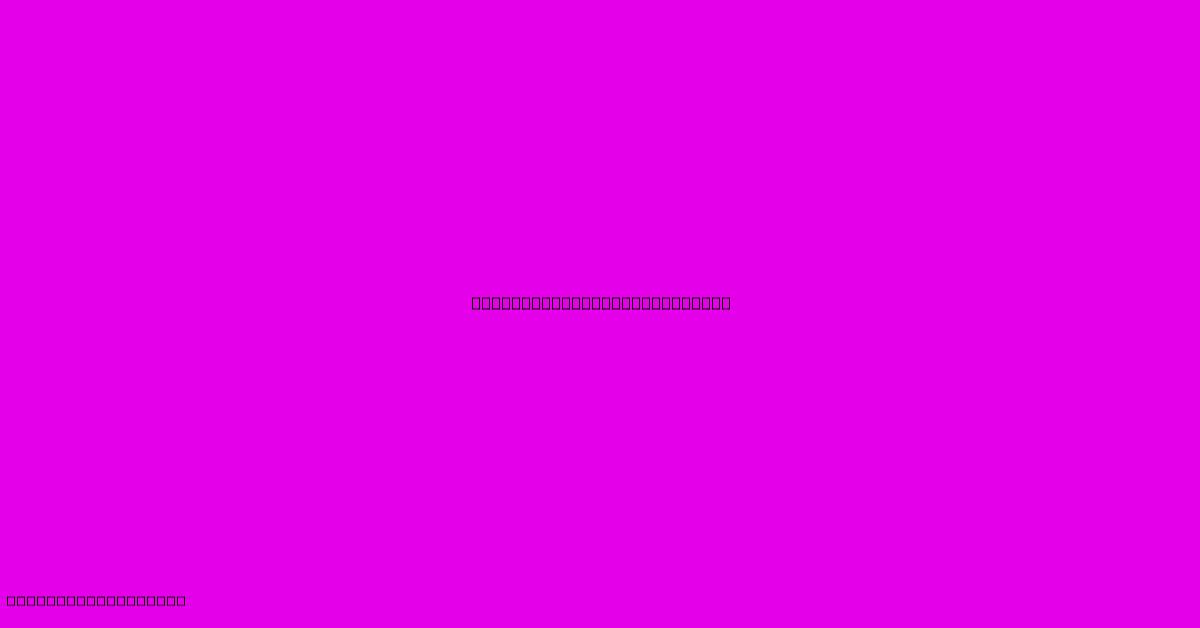
Discover more in-depth information on our site. Click the link below to dive deeper: Visit the Best Website meltwatermedia.ca. Make sure you don’t miss it!
Table of Contents
Unveiling the Power of Dynamic Scoring: A Comprehensive Guide
Hook: Does your current scoring system truly reflect the ever-changing landscape of your business? A bold assertion: Dynamic scoring offers a powerful solution, adapting to real-time data to provide far more accurate and insightful evaluations than static methods.
Editor's Note: This comprehensive guide to dynamic scoring has been published today.
Relevance & Summary: Understanding and implementing dynamic scoring is crucial for businesses across various sectors. This guide explores the definition, benefits, applications, and challenges of dynamic scoring, providing a framework for its effective use in improving decision-making processes, risk assessment, and performance optimization. Keywords include: dynamic scoring, lead scoring, predictive modeling, real-time data, machine learning, risk assessment, credit scoring, customer segmentation.
Analysis: This guide synthesizes information from academic research on predictive modeling, statistical analysis of real-world dynamic scoring applications (including case studies from diverse industries), and best practices outlined by industry experts.
Key Takeaways:
- Dynamic scoring adapts to changing data, offering more accurate and timely results than static systems.
- Its applications span numerous industries, enhancing decision-making and risk management.
- Effective implementation requires careful data selection, model building, and ongoing monitoring.
- Challenges include data quality, model complexity, and the need for continuous adaptation.
Dynamic Scoring: Adapting to the Ever-Changing Landscape
Introduction: Dynamic scoring represents a significant advancement over traditional, static scoring systems. Unlike static scoring, which uses a fixed set of criteria and weights, dynamic scoring leverages real-time data and often incorporates machine learning algorithms to continuously recalculate scores based on new information. This adaptability makes it particularly valuable in environments characterized by volatility and frequent change.
Key Aspects: The core components of dynamic scoring include: data ingestion (collecting and processing relevant data from diverse sources), model building (developing algorithms that weigh and combine data points), scoring engine (the system that calculates scores based on the model), and feedback loop (a mechanism for continuous model refinement based on outcomes).
Discussion:
The power of dynamic scoring stems from its ability to incorporate various data sources. These sources can range from structured data (e.g., CRM data, transactional records) to unstructured data (e.g., social media sentiment, customer reviews). The weighting assigned to each data point isn't fixed; instead, machine learning algorithms can identify the most predictive variables and adjust weights accordingly. This dynamic adjustment allows the system to adapt to evolving patterns and trends, enhancing its accuracy over time. For instance, in lead scoring, a dynamic system might give greater weight to website engagement metrics during certain periods or adjust based on past campaign performance, providing far more granular and predictive insights.
Dynamic Scoring in Different Applications
Lead Scoring
Introduction: In the context of lead scoring, dynamic scoring revolutionizes sales and marketing efforts. It moves beyond simple point systems, incorporating engagement levels, website behavior, and demographic data to predict the likelihood of conversion.
Facets:
- Role: Dynamic lead scoring identifies high-potential leads, enabling sales teams to prioritize their efforts and improve conversion rates.
- Examples: A dynamic lead scoring model might increase the weight given to a prospect who downloads a whitepaper, attends a webinar, or engages significantly with the company's social media.
- Risks and Mitigations: Overly complex models can be difficult to interpret; simpler models, while less accurate, offer greater transparency. Regular model validation and data cleansing mitigate this.
- Impacts and Implications: Improved lead prioritization, increased sales conversion rates, optimized marketing spend, and a better understanding of customer behavior.
Summary: Dynamic lead scoring bridges the gap between marketing and sales, providing a more data-driven and efficient approach to lead management, ultimately driving revenue growth.
Credit Scoring
Introduction: Dynamic credit scoring is transforming the lending industry, providing a more nuanced and accurate assessment of creditworthiness than traditional methods.
Further Analysis: Unlike static models that rely primarily on historical data, dynamic credit scoring incorporates real-time data, such as transactional activity, payment patterns, and even social media activity, to assess the borrower's current creditworthiness. This allows lenders to make more informed decisions, reducing the risk of defaults while potentially expanding access to credit for deserving borrowers.
Closing: Dynamic credit scoring is a powerful tool for improving lending decisions, but it’s crucial to manage ethical concerns and ensure responsible implementation to avoid bias and discrimination.
Customer Segmentation
Introduction: Dynamic scoring offers a robust method for customer segmentation, enabling businesses to personalize their offerings and enhance customer experience.
Further Analysis: By integrating data on purchasing behavior, website interactions, and customer service interactions, a dynamic model can categorize customers into segments with varying levels of value or engagement. This enables targeted marketing campaigns, tailored product recommendations, and proactive customer support, fostering customer loyalty and driving sales.
Closing: The ability of dynamic scoring to adapt to evolving customer behavior is a key advantage, ensuring the segments remain relevant and actionable over time.
FAQs on Dynamic Scoring
Introduction: This section addresses common questions surrounding the implementation and application of dynamic scoring.
Questions:
-
Q: What types of data are suitable for dynamic scoring? A: Both structured and unstructured data can be utilized, including transactional data, behavioral data, demographic data, and social media data.
-
Q: What are the potential challenges of implementing dynamic scoring? A: Challenges include data quality issues, model complexity, the need for ongoing monitoring and recalibration, and the risk of bias in data and algorithms.
-
Q: How often should dynamic scoring models be updated? A: The frequency of updates depends on the volatility of the data and the business context. Regular monitoring and testing are crucial to ensure the model's accuracy.
-
Q: Can dynamic scoring be applied to small businesses? A: Yes, although the complexity of implementation might vary depending on the available resources and data volume.
-
Q: What are the ethical considerations associated with dynamic scoring? A: It’s critical to ensure fairness and avoid bias in data selection and model development. Transparency and explainability are also crucial.
-
Q: How does dynamic scoring differ from static scoring? A: Dynamic scoring adapts to changing data, providing more accurate and timely results, unlike static systems which use fixed weights and criteria.
Summary: Understanding the implications and potential challenges of dynamic scoring is vital for its successful implementation.
Transition: Let’s move on to practical tips for implementing dynamic scoring effectively.
Tips for Implementing Dynamic Scoring
Introduction: This section outlines key steps to successfully implement a dynamic scoring system.
Tips:
-
Define Clear Objectives: Establish specific, measurable, achievable, relevant, and time-bound goals for your dynamic scoring system. What do you aim to achieve? Improved lead conversion? Better risk assessment? Enhanced customer segmentation?
-
Data Quality is Paramount: Ensure data accuracy, completeness, and consistency. Garbage in, garbage out – a poorly curated dataset will result in an inaccurate model.
-
Choose the Right Algorithm: Select an algorithm appropriate for your data and objectives. Consider factors like data volume, complexity, and the desired level of explainability.
-
Develop a Robust Testing Strategy: Thoroughly test your model on historical and real-time data to validate its accuracy and identify potential biases.
-
Monitor and Refine Continuously: Dynamic scoring is an iterative process. Regularly monitor model performance, make adjustments as needed, and retrain the model with new data to maintain its accuracy and effectiveness.
-
Ensure Transparency and Explainability: Develop models that are easy to understand and interpret. This is crucial for building trust and ensuring responsible use.
-
Integrate with Existing Systems: Seamlessly integrate your dynamic scoring system with other business tools, such as CRM, marketing automation platforms, and risk management systems.
-
Invest in Skilled Personnel: Successful implementation requires expertise in data science, machine learning, and business process management.
Summary: Careful planning, data management, and ongoing monitoring are key to successfully leveraging the power of dynamic scoring.
Transition: Let's conclude with a summary of this guide.
Summary of Dynamic Scoring
Summary: This guide has explored the definition, applications, and implementation of dynamic scoring, highlighting its transformative potential across diverse industries. The ability to adapt to changing data and leverage real-time information provides significant advantages over traditional static scoring methods.
Closing Message: Dynamic scoring represents a powerful tool for enhancing decision-making and optimizing business processes. By understanding its intricacies and implementing it responsibly, organizations can unlock significant value and gain a competitive edge in today's rapidly evolving landscape. The future of scoring lies in its continuous adaptation and the ability to learn from evolving data patterns.
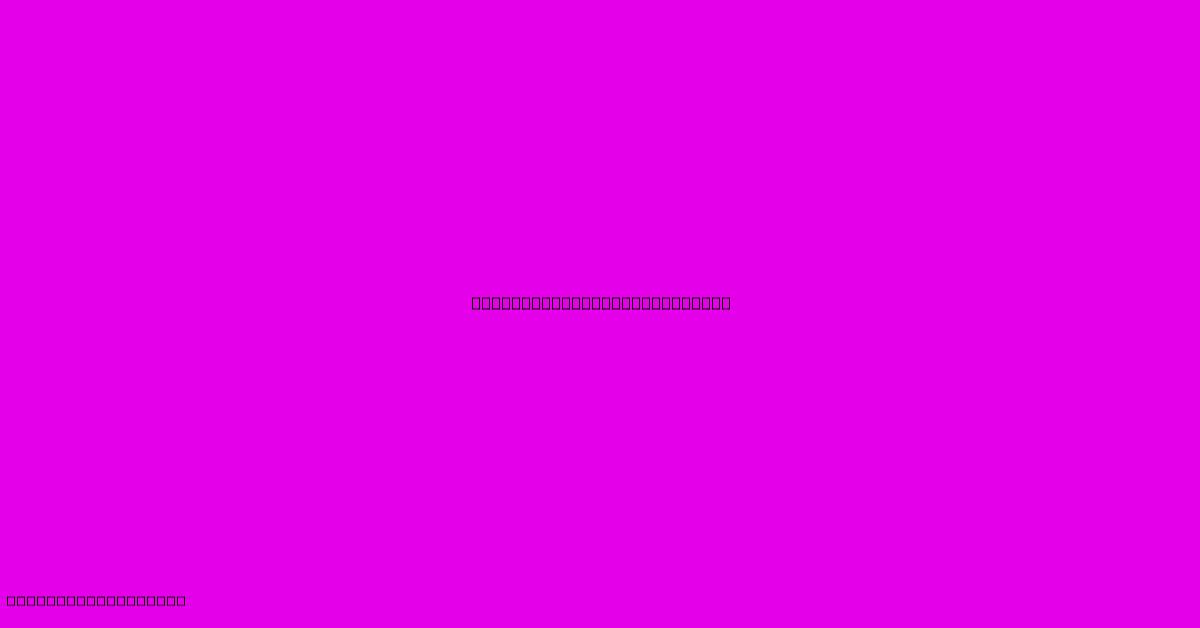
Thank you for taking the time to explore our website Dynamic Scoring Definition. We hope you find the information useful. Feel free to contact us for any questions, and don’t forget to bookmark us for future visits!
We truly appreciate your visit to explore more about Dynamic Scoring Definition. Let us know if you need further assistance. Be sure to bookmark this site and visit us again soon!
Featured Posts
-
Nonaccrual Experience Nae Method Definition
Jan 03, 2025
-
Nfa Compliance Rule 2 43b Definition
Jan 03, 2025
-
Canadian Securities Course Csc Definition Subjects Exams
Jan 03, 2025
-
Normalized Earnings Definition Purpose Benefits And Examples
Jan 03, 2025
-
How To Protect Magnetic Strip On Credit Card
Jan 03, 2025